Binomial proportion confidence interval
Instatistics,abinomial proportion confidence intervalis aconfidence intervalfor the probability of success calculated from the outcome of a series of success–failure experiments (Bernoulli trials). In other words, a binomial proportion confidence interval is an interval estimate of a success probabilitywhen only the number of experimentsand the number of successesare known.
There are several formulas for a binomial confidence interval, but all of them rely on the assumption of abinomial distribution.In general, a binomial distribution applies when an experiment is repeated a fixed number of times, each trial of the experiment has two possible outcomes (success and failure), the probability of success is the same for each trial, and the trials arestatistically independent.Because the binomial distribution is adiscrete probability distribution(i.e., not continuous) and difficult to calculate for large numbers of trials, a variety of approximations are used to calculate this confidence interval, all with their own tradeoffs in accuracy and computational intensity.
A simple example of a binomial distribution is the set of various possible outcomes, and their probabilities, for the number of heads observed when acoin is flippedten times. The observed binomial proportion is the fraction of the flips that turn out to be heads. Given this observed proportion, the confidence interval for the true probability of the coin landing on heads is a range of possible proportions, which may or may not contain the true proportion. A 95% confidence interval for the proportion, for instance, will contain the true proportion 95% of the times that the procedure for constructing the confidence interval is employed.[1]
Problems with using a normal approximation or "Wald interval"[edit]

A commonly used formula for a binomial confidence interval relies on approximating the distribution of error about a binomially-distributed observation,,with anormal distribution.[3] The normal approximation depends on thede Moivre–Laplace theorem(the original,binomial-only version of thecentral limit theorem) and becomes unreliable when it violates the theorems' premises, as the sample size becomes small or the success probability grows close to either0or1.[4]
Using the normal approximation, the success probabilityis estimated by
whereis the proportion of successes in aBernoulli trialprocess and an estimator forin the underlyingBernoulli distribution.The equivalent formula in terms of observation counts is
where the data are the results oftrials that yieldedsuccesses andfailures. The distribution function argumentis thequantileof astandard normal distribution(i.e., theprobit) corresponding to the target error rateFor a 95% confidence level, the errorsoand
When using the Wald formula to estimate,or just considering the possible outcomes of this calculation, two problems immediately become apparent:
- First, forapproaching either1or0,the interval narrows to zero width (falsely implying certainty).
- Second, for values of(probability too low / too close to0), the interval boundaries exceed(overshoot).
(Another version of the second, overshoot problem, arises when insteadfalls below the same upper bound: probability too high / too close to1.)
An important theoretical derivation of this confidence interval involves the inversion of a hypothesis test. Under this formulation, the confidence interval represents those values of the population parameter that would have largevalues if they were tested as a hypothesizedpopulation proportion.[clarification needed]The collection of values,for which the normal approximation is valid can be represented as
whereis the lowerquantileof astandard normal distribution,vs.which is theupperquantile.
Since the test in the middle of the inequality is aWald test,the normal approximation interval is sometimes called theWald intervalorWald method,afterAbraham Wald,but it was first described byLaplace(1812).[5]
Bracketing the confidence interval[edit]
Extending the normal approximation and Wald-Laplace interval concepts,Michael Shorthas shown that inequalities on the approximation error between the binomial distribution and the normal distribution can be used to accurately bracket the estimate of the confidence interval around[6]
with
and whereis again the (unknown) proportion of successes in a Bernoulli trial process (as opposed tothat estimates it) measured withtrials yieldingsuccesses,is thequantile of a standard normal distribution (i.e., the probit) corresponding to the target error rateand the constantsandare simple algebraic functions of[6]For a fixed(and hence), the above inequalities give easily computed one- or two-sided intervals which bracket the exact binomial upper and lower confidence limits corresponding to the error rate
Standard error of a proportion estimation when using weighted data[edit]
Let there be a simple random samplewhere eachisi.i.dfrom aBernoulli(p) distribution and weightis the weight for each observation, with the(positive) weightsnormalized so they sum to1.Theweighted sample proportionis:Since each of theis independent from all the others, and each one has variancefor everythesampling variance of the proportiontherefore is:[7]
Thestandard errorofis the square root of this quantity. Because we do not knowwe have to estimate it. Although there are many possible estimators, a conventional one is to usethe sample mean, and plug this into the formula. That gives:
For otherwise unweighted data, the effective weights are uniformgivingThebecomesleading to the familiar formulas, showing that the calculation for weighted data is a direct generalization of them.
Wilson score interval[edit]
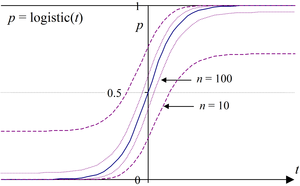
TheWilson score intervalwas developed byE.B. Wilson(1927).[8] It is an improvement over the normal approximation interval in multiple respects: Unlike the symmetric normal approximation interval (above), the Wilson score interval isasymmetric,and it doesn't suffer from problems ofovershootandzero-width intervalsthat afflict the normal interval. It can be safely employed with small samples and skewed observations.[3]The observedcoverage probabilityis consistently closer to the nominal value,[2]
Like the normal interval, the interval can be computed directly from a formula.
Wilson started with the normal approximation to the binomial:
whereis the standard normal interval half-width corresponding to the desired confidenceThe analytic formula for a binomial sample standard deviation is
- or
Transforming the relation into a standard-form quadratic equation fortreatingandas known values from the sample (see prior section), and using the value ofthat corresponds to the desired confidencefor the estimate ofgives this:
whereis an abbreviation for
An equivalent expression using the observation countsandis
with the counts as above:the count of observed "successes",the count of observed "failures", and their sum is the total number of observations
In practical tests of the formula's results, users find that this interval has good properties even for a small number of trials and / or the extremes of the probability estimate,[2][3][9]
Intuitively, the center value of this interval is the weighted average ofandwithreceiving greater weight as the sample size increases. Formally, the center value corresponds to using apseudocountofthe number of standard deviations of the confidence interval: Add this number to both the count of successes and of failures to yield the estimate of the ratio. For the common two standard deviations in each direction interval (approximately 95% coverage, which itself is approximately 1.96 standard deviations), this yields the estimatewhich is known as the "plus four rule".
Although the quadratic can be solved explicitly, in most cases Wilson's equations can also be solved numerically using the fixed-point iteration
with
The Wilson interval can also be derived from thesingle sample z-testorPearson's chi-squared testwith two categories. The resulting interval,
(withthe lowerquantile) can then be solved forto produce the Wilson score interval. The test in the middle of the inequality is ascore test.
The interval equality principle[edit]

Since the interval is derived by solving from the normal approximation to the binomial, the Wilson score intervalhas the property of being guaranteed to obtain the same result as the equivalentz-testorchi-squared test.
This property can be visualised by plotting theprobability density functionfor the Wilson score interval (seeWallis).[9](pp 297-313) After that, then also plotting a normalPDFacross each bound. The tail areas of the resulting Wilson and normal distributions represent the chance of a significant result, in that direction, must be equal.
The continuity-corrected Wilson score interval and theClopper-Pearson intervalare also compliant with this property. The practical import is that these intervals may be employed assignificance tests,with identical results to the source test, and new tests may be derived by geometry.[9]
Wilson score interval with continuity correction[edit]
The Wilson interval may be modified by employing acontinuity correction,in order to align the minimumcoverage probability,rather than the average coverage probability, with the nominal value,
Just as the Wilson interval mirrorsPearson's chi-squared test,the Wilson interval with continuity correction mirrors the equivalentYates' chi-squared test.
The following formulae for the lower and upper bounds of the Wilson score interval with continuity correctionare derived from Newcombe:[2]
forand
Ifthenmust instead be set toifthenmust be instead set to
Wallis (2021)[9]identifies a simpler method for computing continuity-corrected Wilson intervals that employs a special function based on Wilson's lower-bound formula: In Wallis' notation, for the lower bound, let
whereis the selected tolerable error level forThen
This method has the advantage of being further decomposable.
Jeffreys interval[edit]
TheJeffreys intervalhas a Bayesian derivation, but good frequentist properties (outperforming most frequentist constructions). In particular, it has coverage properties that are similar to those of the Wilson interval, but it is one of the few intervals with the advantage of beingequal-tailed(e.g., for a 95% confidence interval, the probabilities of the interval lying above or below the true value are both close to 2.5%). In contrast, the Wilson interval has a systematic bias such that it is centred too close to[10]
The Jeffreys interval is the Bayesiancredible intervalobtained when using thenon-informativeJeffreys priorfor the binomial proportionTheJeffreys prior for this problemis aBeta distributionwith parametersaconjugate prior.After observingsuccesses intrials, theposterior distributionforis a Beta distribution with parameters
Whenandthe Jeffreys interval is taken to be theequal-tailed posterior probability interval, i.e., theandquantiles of a Beta distribution with parameters
In order to avoid the coverage probability tending to zero whenor1,whenthe upper limit is calculated as before but the lower limit is set to0,and whenthe lower limit is calculated as before but the upper limit is set to1.[4]
Jeffreys' interval can also be thought of as a frequentist interval based on inverting the p-value from theG-testafter applying theYates correctionto avoid a potentially-infinite value for the test statistic.
Clopper–Pearson interval[edit]
The Clopper–Pearson interval is an early and very common method for calculating binomial confidence intervals.[11] This is often called an 'exact' method, as it attains the nominal coverage level in an exact sense, meaning that the coverage level is never less than the nominal[2]
The Clopper–Pearson interval can be written as
or equivalently,
with
and
whereis the number of successes observed in the sample andis a binomial random variable withtrials and probability of success
Equivalently we can say that the Clopper–Pearson interval iswith confidence levelifis the infimum of those such that the following tests of hypothesis succeed with significance
- H0:with HA:
- H0:with HA:
Because of a relationship between the binomial distribution and thebeta distribution,the Clopper–Pearson interval is sometimes presented in an alternate format that uses quantiles from the beta distribution.[12]
whereis the number of successes,is the number of trials, andis thepthquantilefrom a beta distribution with shape parametersand
Thus,where:
The binomial proportion confidence interval is thenas follows from the relation between theBinomial distribution cumulative distribution functionand theregularized incomplete beta function.
Whenis either0orclosed-form expressions for the interval bounds are available: whenthe interval is
and when it is
The beta distribution is, in turn, related to theF-distributionso a third formulation of the Clopper–Pearson interval can be written usingFquantiles:
whereis the number of successes,is the number of trials, andis thequantile from anF-distributionwithanddegrees of freedom.[13]
The Clopper–Pearson interval is an 'exact' interval, since it is based directly on the binomial distribution rather than any approximation to the binomial distribution. This interval never has less than the nominal coverage for any population proportion, but that means that it is usually conservative. For example, the true coverage rate of a 95% Clopper–Pearson interval may be well above 95%, depending onand[4]Thus the interval may be wider than it needs to be to achieve 95% confidence, and wider than other intervals. In contrast, it is worth noting that other confidence interval may have coverage levels that are lower than the nominali.e., the normal approximation (or "standard" ) interval, Wilson interval,[8]Agresti–Coull interval,[13] etc., with a nominal coverage of 95% may in fact cover less than 95%,[4]even for large sample sizes.[12]
The definition of the Clopper–Pearson interval can also be modified to obtain exact confidence intervals for different distributions. For instance, it can also be applied to the case where the samples are drawn without replacement from a population of a known size, instead of repeated draws of a binomial distribution. In this case, the underlying distribution would be thehypergeometric distribution.
The interval boundaries can be computed with numerical functionsqbeta[14] in R and scipy.stats.beta.ppf[15] in Python.
fromscipy.statsimportbeta
k=20
n=400
alpha=0.05
p_u,p_o=beta.ppf([alpha/2,1-alpha/2],[k,k+1],[n-k+1,n-k])
Agresti–Coull interval[edit]
The Agresti–Coull interval is also another approximate binomial confidence interval.[13]
Givensuccesses intrials, define
and
Then, a confidence interval foris given by
whereis the quantile of a standard normal distribution, as before (for example, a 95% confidence interval requiresthereby producing). According toBrown,Cai,& DasGupta (2001),[4]takinginstead of 1.96 produces the "add 2 successes and 2 failures" interval previously described byAgresti&Coull.[13]
This interval can be summarised as employing the centre-point adjustment,of the Wilson score interval, and then applying the Normal approximation to this point.[3][4]
Arcsine transformation[edit]
The arcsine transformation has the effect of pulling out the ends of the distribution.[16] While it can stabilize the variance (and thus confidence intervals) of proportion data, its use has been criticized in several contexts.[17]
Letbe the number of successes intrials and letThe variance ofis
Using thearc sinetransform, the variance of the arcsine ofis[18]
So, the confidence interval itself has the form
whereis thequantile of a standard normal distribution.
This method may be used to estimate the variance ofbut its use is problematic whenis close to0or1.
tatransform[edit]
Letbe the proportion of successes. For
This family is a generalisation of the logit transform which is a special case witha= 1 and can be used to transform a proportional data distribution to an approximatelynormal distribution.The parameterahas to be estimated for the data set.
Rule of three — for when no successes are observed[edit]
Therule of threeis used to provide a simple way of stating an approximate 95% confidence interval forin the special case that no successes () have been observed.[19] The interval is
By symmetry, in the case of only successes (), the interval is
Comparison and discussion[edit]
There are several research papers that compare these and other confidence intervals for the binomial proportion.[3][2][20][21]
Both Ross (2003)[22] and Agresti & Coull (1998)[13] point out that exact methods such as the Clopper–Pearson interval may not work as well as some approximations. The normal approximation interval and its presentation in textbooks has been heavily criticised, with many statisticians advocating that it not be used.[4] The principal problems areovershoot(bounds exceed),zero-width intervalsator1(falsely implying certainty),[2]and overall inconsistency with significance testing.[3]
Of the approximations listed above, Wilson score interval methods (with or without continuity correction) have been shown to be the most accurate and the most robust,[3][4][2]though some prefer Agresti & Coulls' approach for larger sample sizes.[4]Wilson and Clopper–Pearson methods obtain consistent results with source significance tests,[9]and this property is decisive for many researchers.
Many of these intervals can be calculated inRusing packages likebinom.[23]
See also[edit]
- binomial distribution#Confidence intervals
- estimation theory
- pseudocount
- CDF-based_nonparametric_confidence_interval#Pointwise_band
References[edit]
- ^ Sullivan, Lisa (2017-10-27)."Confidence Intervals".sphweb.bumc.bu.edu(course notes). Boston, MA:Boston UniversitySchool of Public Health. BS704.
- ^abcdefgh Newcombe, R.G. (1998). "Two-sided confidence intervals for the single proportion: Comparison of seven methods".Statistics in Medicine.17(8): 857–872.doi:10.1002/(SICI)1097-0258(19980430)17:8<857::AID-SIM777>3.0.CO;2-E.PMID9595616.
- ^abcdefg Wallis, Sean A. (2013)."Binomial confidence intervals and contingency tests: Mathematical fundamentals and the evaluation of alternative methods"(PDF).Journal of Quantitative Linguistics.20(3): 178–208.doi:10.1080/09296174.2013.799918.S2CID16741749.
- ^abcdefghi Brown, Lawrence D.;Cai, T. Tony;DasGupta, Anirban (2001). "Interval estimation for a binomial proportion".Statistical Science.16(2): 101–133.CiteSeerX10.1.1.50.3025.doi:10.1214/ss/1009213286.MR1861069.Zbl1059.62533.
- ^ Laplace, P.S.(1812).Théorie analytique des probabilités[Analyitic Probability Theory] (in French). Ve. Courcier. p. 283.
- ^ab Short, Michael (2021-11-08)."On binomial quantile and proportion bounds: With applications in engineering and informatics".Communications in Statistics - Theory and Methods.52(12): 4183–4199.doi:10.1080/03610926.2021.1986540.ISSN0361-0926.S2CID243974180.
- ^"How to calculate the standard error of a proportion using weighted data?".stats.stackexchange.com.159220 / 253.
- ^ab Wilson, E.B.(1927). "Probable inference, the law of succession, and statistical inference".Journal of the American Statistical Association.22(158): 209–212.doi:10.1080/01621459.1927.10502953.JSTOR2276774.
- ^abcde Wallis, Sean A. (2021).Statistics in Corpus Linguistics: A new approach.New York, NY: Routledge.ISBN9781138589384.
- ^ Cai, T.T.(2005). "One-sided confidence intervals in discrete distributions".Journal of Statistical Planning and Inference.131(1): 63–88.doi:10.1016/j.jspi.2004.01.005.
- ^ Clopper, C.;Pearson, E.S.(1934). "The use of confidence or fiducial limits illustrated in the case of the binomial".Biometrika.26(4): 404–413.doi:10.1093/biomet/26.4.404.
- ^abc Thulin, Måns (2014-01-01). "The cost of using exact confidence intervals for a binomial proportion".Electronic Journal of Statistics.8(1): 817–840.arXiv:1303.1288.doi:10.1214/14-EJS909.ISSN1935-7524.S2CID88519382.
- ^abcde Agresti, Alan;Coull, Brent A. (1998). "Approximate is better than 'exact' for interval estimation of binomial proportions".The American Statistician.52(2): 119–126.doi:10.2307/2685469.JSTOR2685469.MR1628435.
- ^ "The Beta distribution".stat.ethz.ch(software doc). R Manual.Retrieved2023-12-02.
- ^ "scipy.stats.beta".SciPy Manual.docs.scipy.org(software doc) (1.11.4 ed.).Retrieved2023-12-02.
- ^ Holland, Steven."Transformations of proportions and percentages".strata.uga.edu.Retrieved2020-09-08.
- ^ Warton, David I.; Hui, Francis K.C. (January 2011)."The arcsine is asinine: The analysis of proportions in ecology".Ecology.92(1): 3–10.Bibcode:2011Ecol...92....3W.doi:10.1890/10-0340.1.hdl:1885/152287.ISSN0012-9658.PMID21560670.
- ^ Shao, J. (1998).Mathematical Statistics.New York, NY: Springer.
- ^ Simon, Steve (2010)."Confidence interval with zero events".Ask Professor Mean. Kansas City, MO: The Children's Mercy Hospital. Archived fromthe originalon 15 October 2011.Stats topics on Medical Research
- ^ Sauro, J.; Lewis, J.R. (2005).Comparison of Wald, Adj-Wald, exact, and Wilson intervals calculator(PDF).Human Factors and Ergonomics Society, 49th Annual Meeting (HFES 2005). Orlando, FL. pp. 2100–2104. Archived fromthe original(PDF)on 18 June 2012.
- ^ Reiczigel, J. (2003)."Confidence intervals for the binomial parameter: Some new considerations"(PDF).Statistics in Medicine.22(4): 611–621.doi:10.1002/sim.1320.PMID12590417.S2CID7715293.
- ^ Ross, T.D. (2003)."Accurate confidence intervals for binomial proportion and Poisson rate estimation".Computers in Biology and Medicine.33(6): 509–531.doi:10.1016/S0010-4825(03)00019-2.PMID12878234.
- ^ Dorai-Raj, Sundar (2 May 2022).binom: Binomial confidence intervals for several parameterizations(software doc.).Retrieved2 December2023.