Neural oscillations,orbrainwaves,are rhythmic or repetitive patterns of neural activity in thecentral nervous system.Neural tissuecan generateoscillatory activityin many ways, driven either by mechanisms within individualneuronsor by interactions between neurons. In individual neurons, oscillations can appear either as oscillations inmembrane potentialor as rhythmic patterns ofaction potentials,which then produce oscillatory activation ofpost-synapticneurons. At the level ofneural ensembles,synchronized activity of large numbers of neurons can give rise tomacroscopicoscillations, which can be observed in anelectroencephalogram.Oscillatory activity in groups of neurons generally arises from feedback connections between the neurons that result in the synchronization of their firing patterns. The interaction between neurons can give rise to oscillations at a different frequency than the firing frequency of individual neurons. A well-known example of macroscopic neural oscillations isalpha activity.

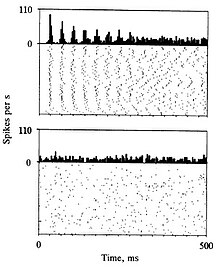
Neural oscillations in humans were observed by researchers as early as 1924 (byHans Berger). More than 50 years later, intrinsic oscillatory behavior was encountered in vertebrate neurons, but its functional role is still not fully understood.[3]The possible roles of neural oscillations includefeature binding,information transfer mechanismsand thegeneration of rhythmic motor output.Over the last decades more insight has been gained, especially with advances inbrain imaging.A major area of research inneuroscienceinvolves determining how oscillations are generated and what their roles are. Oscillatory activity in the brain is widely observed at differentlevels of organizationand is thought to play a key role in processing neural information. Numerous experimental studies support a functional role of neural oscillations; a unified interpretation, however, is still lacking.
History
editRichard Catondiscovered electrical activity in the cerebral hemispheres of rabbits and monkeys and presented his findings in 1875.[4]Adolf Beckpublished in 1890 his observations of spontaneous electrical activity of the brain of rabbits and dogs that included rhythmic oscillations altered by light, detected with electrodes directly placed on the surface of the brain.[5]BeforeHans Berger,Vladimir Vladimirovich Pravdich-Neminskypublished the first animal EEG and theevoked potentialof a dog.[6]
Overview
editNeural oscillations are observed throughout the central nervous system at all levels, and includespike trains,local field potentialsand large-scaleoscillationswhich can be measured byelectroencephalography(EEG). In general, oscillations can be characterized by theirfrequency,amplitudeandphase.These signal properties can be extracted from neural recordings usingtime-frequency analysis.In large-scale oscillations, amplitude changes are considered to result from changes in synchronization within aneural ensemble,also referred to as local synchronization. In addition to local synchronization, oscillatory activity of distant neural structures (single neurons or neural ensembles) can synchronize. Neural oscillations and synchronization have been linked to many cognitive functions such as information transfer, perception, motor control and memory.[7][8][9][10]
The opposite of neuron synchronization is neural isolation, which is when electrical activity of neurons is not temporally synchronized. This is when the likelihood of the neuron to reach its threshold potential for the signal to propagate to the next neuron decreases. This phenomenon is typically observed as the spectral intensity decreases from the summation of these neurons firing, which can be utilized to differentiate cognitive function or neural isolation. However, new non-linear methods have been used that couple temporal and spectral entropic relationships simultaneously to characterize how neurons are isolated, (the signal's inability to propagate to adjacent neurons), an indicator of impairment (e.g., hypoxia).[1]
Neural oscillations have been most widely studied in neural activity generated by large groups of neurons. Large-scale activity can be measured by techniques such as EEG. In general, EEG signals have a broad spectral content similar topink noise,but also reveal oscillatory activity in specific frequency bands. The first discovered and best-known frequency band isalpha activity(8–12Hz)[11][12][13]that can be detected from theoccipital lobeduring relaxed wakefulness and which increases when the eyes are closed.[14]Other frequency bands are:delta(1–4 Hz),theta(4–8 Hz),beta(13–30 Hz), lowgamma(30–70 Hz),[15]and high gamma (70–150 Hz) frequency bands. Faster rhythms such as gamma activity have been linked to cognitive processing. Indeed, EEG signals change dramatically during sleep. In fact, different sleep stages are commonly characterized by their spectral content.[16]Consequently, neural oscillations have been linked to cognitive states, such asawarenessandconsciousness.[17][18][15][13]
Although neural oscillations in human brain activity are mostly investigated using EEG recordings, they are also observed using more invasive recording techniques such assingle-unit recordings.Neurons can generate rhythmic patterns ofaction potentialsor spikes. Some types of neurons have the tendency to fire at particular frequencies, either asresonators[19]or asintrinsic oscillators.[2]Burstingis another form of rhythmic spiking. Spiking patterns are considered fundamental forinformation codingin the brain. Oscillatory activity can also be observed in the form ofsubthreshold membrane potential oscillations(i.e. in the absence of action potentials).[20]If numerous neurons spike insynchrony,they can give rise to oscillations inlocal field potentials.Quantitative models can estimate the strength of neural oscillations in recorded data.[21]
Neural oscillations are commonly studied within a mathematical framework and belong to the field of "neurodynamics", an area of research in thecognitive sciencesthat places a strong focus on the dynamic character of neural activity in describingbrainfunction.[22]It considers the brain adynamical systemand usesdifferential equationsto describe how neural activity evolves over time. In particular, it aims to relate dynamic patterns of brain activity to cognitive functions such as perception and memory. In very abstract form, neural oscillations can be analyzedanalytically.[23][24]When studied in a more physiologically realistic setting, oscillatory activity is generally studied usingcomputer simulationsof acomputational model.
The functions of neural oscillations are wide-ranging and vary for different types of oscillatory activity. Examples are the generation of rhythmic activity such as aheartbeatand theneural bindingof sensory features in perception, such as the shape and color of an object. Neural oscillations also play an important role in manyneurological disorders,such as excessive synchronization duringseizureactivity inepilepsy,ortremorin patients withParkinson's disease.Oscillatory activity can also be used to control external devices such as abrain–computer interface.[25]
Physiology
editOscillatory activity is observed throughout thecentral nervous systemat all levels of organization. Three different levels have been widely recognized: the micro-scale (activity of a single neuron), the meso-scale (activity of a local group of neurons) and the macro-scale (activity of different brain regions).[26]
Microscopic
editNeurons generateaction potentialsresulting from changes in the electric membrane potential. Neurons can generate multiple action potentials in sequence forming so-called spike trains. These spike trains are the basis forneural codingand information transfer in the brain. Spike trains can form all kinds of patterns, such as rhythmic spiking andbursting,and often display oscillatory activity.[27]Oscillatory activity in single neurons can also be observed insub-threshold fluctuationsin membrane potential. These rhythmic changes in membrane potential do not reach the critical threshold and therefore do not result in an action potential. They can result from postsynaptic potentials from synchronous inputs or from intrinsic properties of neurons.
Neuronal spiking can be classified by its activity pattern. The excitability of neurons can be subdivided in Class I and II. Class I neurons can generate action potentials with arbitrarily low frequency depending on the input strength, whereas Class II neurons generate action potentials in a certain frequency band, which is relatively insensitive to changes in input strength.[19]Class II neurons are also more prone to display sub-threshold oscillations in membrane potential.
Mesoscopic
editA group of neurons can also generate oscillatory activity. Through synaptic interactions, thefiring patternsof different neurons may become synchronized and the rhythmic changes in electric potential caused by their action potentials may accumulate (constructive interference). That is, synchronized firing patterns result in synchronized input into other cortical areas, which gives rise to large-amplitude oscillations of thelocal field potential.These large-scale oscillations can also be measured outside the scalp usingelectroencephalography(EEG) andmagnetoencephalography(MEG). The electric potentials generated by single neurons are far too small to be picked up outside the scalp, and EEG or MEG activity always reflects the summation of the synchronous activity of thousands or millions of neurons that have similar spatial orientation.[28]
Neurons in aneural ensemblerarely all fire at exactly the same moment, i.e. fully synchronized. Instead, the probability of firing is rhythmically modulated such that neurons are more likely to fire at the same time, which gives rise to oscillations in their mean activity. (See figure at top of page.) As such, the frequency oflarge-scaleoscillations does not need to match the firing pattern of individual neurons. Isolated cortical neurons fire regularly under certain conditions, but in the intact brain, cortical cells are bombarded by highly fluctuating synaptic inputs and typically fire seemingly at random. However, if the probability of a large group of neurons firing is rhythmically modulated at a common frequency, they will generate oscillations in the mean field. (See also figure at top of page.)[27]
Neural ensembles can generate oscillatory activityendogenouslythrough local interactions between excitatory and inhibitory neurons. In particular, inhibitoryinterneuronsplay an important role in producing neural ensemble synchrony by generating a narrow window for effective excitation and rhythmically modulating the firing rate of excitatory neurons.[29]
Macroscopic
editNeural oscillation can also arise from interactions between different brain areas coupled through the structuralconnectome.Time delaysplay an important role here. Because all brain areas are bidirectionally coupled, these connections between brain areas formfeedbackloops.Positive feedbackloops tend to cause oscillatory activity where frequency is inversely related to the delay time. An example of such a feedback loop is the connections between thethalamusandcortex– thethalamocortical radiations.This thalamocortical network is able to generate oscillatory activity known asrecurrent thalamo-cortical resonance.[30]The thalamocortical network plays an important role in the generation ofalpha activity.[31][32]In a whole-brain network model with realistic anatomical connectivity and propagation delays between brain areas, oscillations in thebeta frequency rangeemerge from the partial synchronisation of subsets of brain areas oscillating in the gamma-band (generated at the mesoscopic level).[33]
Mechanisms
editNeuronal properties
editScientists have identified some intrinsicneuronal propertiesthat play an important role in generating membrane potential oscillations. In particular,voltage-gated ion channelsare critical in the generation of action potentials. The dynamics of these ion channels have been captured in the well-establishedHodgkin–Huxley modelthat describes how action potentials are initiated and propagated by means of a set of differential equations. Usingbifurcation analysis,different oscillatory varieties of these neuronal models can be determined, allowing for the classification of types of neuronal responses. The oscillatory dynamics of neuronal spiking as identified in the Hodgkin–Huxley model closely agree with empirical findings.
In addition to periodic spiking,subthreshold membrane potential oscillations,i.e.resonancebehavior that does not result in action potentials, may also contribute to oscillatory activity by facilitating synchronous activity of neighboring neurons.[34][35]
Like pacemaker neurons incentral pattern generators,subtypes of cortical cells fire bursts of spikes (brief clusters of spikes) rhythmically at preferred frequencies.[2]Bursting neurons have the potential to serve as pacemakers for synchronous network oscillations, and bursts of spikes may underlie or enhance neuronal resonance.[27]Many of these neurons can be considered intrinsic oscillators, namely, neurons that generate their oscillations intrinsically, as their oscillation frequencies can be modified by local applications of glutamate in-vivo.[36]
Network properties
editApart from intrinsic properties of neurons,biological neural networkproperties are also an important source of oscillatory activity. Neuronscommunicatewith one another via synapses and affect the timing of spike trains in the post-synaptic neurons. Depending on the properties of the connection, such as the coupling strength, time delay and whether coupling isexcitatoryorinhibitory,the spike trains of the interacting neurons may becomesynchronized.[37]Neurons are locally connected, forming small clusters that are calledneural ensembles.Certain network structures promote oscillatory activity at specific frequencies. For example, neuronal activity generated by two populations of interconnectedinhibitoryandexcitatorycells can show spontaneous oscillations that are described by theWilson-Cowan model.
If a group of neurons engages in synchronized oscillatory activity, the neural ensemble can be mathematically represented as a single oscillator.[26]Different neural ensembles are coupled through long-range connections and form a network of weakly coupled oscillators at the next spatial scale. Weakly coupled oscillators can generate a range of dynamics including oscillatory activity.[38]Long-range connections between different brain structures, such as thethalamusand thecortex(seethalamocortical oscillation), involve time-delays due to the finiteconduction velocityof axons. Because most connections are reciprocal, they formfeed-back loopsthat support oscillatory activity. Oscillations recorded from multiple cortical areas can become synchronized to formlarge-scale brain networks,whose dynamics and functional connectivity can be studied by means ofspectral analysisandGranger causalitymeasures.[39]Coherent activity of large-scale brain activity may form dynamic links between brain areas required for the integration of distributed information.[18]
Microglia– the major immune cells of the brain – have been shown to play an important role in shaping network connectivity, and thus, influencing neuronal network oscillations bothex vivoandin vivo.[40]
Neuromodulation
editIn addition to fast directsynaptic interactionsbetween neurons forming a network, oscillatory activity is regulated byneuromodulatorson a much slower time scale. That is, the concentration levels of certain neurotransmitters are known to regulate the amount of oscillatory activity. For instance,GABAconcentration has been shown to be positively correlated with frequency of oscillations in induced stimuli.[41]A number ofnucleiin thebrainstemhave diffuse projections throughout the brain influencing concentration levels of neurotransmitters such asnorepinephrine,acetylcholineandserotonin.These neurotransmitter systems affect the physiological state, e.g.,wakefulnessorarousal,and have a pronounced effect on amplitude of different brain waves, such as alpha activity.[42]
Mathematical description
editOscillations can often be described and analyzed using mathematics. Mathematicians have identified severaldynamicalmechanisms that generate rhythmicity. Among the most important areharmonic(linear) oscillators,limit cycleoscillators, and delayed-feedbackoscillators.[43]Harmonic oscillations appear very frequently in nature—examples are sound waves, the motion of apendulum,and vibrations of every sort. They generally arise when a physical system is perturbed by a small degree from aminimum-energy state,and are well understood mathematically.
Noise-driven harmonic oscillators realistically simulate alpha rhythm in the waking EEG as well as slow waves and spindles in the sleep EEG. SuccessfulEEG analysisalgorithms were based on such models. Several other EEG components are better described by limit-cycle or delayed-feedback oscillations.
Limit-cycle oscillations arise from physical systems that show large deviations fromequilibrium,whereas delayed-feedback oscillations arise when components of a system affect each other after significant time delays. Limit-cycle oscillations can be complex but there are powerful mathematical tools for analyzing them; the mathematics of delayed-feedback oscillations is primitive in comparison. Linear oscillators and limit-cycle oscillators qualitatively differ in terms of how they respond to fluctuations in input. In a linear oscillator, the frequency is more or less constant but the amplitude can vary greatly. In a limit-cycle oscillator, the amplitude tends to be more or less constant but the frequency can vary greatly. Aheartbeatis an example of a limit-cycle oscillation in that the frequency of beats varies widely, while each individual beat continues to pump about the same amount of blood.
Computational modelsadopt a variety of abstractions in order to describe complex oscillatory dynamics observed in brain activity. Many models are used in the field, each defined at a different level of abstraction and trying to model different aspects of neural systems. They range from models of the short-term behaviour of individual neurons, through models of how the dynamics ofneural circuitryarise from interactions between individual neurons, to models of how behaviour can arise from abstract neural modules that represent complete subsystems.
Single neuron model
editA model of a biological neuron is a mathematical description of the properties of nerve cells, or neurons, that is designed to accurately describe and predict its biological processes. One of the most successful neuron models is the Hodgkin–Huxley model, for whichHodgkinandHuxleywon the 1963 Nobel Prize in physiology or medicine. The model is based on data from thesquid giant axonand consists of nonlinear differential equations that approximate the electrical characteristics of a neuron, including the generation and propagation ofaction potentials.The model is so successful at describing these characteristics that variations of its "conductance-based" formulation continue to be utilized in neuron models over a half a century later.[44]
The Hodgkin–Huxley model is too complicated to understand using classical mathematical techniques, so researchers often turn to simplifications such as theFitzHugh–Nagumo modeland theHindmarsh–Rose model,or highly idealized neuron models such as the leaky integrate-and-fire neuron, originally developed by Lapique in 1907.[45][46]Such models only capture salient membrane dynamics such as spiking orburstingat the cost of biophysical detail, but are more computationally efficient, enabling simulations of largerbiological neural networks.
Spiking model
editA neural network model describes a population of physically interconnected neurons or a group of disparate neurons whose inputs or signalling targets define a recognizable circuit. These models aim to describe how the dynamics of neural circuitry arise from interactions between individual neurons. Local interactions between neurons can result in the synchronization of spiking activity and form the basis of oscillatory activity. In particular, models of interactingpyramidal cellsand inhibitoryinterneuronshave been shown to generate brain rhythms such asgamma activity.[47]Similarly, it was shown that simulations of neural networks with a phenomenological model for neuronal response failures can predict spontaneous broadband neural oscillations.[48]
Neural mass model
editNeural field models are another important tool in studying neural oscillations and are a mathematical framework describing evolution of variables such as mean firing rate in space and time. In modeling the activity of large numbers of neurons, the central idea is to take the density of neurons to thecontinuum limit,resulting in spatially continuousneural networks.Instead of modelling individual neurons, this approach approximates a group of neurons by its average properties and interactions. It is based on themean field approach,an area ofstatistical physicsthat deals with large-scale systems. Models based on these principles have been used to provide mathematical descriptions of neural oscillations and EEG rhythms. They have for instance been used to investigate visual hallucinations.[50]
Kuramoto model
editTheKuramoto modelof coupled phase oscillators[51]is one of the most abstract and fundamental models used to investigate neural oscillations and synchronization. It captures the activity of a local system (e.g., a single neuron or neural ensemble) by its circularphasealone and hence ignores the amplitude of oscillations (amplitude is constant).[52]Interactions amongst these oscillators are introduced by a simple algebraic form (such as asinefunction) and collectively generate a dynamical pattern at the global scale.
The Kuramoto model is widely used to study oscillatory brain activity, and several extensions have been proposed that increase its neurobiological plausibility, for instance by incorporating topological properties of local cortical connectivity.[53]In particular, it describes how the activity of a group of interacting neurons can become synchronized and generate large-scale oscillations.
Simulations using the Kuramoto model with realistic long-range cortical connectivity and time-delayed interactions reveal the emergence of slow patterned fluctuations that reproduce resting-stateBOLDfunctional maps, which can be measured usingfMRI.[54]
Activity patterns
editBoth single neurons and groups of neurons can generate oscillatory activity spontaneously. In addition, they may show oscillatory responses to perceptual input or motor output. Some types of neurons will fire rhythmically in the absence of any synaptic input. Likewise, brain-wide activity reveals oscillatory activity while subjects do not engage in any activity, so-calledresting-state activity.These ongoing rhythms can change in different ways in response to perceptual input or motor output. Oscillatory activity may respond by increases or decreases in frequency and amplitude or show a temporary interruption, which is referred to as phase resetting. In addition, external activity may not interact with ongoing activity at all, resulting in an additive response.
Ongoing activity
editSpontaneous activity isbrainactivity in the absence of an explicit task, such as sensory input or motor output, and hence also referred to as resting-state activity. It is opposed to induced activity, i.e. brain activity that is induced by sensory stimuli or motor responses.
The termongoing brain activityis used inelectroencephalographyandmagnetoencephalographyfor those signal components that are not associated with the processing of astimulusor the occurrence of specific other events, such as moving a body part, i.e. events that do not formevoked potentials/evoked fields,or induced activity.
Spontaneous activity is usually considered to benoiseif one is interested in stimulus processing; however, spontaneous activity is considered to play a crucial role during brain development, such as in network formation and synaptogenesis. Spontaneous activity may be informative regarding the current mental state of the person (e.g. wakefulness, alertness) and is often used in sleep research. Certain types of oscillatory activity, such asalpha waves,are part of spontaneous activity. Statistical analysis of power fluctuations of alpha activity reveals a bimodal distribution, i.e. a high- and low-amplitude mode, and hence shows that resting-state activity does not just reflect anoiseprocess.[55]
In case of fMRI, spontaneous fluctuations in theblood-oxygen-level dependent(BOLD) signal reveal correlation patterns that are linked to resting state networks, such as thedefault network.[56]The temporal evolution of resting state networks is correlated with fluctuations of oscillatory EEG activity in different frequency bands.[57]
Ongoing brain activity may also have an important role in perception, as it may interact with activity related to incoming stimuli. Indeed,EEGstudies suggest that visual perception is dependent on both the phase and amplitude of cortical oscillations. For instance, the amplitude and phase of alpha activity at the moment of visual stimulation predicts whether a weak stimulus will be perceived by the subject.[58][59][60]
Frequency response
editIn response to input, a neuron orneuronal ensemblemay change the frequency at which it oscillates, thus changing therateat which it spikes. Often, a neuron's firing rate depends on the summed activity it receives. Frequency changes are also commonly observed incentral pattern generatorsand directly relate to the speed ofmotor activities,such as step frequency in walking. However, changes inrelativeoscillation frequency between differentbrain areasis not so common because the frequency of oscillatory activity is often related to the time delays between brain areas.
Amplitude response
editNext to evoked activity, neural activity related to stimulus processing may result in induced activity. Induced activity refers to modulation in ongoing brain activity induced by processing of stimuli or movement preparation. Hence, they reflect an indirect response in contrast to evoked responses. A well-studied type of induced activity is amplitude change in oscillatory activity. For instance,gamma activityoften increases during increased mental activity such as during object representation.[61]Because induced responses may have different phases across measurements and therefore would cancel out during averaging, they can only be obtained usingtime-frequency analysis.Induced activity generally reflects the activity of numerous neurons: amplitude changes in oscillatory activity are thought to arise from the synchronization of neural activity, for instance by synchronization of spike timing or membrane potential fluctuations of individual neurons. Increases in oscillatory activity are therefore often referred to as event-related synchronization, while decreases are referred to as event-related desynchronization.[62]
Phase resetting
editPhase resetting occurs when input to a neuron or neuronal ensemble resets the phase of ongoing oscillations.[63]It is very common in single neurons where spike timing is adjusted to neuronal input (a neuron may spike at a fixed delay in response to periodic input, which is referred to as phase locking[19]) and may also occur in neuronal ensembles when the phases of their neurons are adjusted simultaneously. Phase resetting is fundamental for the synchronization of different neurons or different brain regions[18][38]because the timing of spikes can become phase locked to the activity of other neurons.
Phase resetting also permits the study of evoked activity, a term used inelectroencephalographyandmagnetoencephalographyfor responses in brain activity that are directly related tostimulus-related activity.Evoked potentialsandevent-related potentialsare obtained from an electroencephalogram by stimulus-locked averaging, i.e. averaging different trials at fixed latencies around the presentation of a stimulus. As a consequence, those signal components that are the same in each single measurement are conserved and all others, i.e. ongoing or spontaneous activity, are averaged out. That is, event-related potentials only reflect oscillations in brain activity that arephase-locked to the stimulus or event. Evoked activity is often considered to be independent from ongoing brain activity, although this is an ongoing debate.[64][65]
Asymmetric amplitude modulation
editIt has recently been proposed that even if phases are not aligned across trials, induced activity may still causeevent-related potentialsbecause ongoing brain oscillations may not be symmetric and thus amplitude modulations may result in a baseline shift that does not average out.[66][67]This model implies that slow event-related responses, such as asymmetric alpha activity, could result from asymmetric brain oscillation amplitude modulations, such as an asymmetry of the intracellular currents that propagate forward and backward down the dendrites.[68]Under this assumption, asymmetries in the dendritic current would cause asymmetries in oscillatory activity measured by EEG and MEG, since dendritic currents in pyramidal cells are generally thought to generate EEG and MEG signals that can be measured at the scalp.[69]
Cross-frequency coupling
editCross-frequency coupling (CFC) describes the coupling (statistical correlation) between a slow wave and a fast wave. There are many kinds, generally written as A-B coupling, meaning the A of a slow wave is coupled with the B of a fast wave. For example, phase–amplitude coupling is where the phase of a slow wave is coupled with the amplitude of a fast wave.[70]
Thetheta-gamma codeis a coupling between theta wave and gamma wave in the hippocampal network. During a theta wave, 4 to 8 non-overlapping neuron ensembles are activated in sequence. This has been hypothesized to form a neural code representing multiple items in a temporal frame[71][72]
Function
editNeural synchronization can be modulated by task constraints, such asattention,and is thought to play a role infeature binding,[73]neuronal communication,[7]andmotor coordination.[9]Neuronal oscillations became a hot topic inneurosciencein the 1990s when the studies of the visual system of the brain by Gray, Singer and others appeared to support theneural bindinghypothesis.[74]According to this idea, synchronous oscillations in neuronal ensembles bind neurons representing different features of an object. For example, when a person looks at a tree, visual cortex neurons representing the tree trunk and those representing the branches of the same tree would oscillate in synchrony to form a single representation of the tree. This phenomenon is best seen inlocal field potentialswhich reflect the synchronous activity of local groups of neurons, but has also been shown inEEGandMEGrecordings providing increasing evidence for a close relation between synchronous oscillatory activity and a variety of cognitive functions such as perceptual grouping[73]and attentional top-down control.[15][13][12]
Pacemaker
editCells in thesinoatrial node,located in theright atriumof the heart, spontaneouslydepolarizeapproximately 100 times per minute. Although all of the heart's cells have the ability to generate action potentials that trigger cardiac contraction, the sinoatrial node normally initiates it, simply because it generates impulses slightly faster than the other areas. Hence, these cells generate the normalsinus rhythmand are called pacemaker cells as they directly control theheart rate.In the absence of extrinsic neural and hormonal control, cells in the SA node will rhythmically discharge. The sinoatrial node is richly innervated by theautonomic nervous system,which up or down regulates the spontaneous firing frequency of the pacemaker cells.
Central pattern generator
editSynchronized firing of neurons also forms the basis of periodic motor commands for rhythmic movements. These rhythmic outputs are produced by a group of interacting neurons that form a network, called acentral pattern generator.Central pattern generators are neuronal circuits that—when activated—can produce rhythmic motor patterns in the absence of sensory or descending inputs that carry specific timing information. Examples arewalking,breathing,andswimming,[75]Most evidence for central pattern generators comes from lower animals, such as thelamprey,but there is also evidence for spinal central pattern generators in humans.[76][77]
Information processing
editNeuronal spiking is generally considered the basis for information transfer in the brain. For such a transfer, information needs to be coded in a spiking pattern. Different types of coding schemes have been proposed, such asrate codingandtemporal coding.Neural oscillations could create periodic time windows in which input spikes have larger effect on neurons, thereby providing a mechanism for decoding temporal codes.[78]
Temporal decoding
editSingle-cell intrinsic oscillators serve as valuable tools for decoding temporally-encoded sensory information. This information is encoded through inter-spike intervals, and intrinsic oscillators can act as 'temporal rulers' for precisely measuring these intervals. One notable mechanism for achieving this is the neuronalphase-locked loop(NPLL). In this mechanism, cortical oscillators undergo modulation influenced by the firing rates of thalamocortical 'phase detectors,' which, in turn, gauge the disparity between the cortical and sensory periodicity.[79]
Perception
editSynchronization of neuronal firing may serve as a means to group spatially segregated neurons that respond to the same stimulus in order to bind these responses for further joint processing, i.e. to exploit temporal synchrony to encode relations. Purely theoretical formulations of the binding-by-synchrony hypothesis were proposed first,[80]but subsequently extensive experimental evidence has been reported supporting the potential role of synchrony as a relational code.[81]
The functional role of synchronized oscillatory activity in the brain was mainly established in experiments performed on awake kittens with multiple electrodes implanted in the visual cortex. These experiments showed that groups of spatially segregated neurons engage in synchronous oscillatory activity when activated by visual stimuli. The frequency of these oscillations was in the range of 40 Hz and differed from the periodic activation induced by the grating, suggesting that the oscillations and their synchronization were due to internal neuronal interactions.[81]Similar findings were shown in parallel by the group of Eckhorn, providing further evidence for the functional role of neural synchronization in feature binding.[82]Since then, numerous studies have replicated these findings and extended them to different modalities such as EEG, providing extensive evidence of the functional role ofgammaoscillations in visual perception.
Gilles Laurent and colleagues showed that oscillatory synchronization has an important functional role in odor perception. Perceiving different odors leads to different subsets of neurons firing on different sets of oscillatory cycles.[83]These oscillations can be disrupted byGABAblockerpicrotoxin,[84]and the disruption of the oscillatory synchronization leads to impairment of behavioral discrimination of chemically similar odorants in bees,[85]and to more similar responses across odors in downstream β-lobe neurons.[86]Recent follow-up of this work has shown that oscillations create periodic integration windows forKenyon cellsin the insectmushroom body,such that incoming spikes from theantennal lobeare more effective in activating Kenyon cells only at specific phases of the oscillatory cycle.[78]
Neural oscillations are also thought be involved in thesense of time[87]and in somatosensory perception.[88]However, recent findings argue against a clock-like function of cortical gamma oscillations.[89]
Motor coordination
editOscillations have been commonly reported in the motor system. Pfurtscheller and colleagues found a reduction inalpha(8–12 Hz) andbeta(13–30 Hz) oscillations inEEGactivity when subjects made a movement.[62][90]Using intra-cortical recordings, similar changes in oscillatory activity were found in the motor cortex when the monkeys performed motor acts that required significant attention.[91][92]In addition, oscillations at spinal level become synchronised to beta oscillations in the motor cortex during constant muscle activation, as determined bycortico-muscular coherence.[93][94][95]Likewise, muscle activity of different muscles revealsinter-muscular coherenceat multiple distinct frequencies reflecting the underlyingneural circuitryinvolved inmotor coordination.[96][97]
Recently it was found that cortical oscillations propagate astravelling wavesacross the surface of the motor cortex along dominant spatial axes characteristic of the local circuitry of the motor cortex.[98]It has been proposed that motor commands in the form of travelling waves can be spatially filtered by the descending fibres to selectively control muscle force.[99]Simulations have shown that ongoing wave activity in cortex can elicit steady muscle force with physiological levels of EEG-EMG coherence.[100]
Oscillatory rhythms at 10 Hz have been recorded in a brain area called theinferior olive,which is associated with the cerebellum.[20]These oscillations are also observed in motor output of physiologicaltremor[101]and when performing slow finger movements.[102]These findings may indicate that the human brain controls continuous movements intermittently. In support, it was shown that these movement discontinuities are directly correlated to oscillatory activity in a cerebello-thalamo-cortical loop, which may represent a neural mechanism for the intermittent motor control.[103]
Memory
editNeural oscillations, in particularthetaactivity, are extensively linked to memory function. Theta rhythms are very strong in rodent hippocampi and entorhinal cortex during learning and memory retrieval, and they are believed to be vital to the induction oflong-term potentiation,a potential cellular mechanism for learning and memory.Couplingbetween theta andgammaactivity is thought to be vital for memory functions, includingepisodic memory.[104][105]Tight coordination of single-neuron spikes with local theta oscillations is linked to successful memory formation in humans, as more stereotyped spiking predicts better memory.[106]
Sleep and consciousness
editSleep is a naturally recurring state characterized by reduced or absentconsciousnessand proceeds in cycles ofrapid eye movement(REM) andnon-rapid eye movement(NREM) sleep. Sleep stages are characterized by spectral content ofEEG:for instance, stage N1 refers to the transition of the brain from alpha waves (common in the awake state) to theta waves, whereas stage N3 (deep or slow-wave sleep) is characterized by the presence of delta waves.[107]The normal order of sleep stages is N1 → N2 → N3 → N2 → REM.[citation needed]
Development
editNeural oscillations may play a role in neural development. For example,retinal wavesare thought to have properties that define early connectivity of circuits and synapses between cells in the retina.[108]
Pathology
editSpecific types of neural oscillations may also appear in pathological situations, such asParkinson's diseaseorepilepsy.These pathological oscillations often consist of an aberrant version of a normal oscillation. For example, one of the best known types is thespike and waveoscillation, which is typical of generalized or absence epileptic seizures, and which resembles normal sleep spindle oscillations.
Tremor
editA tremor is an involuntary, somewhat rhythmic, muscle contraction and relaxation involving to-and-fro movements of one or more body parts. It is the most common of all involuntary movements and can affect the hands, arms, eyes, face, head, vocal cords, trunk, and legs. Most tremors occur in the hands. In some people, tremor is a symptom of another neurological disorder. Many different forms of tremor have been identified, such asessential tremororParkinsoniantremor. It is argued that tremors are likely to be multifactorial in origin, with contributions from neural oscillations in the central nervous systems, but also from peripheral mechanisms such as reflex loop resonances.[109]
Epilepsy
editEpilepsy is a common chronic neurological disorder characterized byseizures.These seizures are transient signs and/or symptoms of abnormal, excessive orhypersynchronous neuronal activityin the brain.[110]
Thalamocortical dysrhythmia
editIn thalamocortical dysrhythmia (TCD), normalthalamocortical resonanceis disrupted. The thalamic loss of input allows the frequency of the thalamo-cortical column to slow into the theta or delta band as identified by MEG and EEG by machine learning.[111]TCD can be treated withneurosurgicalmethods likethalamotomy.
Applications
editClinical endpoints
editNeural oscillations are sensitive to several drugs influencing brain activity; accordingly,biomarkersbased on neural oscillations are emerging assecondary endpointsin clinical trials and in quantifying effects in pre-clinical studies. These biomarkers are often named "EEG biomarkers" or "Neurophysiological Biomarkers" and are quantified usingquantitative electroencephalography (qEEG).EEG biomarkers can be extracted from the EEG using the open-sourceNeurophysiological Biomarker Toolbox.
Brain–computer interface
editNeural oscillation has been applied as a control signal in variousbrain–computer interfaces(BCIs).[112]For example, a non-invasive BCI can be created by placing electrodes on the scalp and then measuring the weak electric signals. Although individual neuron activities cannot be recorded through non-invasive BCI because the skull damps and blurs the electromagnetic signals, oscillatory activity can still be reliably detected. The BCI was introduced by Vidal in 1973[113]as challenge of using EEG signals to control objects outside human body.
After the BCI challenge, in 1988, alpha rhythm was used in a brain rhythm based BCI for control of a physical object, a robot.[114][115]Alpha rhythm based BCI was the first BCI for control of a robot.[116][117]In particular, some forms of BCI allow users to control a device by measuring the amplitude of oscillatory activity in specific frequency bands, includingmuandbetarhythms.
Examples
editA non-inclusive list of types of oscillatory activity found in the central nervous system:
See also
editReferences
edit- ^abNapoli, Nicholas J.; Demas, Matthew; Stephens, Chad L.; Kennedy, Kellie D.; Harrivel, Angela R.; Barnes, Laura E.; Pope, Alan T. (2020-03-03)."Activation Complexity: A Cognitive Impairment Tool for Characterizing Neuro-isolation".Scientific Reports.10(1): 3909.Bibcode:2020NatSR..10.3909N.doi:10.1038/s41598-020-60354-2.ISSN2045-2322.PMC7054256.PMID32127579.This article incorporates text from this source, which is available under theCC BY 4.0license.
- ^abcAhissar, E. & Vaadia, E. Oscillatory activity of single units in a somatosensory cortex of an awake monkey and their possible role in texture analysis. Proc Natl Acad Sci USA 87, 8935-8939 (1990).
- ^Llinás RR (2014)."Intrinsic electrical properties of mammalian neurons and CNS function: a historical perspective".Frontiers in Cellular Neuroscience.8:320.doi:10.3389/fncel.2014.00320.PMC4219458.PMID25408634.
- ^"Caton, Richard - The electric currents of the brain".echo.mpiwg-berlin.mpg.de.Retrieved2018-12-21.
- ^Coenen A, Fine E, Zayachkivska O (2014). "Adolf Beck: a forgotten pioneer in electroencephalography".Journal of the History of the Neurosciences.23(3):276–86.doi:10.1080/0964704x.2013.867600.PMID24735457.S2CID205664545.
- ^Pravdich-Neminsky VV (1913). "Ein Versuch der Registrierung der elektrischen Gehirnerscheinungen".Zentralblatt für Physiologie.27:951–60.
- ^abFries P (October 2005). "A mechanism for cognitive dynamics: neuronal communication through neuronal coherence".Trends in Cognitive Sciences.9(10):474–80.doi:10.1016/j.tics.2005.08.011.PMID16150631.S2CID6275292.
- ^Fell J, Axmacher N (February 2011). "The role of phase synchronization in memory processes".Nature Reviews. Neuroscience.12(2):105–18.doi:10.1038/nrn2979.PMID21248789.S2CID7422401.
- ^abSchnitzler A, Gross J (April 2005). "Normal and pathological oscillatory communication in the brain".Nature Reviews. Neuroscience.6(4):285–96.doi:10.1038/nrn1650.PMID15803160.S2CID2749709.
- ^Baldauf D, Desimone R (April 2014)."Neural mechanisms of object-based attention".Science.344(6182):424–7.Bibcode:2014Sci...344..424B.doi:10.1126/science.1247003.PMID24763592.S2CID34728448.
- ^Foster JJ, Sutterer DW, Serences JT, Vogel EK, Awh E (July 2017)."Alpha-Band Oscillations Enable Spatially and Temporally Resolved Tracking of Covert Spatial Attention".Psychological Science.28(7):929–941.doi:10.1177/0956797617699167.PMC5675530.PMID28537480.
- ^abde Vries IE, Marinato G, Baldauf D (August 2021)."Decoding object-based auditory attention from source-reconstructed MEG alpha oscillations".The Journal of Neuroscience.41(41):8603–8617.doi:10.1523/JNEUROSCI.0583-21.2021.PMC8513695.PMID34429378.
- ^abcBagherzadeh Y, Baldauf D, Pantazis D, Desimone R (February 2020)."Alpha Synchrony and the Neurofeedback Control of Spatial Attention".Neuron.105(3): 577–587.e5.doi:10.1016/j.neuron.2019.11.001.hdl:11572/252726.PMID31812515.S2CID208614924.
- ^Berger H, Gray CM (1929). "Uber das Elektroenkephalogramm des Menschen".Arch Psychiat Nervenkr.87:527–570.doi:10.1007/BF01797193.hdl:11858/00-001M-0000-002A-5DE0-7.S2CID10835361.
- ^abcBaldauf D, Desimone R (April 2014)."Neural mechanisms of object-based attention".Science.344(6182):424–7.Bibcode:2014Sci...344..424B.doi:10.1126/science.1247003.PMID24763592.S2CID34728448.
- ^Dement W, Kleitman N (November 1957). "Cyclic variations in EEG during sleep and their relation to eye movements, body motility, and dreaming".Electroencephalography and Clinical Neurophysiology.9(4):673–90.doi:10.1016/0013-4694(57)90088-3.PMID13480240.
- ^Engel AK, Singer W (January 2001). "Temporal binding and the neural correlates of sensory awareness".Trends in Cognitive Sciences.5(1):16–25.doi:10.1016/S1364-6613(00)01568-0.PMID11164732.S2CID11922975.
- ^abcVarela F, Lachaux JP, Rodriguez E, Martinerie J (April 2001). "The brainweb: phase synchronization and large-scale integration".Nature Reviews. Neuroscience.2(4):229–39.doi:10.1038/35067550.PMID11283746.S2CID18651043.
- ^abcIzhikevich EM (2007).Dynamical systems in neuroscience.Cambridge, Massachusetts: The MIT Press.
- ^abLlinás R, Yarom Y (July 1986)."Oscillatory properties of guinea-pig inferior olivary neurones and their pharmacological modulation: an in vitro study".The Journal of Physiology.376:163–82.doi:10.1113/jphysiol.1986.sp016147.PMC1182792.PMID3795074.
- ^Mureşan RC, Jurjuţ OF, Moca VV, Singer W, Nikolić D (March 2008). "The oscillation score: an efficient method for estimating oscillation strength in neuronal activity".Journal of Neurophysiology.99(3):1333–53.doi:10.1152/jn.00772.2007.PMID18160427.
- ^Burrow T (1943). "The neurodynamics of behavior. A phylobiological foreword".Philosophy of Science.10(4):271–288.doi:10.1086/286819.S2CID121438105.
- ^Amari, Shun-ichi (1977-06-01)."Dynamics of pattern formation in lateral-inhibition type neural fields".Biological Cybernetics.27(2):77–87.doi:10.1007/BF00337259.ISSN1432-0770.PMID911931.S2CID2811608.
- ^Schöner, G.; Kelso, J. A. S. (1988-03-25)."Dynamic Pattern Generation in Behavioral and Neural Systems".Science.239(4847):1513–1520.Bibcode:1988Sci...239.1513S.doi:10.1126/science.3281253.ISSN0036-8075.PMID3281253.
- ^Vansteensel MJ, Pels EG, Bleichner MG, Branco MP, Denison T, Freudenburg ZV, et al. (November 2016)."Fully Implanted Brain-Computer Interface in a Locked-In Patient with ALS".The New England Journal of Medicine.375(21):2060–2066.doi:10.1056/NEJMoa1608085.hdl:1874/344360.PMC5326682.PMID27959736.
- ^abHaken H (1996).Principles of brain functioning.Springer.ISBN978-3-540-58967-9.
- ^abcWang XJ (July 2010)."Neurophysiological and computational principles of cortical rhythms in cognition".Physiological Reviews.90(3):1195–268.doi:10.1152/physrev.00035.2008.PMC2923921.PMID20664082.
- ^Nunez PL, Srinivasan R (1981).Electric fields of the brain: The neurophysics of EEG.Oxford University Press.ISBN9780195027969.
- ^Cardin JA, Carlén M, Meletis K, Knoblich U, Zhang F, Deisseroth K, et al. (June 2009)."Driving fast-spiking cells induces gamma rhythm and controls sensory responses".Nature.459(7247):663–7.Bibcode:2009Natur.459..663C.doi:10.1038/nature08002.PMC3655711.PMID19396156.
- ^Llinás R, Ribary U, Contreras D, Pedroarena C (November 1998)."The neuronal basis for consciousness".Philosophical Transactions of the Royal Society of London. Series B, Biological Sciences.353(1377):1841–9.doi:10.1098/rstb.1998.0336.PMC1692417.PMID9854256.
- ^Bollimunta A, Mo J, Schroeder CE, Ding M (March 2011)."Neuronal mechanisms and attentional modulation of corticothalamic α oscillations".The Journal of Neuroscience.31(13):4935–43.doi:10.1523/JNEUROSCI.5580-10.2011.PMC3505610.PMID21451032.
- ^Suffczynski P, Kalitzin S, Pfurtscheller G, Lopes da Silva FH (December 2001). "Computational model of thalamo-cortical networks: dynamical control of alpha rhythms in relation to focal attention".International Journal of Psychophysiology.43(1):25–40.doi:10.1016/S0167-8760(01)00177-5.PMID11742683.
- ^Cabral J, Luckhoo H, Woolrich M, Joensson M, Mohseni H, Baker A, et al. (April 2014)."Exploring mechanisms of spontaneous functional connectivity in MEG: how delayed network interactions lead to structured amplitude envelopes of band-pass filtered oscillations".NeuroImage.90:423–35.doi:10.1016/j.neuroimage.2013.11.047.hdl:10230/23081.PMID24321555.
- ^Llinás RR (December 1988). "The intrinsic electrophysiological properties of mammalian neurons: insights into central nervous system function".Science.242(4886):1654–64.Bibcode:1988Sci...242.1654L.doi:10.1126/science.3059497.PMID3059497.
- ^Llinás RR, Grace AA, Yarom Y (February 1991)."In vitro neurons in mammalian cortical layer 4 exhibit intrinsic oscillatory activity in the 10- to 50-Hz frequency range".Proceedings of the National Academy of Sciences of the United States of America.88(3):897–901.Bibcode:1991PNAS...88..897L.doi:10.1073/pnas.88.3.897.PMC50921.PMID1992481.
- ^Ahissar, E., Haidarliu, S., and Zacksenhouse, M. (1997). Decoding temporally encoded sensory input by cortical oscillations and thalamic phase comparators. Proc Natl Acad Sci USA 94, 11633-11638.
- ^Zeitler M, Daffertshofer A, Gielen CC (June 2009)."Asymmetry in pulse-coupled oscillators with delay"(PDF).Physical Review E.79(6 Pt 2): 065203.Bibcode:2009PhRvE..79f5203Z.doi:10.1103/PhysRevE.79.065203.hdl:1871/29169.PMID19658549.
- ^abPikovsky A, Rosenblum M, Kurths J (2001).Synchronization: a universal concept in nonlinear sciences.Cambridge University Press.ISBN978-0-521-53352-2.
- ^Andrea Brovelli, Steven L. Bressler and their colleagues,2004
- ^Peter, Berki; Csaba, Cserep; Zsuzsanna, Környei (2024)."Microglia contribute to neuronal synchrony despite endogenous ATP-related phenotypic transformation in acute mouse brain slices".Nature Communications.15(1): 5402.Bibcode:2024NatCo..15.5402B.doi:10.1038/s41467-024-49773-1.PMC11208608.PMID38926390.
- ^Muthukumaraswamy SD, Edden RA, Jones DK, Swettenham JB, Singh KD (May 2009)."Resting GABA concentration predicts peak gamma frequency and fMRI amplitude in response to visual stimulation in humans".Proceedings of the National Academy of Sciences of the United States of America.106(20):8356–61.Bibcode:2009PNAS..106.8356M.doi:10.1073/pnas.0900728106.PMC2688873.PMID19416820.
- ^Moruzzi G, Magoun HW (November 1949)."Brain stem reticular formation and activation of the EEG".Electroencephalography and Clinical Neurophysiology.1(4):455–73.doi:10.1016/0013-4694(49)90219-9.PMID18421835.
- ^Buzsáki G, Draguhn A (June 2004). "Neuronal oscillations in cortical networks".Science.304(5679):1926–9.Bibcode:2004Sci...304.1926B.doi:10.1126/science.1099745.PMID15218136.S2CID8002293.
- ^Catterall, W. A., Raman, I. M., Robinson, H. P. C., Sejnowski, T. J., Paulsen, O. (2 October 2012)."The Hodgkin-Huxley Heritage: From Channels to Circuits".The Journal of Neuroscience.32(41):14064–14073.doi:10.1523/JNEUROSCI.3403-12.2012.PMC3500626.PMID23055474.
- ^Lapicque, LM (1907). "Recherches quantitatives sur l'excitation electrique des nerfs".J Physiol Paris.9:620–635.
- ^Abbott, Larry (1999). "Lapicque's introduction of the integrate-and-fire model neuron (1907)".Brain Research Bulletin.50(5):303–304.doi:10.1016/S0361-9230(99)00161-6.PMID10643408.S2CID46170924.
- ^Whittington MA, Traub RD, Kopell N, Ermentrout B, Buhl EH (December 2000). "Inhibition-based rhythms: experimental and mathematical observations on network dynamics".International Journal of Psychophysiology.38(3):315–36.CiteSeerX10.1.1.16.6410.doi:10.1016/S0167-8760(00)00173-2.PMID11102670.
- ^Goldental A, Vardi R, Sardi S, Sabo P, Kanter I (2015)."Broadband macroscopic cortical oscillations emerge from intrinsic neuronal response failures".Frontiers in Neural Circuits.9:65.arXiv:1511.00235.doi:10.3389/fncir.2015.00065.PMC4626558.PMID26578893.
- ^Wendling F, Bellanger JJ, Bartolomei F, Chauvel P (October 2000). "Relevance of nonlinear lumped-parameter models in the analysis of depth-EEG epileptic signals".Biological Cybernetics.83(4):367–78.doi:10.1007/s004220000160.PMID11039701.S2CID8751526.
- ^Bressloff PC, Cowan JD (2003) Spontaneous pattern formation in primary visual cortex. In: J Hogan, AR Krauskopf, M di Bernado, RE Wilson (Eds.), Nonlinear dynamics and chaos: where do we go from here?
- ^Kuramoto Y (1984).Chemical Oscillations, Waves, and Turbulence.Dover Publications.
- ^Ermentrout B (1994). "An introduction to neural oscillators". In F Ventriglia (ed.).Neural Modeling and Neural Networks.pp.79–110.
- ^Breakspear M, Heitmann S, Daffertshofer A (2010)."Generative models of cortical oscillations: neurobiological implications of the kuramoto model".Frontiers in Human Neuroscience.4:190.doi:10.3389/fnhum.2010.00190.PMC2995481.PMID21151358.
- ^Cabral J, Hugues E, Sporns O, Deco G (July 2011)."Role of local network oscillations in resting-state functional connectivity".NeuroImage.57(1):130–139.doi:10.1016/j.neuroimage.2011.04.010.PMID21511044.S2CID13959959.
- ^Freyer F, Aquino K, Robinson PA, Ritter P, Breakspear M (July 2009)."Bistability and non-Gaussian fluctuations in spontaneous cortical activity".The Journal of Neuroscience.29(26):8512–24.doi:10.1523/JNEUROSCI.0754-09.2009.PMC6665653.PMID19571142.
- ^Fox MD, Raichle ME (September 2007). "Spontaneous fluctuations in brain activity observed with functional magnetic resonance imaging".Nature Reviews. Neuroscience.8(9):700–11.doi:10.1038/nrn2201.PMID17704812.S2CID15979590.
- ^Laufs H, Krakow K, Sterzer P, Eger E, Beyerle A, Salek-Haddadi A, Kleinschmidt A (September 2003)."Electroencephalographic signatures of attentional and cognitive default modes in spontaneous brain activity fluctuations at rest".Proceedings of the National Academy of Sciences of the United States of America.100(19):11053–8.Bibcode:2003PNAS..10011053L.doi:10.1073/pnas.1831638100.PMC196925.PMID12958209.
- ^Mathewson KE, Gratton G, Fabiani M, Beck DM, Ro T (March 2009)."To see or not to see: prestimulus alpha phase predicts visual awareness".The Journal of Neuroscience.29(9):2725–32.doi:10.1523/JNEUROSCI.3963-08.2009.PMC2724892.PMID19261866.
- ^Busch NA, Dubois J, VanRullen R (June 2009)."The phase of ongoing EEG oscillations predicts visual perception".The Journal of Neuroscience.29(24):7869–76.doi:10.1523/jneurosci.0113-09.2009.PMC6665641.PMID19535598.
- ^van Dijk H, Schoffelen JM, Oostenveld R, Jensen O (February 2008)."Prestimulus oscillatory activity in the alpha band predicts visual discrimination ability".The Journal of Neuroscience.28(8):1816–23.doi:10.1523/jneurosci.1853-07.2008.PMC6671447.PMID18287498.
- ^Tallon-Baudry C, Bertrand O (April 1999). "Oscillatory gamma activity in humans and its role in object representation".Trends in Cognitive Sciences.3(4):151–162.doi:10.1016/S1364-6613(99)01299-1.PMID10322469.S2CID1308261.
- ^abPfurtscheller G, Lopes da Silva FH (November 1999). "Event-related EEG/MEG synchronization and desynchronization: basic principles".Clinical Neurophysiology.110(11):1842–57.doi:10.1016/S1388-2457(99)00141-8.PMID10576479.S2CID24756702.
- ^Tass PA (2007).Phase resetting in medicine and biology: stochastic modelling and data analysis.Berlin Heidelberg: Springer-Verlag.ISBN978-3-540-65697-5.
- ^Makeig S, Westerfield M, Jung TP, Enghoff S, Townsend J, Courchesne E, Sejnowski TJ (January 2002). "Dynamic brain sources of visual evoked responses".Science.295(5555):690–4.Bibcode:2002Sci...295..690M.doi:10.1126/science.1066168.PMID11809976.S2CID15200185.
- ^Mäkinen V, Tiitinen H, May P (February 2005). "Auditory event-related responses are generated independently of ongoing brain activity".NeuroImage.24(4):961–8.doi:10.1016/j.neuroimage.2004.10.020.PMID15670673.S2CID16210275.
- ^Nikulin VV, Linkenkaer-Hansen K, Nolte G, Lemm S,Müller KR,Ilmoniemi RJ, Curio G (May 2007). "A novel mechanism for evoked responses in the human brain".The European Journal of Neuroscience.25(10):3146–54.doi:10.1111/j.1460-9568.2007.05553.x.PMID17561828.S2CID12113334.
- ^Mazaheri A, Jensen O (July 2008)."Asymmetric amplitude modulations of brain oscillations generate slow evoked responses".The Journal of Neuroscience.28(31):7781–7.doi:10.1523/JNEUROSCI.1631-08.2008.PMC6670375.PMID18667610.
- ^Mazaheri A, Jensen O (2008)."Rhythmic pulsing: linking ongoing brain activity with evoked responses".Frontiers in Human Neuroscience.4:177.doi:10.3389/fnhum.2010.00177.PMC2972683.PMID21060804.
- ^Hamalainen M, Hari R, Ilmoniemi RJ, Knuutila J, Lounasmaa OV (1993)."Magnetoencephalography - Theory, instrumentation, and applications to noninvasive studies of the working human brain".Rev Mod Phys.65(2):413–497.Bibcode:1993RvMP...65..413H.doi:10.1103/RevModPhys.65.413.
- ^Hyafil, Alexandre; Giraud, Anne-Lise; Fontolan, Lorenzo; Gutkin, Boris (2015-11-01)."Neural Cross-Frequency Coupling: Connecting Architectures, Mechanisms, and Functions".Trends in Neurosciences.38(11):725–740.doi:10.1016/j.tins.2015.09.001.ISSN0166-2236.PMID26549886.S2CID3545001.
- ^Lisman, John E.; Jensen, Ole (2013-03-20)."The Theta-Gamma Neural Code".Neuron.77(6):1002–1016.doi:10.1016/j.neuron.2013.03.007.ISSN0896-6273.PMC3648857.PMID23522038.
- ^Drieu, Céline; Zugaro, Michaël (2019)."Hippocampal Sequences During Exploration: Mechanisms and Functions".Frontiers in Cellular Neuroscience.13:232.doi:10.3389/fncel.2019.00232.ISSN1662-5102.PMC6584963.PMID31263399.
- ^abSinger W (1993). "Synchronization of cortical activity and its putative role in information processing and learning".Annual Review of Physiology.55:349–74.doi:10.1146/annurev.ph.55.030193.002025.PMID8466179.
- ^Singer W, Gray CM (1995). "Visual feature integration and the temporal correlation hypothesis".Annual Review of Neuroscience.18:555–86.CiteSeerX10.1.1.308.6735.doi:10.1146/annurev.ne.18.030195.003011.PMID7605074.
- ^Marder E, Bucher D (November 2001)."Central pattern generators and the control of rhythmic movements".Current Biology.11(23): R986-96.Bibcode:2001CBio...11.R986M.doi:10.1016/S0960-9822(01)00581-4.PMID11728329.S2CID1294374.
- ^Dimitrijevic MR, Gerasimenko Y, Pinter MM (November 1998). "Evidence for a spinal central pattern generator in humans".Annals of the New York Academy of Sciences.860(1):360–76.Bibcode:1998NYASA.860..360D.doi:10.1111/j.1749-6632.1998.tb09062.x.PMID9928325.S2CID102514.
- ^Danner SM, Hofstoetter US, Freundl B, Binder H, Mayr W, Rattay F, Minassian K (March 2015)."Human spinal locomotor control is based on flexibly organized burst generators".Brain.138(Pt 3):577–88.doi:10.1093/brain/awu372.PMC4408427.PMID25582580.
- ^abGupta N, Singh SS, Stopfer M (December 2016)."Oscillatory integration windows in neurons".Nature Communications.7:13808.Bibcode:2016NatCo...713808G.doi:10.1038/ncomms13808.PMC5171764.PMID27976720.
- ^Ahissar, E., Nelinger, G., Assa, E., Karp, O. & Saraf-Sinik, I. Thalamocortical loops as temporal demodulators across senses. Communications Biology 6, 562 (2023).
- ^Milner PM (November 1974). "A model for visual shape recognition".Psychological Review.81(6):521–35.doi:10.1037/h0037149.PMID4445414.
- ^abGray CM, König P, Engel AK, Singer W (March 1989). "Oscillatory responses in cat visual cortex exhibit inter-columnar synchronization which reflects global stimulus properties".Nature.338(6213):334–7.Bibcode:1989Natur.338..334G.doi:10.1038/338334a0.PMID2922061.S2CID4281744.
- ^Eckhorn R, Bauer R, Jordan W, Brosch M, Kruse W, Munk M, Reitboeck HJ (1988). "Coherent oscillations: a mechanism of feature linking in the visual cortex? Multiple electrode and correlation analyses in the cat".Biological Cybernetics.60(2):121–30.doi:10.1007/BF00202899.PMID3228555.S2CID206771651.
- ^Wehr M, Laurent G (November 1996). "Odour encoding by temporal sequences of firing in oscillating neural assemblies".Nature.384(6605):162–6.Bibcode:1996Natur.384..162W.doi:10.1038/384162a0.PMID8906790.S2CID4286308.
- ^MacLeod K, Laurent G (November 1996). "Distinct mechanisms for synchronization and temporal patterning of odor-encoding neural assemblies".Science.274(5289):976–9.Bibcode:1996Sci...274..976M.doi:10.1126/science.274.5289.976.PMID8875938.S2CID10744144.
- ^Stopfer M, Bhagavan S, Smith BH, Laurent G (November 1997). "Impaired odour discrimination on desynchronization of odour-encoding neural assemblies".Nature.390(6655):70–4.Bibcode:1997Natur.390...70S.doi:10.1038/36335.PMID9363891.S2CID205024830.
- ^MacLeod K, Bäcker A, Laurent G (October 1998). "Who reads temporal information contained across synchronized and oscillatory spike trains?".Nature.395(6703):693–8.Bibcode:1998Natur.395..693M.doi:10.1038/27201.PMID9790189.S2CID4424801.
- ^Buhusi CV, Meck WH (October 2005). "What makes us tick? Functional and neural mechanisms of interval timing".Nature Reviews. Neuroscience.6(10):755–65.doi:10.1038/nrn1764.PMID16163383.S2CID29616055.
- ^Ahissar E, Zacksenhouse M (2001). "Chapter 6 Temporal and spatial coding in the rat vibrissal system".Advances in Neural Population Coding.Progress in Brain Research. Vol. 130. pp.75–87.doi:10.1016/S0079-6123(01)30007-9.ISBN9780444501103.PMID11480290.
{{cite book}}
:|journal=
ignored (help) - ^Burns SP, Xing D, Shapley RM (June 2011)."Is gamma-band activity in the local field potential of V1 cortex a" clock "or filtered noise?".The Journal of Neuroscience.31(26):9658–64.doi:10.1523/jneurosci.0660-11.2011.PMC3518456.PMID21715631.
- ^Pfurtscheller G, Aranibar A (June 1977). "Event-related cortical desynchronization detected by power measurements of scalp EEG".Electroencephalography and Clinical Neurophysiology.42(6):817–26.doi:10.1016/0013-4694(77)90235-8.PMID67933.
- ^Murthy VN, Fetz EE (December 1996). "Oscillatory activity in sensorimotor cortex of awake monkeys: synchronization of local field potentials and relation to behavior".Journal of Neurophysiology.76(6):3949–67.doi:10.1152/jn.1996.76.6.3949.PMID8985892.
- ^Sanes JN, Donoghue JP (May 1993)."Oscillations in local field potentials of the primate motor cortex during voluntary movement".Proceedings of the National Academy of Sciences of the United States of America.90(10):4470–4.Bibcode:1993PNAS...90.4470S.doi:10.1073/pnas.90.10.4470.PMC46533.PMID8506287.
- ^Conway BA, Halliday DM, Farmer SF, Shahani U, Maas P, Weir AI, Rosenberg JR (December 1995)."Synchronization between motor cortex and spinal motoneuronal pool during the performance of a maintained motor task in man".The Journal of Physiology.489 ( Pt 3) (3):917–24.doi:10.1113/jphysiol.1995.sp021104.PMC1156860.PMID8788955.
- ^Salenius S, Portin K, Kajola M, Salmelin R, Hari R (June 1997). "Cortical control of human motoneuron firing during isometric contraction".Journal of Neurophysiology.77(6):3401–5.doi:10.1152/jn.1997.77.6.3401.PMID9212286.S2CID2178927.
- ^Baker SN, Olivier E, Lemon RN (May 1997)."Coherent oscillations in monkey motor cortex and hand muscle EMG show task-dependent modulation".The Journal of Physiology.501 ( Pt 1) (1):225–41.doi:10.1111/j.1469-7793.1997.225bo.x.PMC1159515.PMID9175005.
- ^Boonstra TW, Danna-Dos-Santos A, Xie HB, Roerdink M, Stins JF, Breakspear M (December 2015)."Muscle networks: Connectivity analysis of EMG activity during postural control".Scientific Reports.5:17830.Bibcode:2015NatSR...517830B.doi:10.1038/srep17830.PMC4669476.PMID26634293.
- ^Kerkman JN, Daffertshofer A, Gollo LL, Breakspear M, Boonstra TW (June 2018)."Network structure of the human musculoskeletal system shapes neural interactions on multiple time scales".Science Advances.4(6): eaat0497.Bibcode:2018SciA....4..497K.doi:10.1126/sciadv.aat0497.PMC6021138.PMID29963631.
- ^Rubino D, Robbins KA, Hatsopoulos NG (December 2006). "Propagating waves mediate information transfer in the motor cortex".Nature Neuroscience.9(12):1549–57.doi:10.1038/nn1802.PMID17115042.S2CID16430438.
- ^Heitmann S, Boonstra T, Gong P, Breakspear M, Ermentrout B (2015). "The rhythms of steady posture: Motor commands as spatially organized oscillation patterns".Neurocomputing.170:3–14.doi:10.1016/j.neucom.2015.01.088.
- ^Heitmann S, Boonstra T, Breakspear M (October 2013)."A dendritic mechanism for decoding traveling waves: principles and applications to motor cortex".PLOS Computational Biology.9(10): e1003260.Bibcode:2013PLSCB...9E3260H.doi:10.1371/journal.pcbi.1003260.PMC3814333.PMID24204220.
- ^Allum JH, Dietz V, Freund HJ (May 1978). "Neuronal mechanisms underlying physiological tremor".Journal of Neurophysiology.41(3):557–71.doi:10.1152/jn.1978.41.3.557.PMID660226.
- ^Vallbo AB, Wessberg J (September 1993)."Organization of motor output in slow finger movements in man".The Journal of Physiology.469:673–91.doi:10.1113/jphysiol.1993.sp019837.PMC1143894.PMID8271223.
- ^Gross J, Timmermann L, Kujala J, Dirks M, Schmitz F, Salmelin R, Schnitzler A (February 2002)."The neural basis of intermittent motor control in humans".Proceedings of the National Academy of Sciences of the United States of America.99(4):2299–302.Bibcode:2002PNAS...99.2299G.doi:10.1073/pnas.032682099.PMC122359.PMID11854526.
- ^Buszaki G (2006).Rhythms of the brain.Oxford University Press.
- ^Nyhus E, Curran T (June 2010)."Functional role of gamma and theta oscillations in episodic memory".Neuroscience and Biobehavioral Reviews.34(7):1023–35.doi:10.1016/j.neubiorev.2009.12.014.PMC2856712.PMID20060015.
- ^Rutishauser U, Ross IB, Mamelak AN, Schuman EM (April 2010)."Human memory strength is predicted by theta-frequency phase-locking of single neurons"(PDF).Nature.464(7290):903–7.Bibcode:2010Natur.464..903R.doi:10.1038/nature08860.PMID20336071.S2CID4417989.
- ^Brancaccio A, Tabarelli D, Bigica M, Baldauf D (April 2020)."Cortical source localization of sleep-stage specific oscillatory activity".Scientific Reports.10(1): 6976.Bibcode:2020NatSR..10.6976B.doi:10.1038/s41598-020-63933-5.PMC7181624.PMID32332806.
- ^Feller MB(July 2009)."Retinal waves are likely to instruct the formation of eye-specific retinogeniculate projections".Neural Development.4:24.doi:10.1186/1749-8104-4-24.PMC2706239.PMID19580682.
- ^McAuley JH, Marsden CD (August 2000)."Physiological and pathological tremors and rhythmic central motor control".Brain.123 ( Pt 8) (8):1545–67.doi:10.1093/brain/123.8.1545.PMID10908186.
- ^Shusterman V, Troy WC (June 2008). "From baseline to epileptiform activity: a path to synchronized rhythmicity in large-scale neural networks".Physical Review E.77(6 Pt 1): 061911.Bibcode:2008PhRvE..77f1911S.doi:10.1103/PhysRevE.77.061911.PMID18643304.S2CID13928602.
- ^Vanneste S, Song JJ, De Ridder D (March 2018)."Thalamocortical dysrhythmia detected by machine learning".Nature Communications.9(1): 1103.Bibcode:2018NatCo...9.1103V.doi:10.1038/s41467-018-02820-0.PMC5856824.PMID29549239.
- ^Birbaumer N (November 2006)."Breaking the silence: brain-computer interfaces (BCI) for communication and motor control".Psychophysiology.43(6):517–32.doi:10.1111/j.1469-8986.2006.00456.x.PMID17076808.
- ^Vidal JJ (1973)."Toward direct brain-computer communication".Annual Review of Biophysics and Bioengineering.2:157–80.doi:10.1146/annurev.bb.02.060173.001105.PMID4583653.
- ^Bozinovski S, Sestakov M, Bozinovska L (November 1988). "Using EEG alpha rhythm to control a mobile robot.".Proceedings of the Annual International Conference of the IEEE Engineering in Medicine and Biology Society.New Orleans: IEEE. pp.1515–1516.doi:10.1109/IEMBS.1988.95357.ISBN0-7803-0785-2.S2CID62179588.
- ^Bozinovski S (August 1990). "Mobile robot trajectory control: From fixed rails to direct bioelectric control.". In Kaynak O (ed.).Proceedings of the IEEE International Workshop on Intelligent Motion Control.Vol. 2. Istanbul: IEEE. pp.463–467.doi:10.1109/IMC.1990.687362.S2CID60642344.
- ^Lebedev M (2016). "Augmentation of sensorimotor functions with neural prostheses".Opera Medica et Physiologica.2(3–4):211–227.doi:10.20388/OMP.003.0035(inactive 1 November 2024).
{{cite journal}}
:CS1 maint: DOI inactive as of November 2024 (link) - ^Lebedev MA, Nicolelis MA (April 2017). "Brain-Machine Interfaces: From Basic Science to Neuroprostheses and Neurorehabilitation".Physiological Reviews.97(2):767–837.doi:10.1152/physrev.00027.2016.PMID28275048.
Further reading
edit- Buzsáki G (2006).Rhythms of the Brain.Oxford University Press.ISBN978-0-19-530106-9.
- Freeman W (1975).Mass Action in the Nervous System.Academic Press.ISBN978-0124120471.Archived fromthe originalon 2015-07-05.