Linear algebra
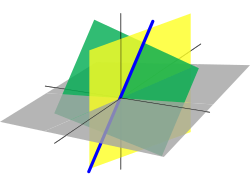
Linear algebrais the branch ofmathematicsconcerninglinear equationssuch as:
linear mapssuch as:
and their representations invector spacesand throughmatrices.[1][2][3]
Linear algebra is central to almost all areas of mathematics. For instance, linear algebra is fundamental in modern presentations ofgeometry,including for defining basic objects such aslines,planesandrotations.Also,functional analysis,a branch ofmathematical analysis,may be viewed as the application of linear algebra tofunction spaces.
Linear algebra is also used in most sciences and fields ofengineering,because it allowsmodelingmany natural phenomena, and computing efficiently with such models. Fornonlinear systems,which cannot be modeled with linear algebra, it is often used for dealing withfirst-order approximations,using the fact that thedifferentialof amultivariate functionat a point is the linear map that best approximates the function near that point.
History
[edit]The procedure (using counting rods) for solving simultaneous linear equations now calledGaussian eliminationappears in the ancient Chinese mathematical textChapter Eight:Rectangular ArraysofThe Nine Chapters on the Mathematical Art.Its use is illustrated in eighteen problems, with two to five equations.[4]
Systems of linear equationsarose in Europe with the introduction in 1637 byRené Descartesofcoordinatesingeometry.In fact, in this new geometry, now calledCartesian geometry,lines and planes are represented by linear equations, and computing their intersections amounts to solving systems of linear equations.
The first systematic methods for solving linear systems useddeterminantsand were first considered byLeibnizin 1693. In 1750,Gabriel Cramerused them for giving explicit solutions of linear systems, now calledCramer's rule.Later,Gaussfurther described the method of elimination, which was initially listed as an advancement ingeodesy.[5]
In 1844Hermann Grassmannpublished his "Theory of Extension" which included foundational new topics of what is today called linear algebra. In 1848,James Joseph Sylvesterintroduced the termmatrix,which is Latin forwomb.
Linear algebra grew with ideas noted in thecomplex plane.For instance, two numberswandzinhave a differencew–z,and the line segmentswzand0(w−z)are of the same length and direction. The segments areequipollent.The four-dimensional systemofquaternionswas discovered byW.R. Hamiltonin 1843.[6]The termvectorwas introduced asv=xi+yj+zkrepresenting a point in space. The quaternion differencep–qalso produces a segment equipollent topq.Otherhypercomplex numbersystems also used the idea of a linear space with abasis.
Arthur Cayleyintroducedmatrix multiplicationand theinverse matrixin 1856, making possible thegeneral linear group.The mechanism ofgroup representationbecame available for describing complex and hypercomplex numbers. Crucially, Cayley used a single letter to denote a matrix, thus treating a matrix as an aggregate object. He also realized the connection between matrices and determinants, and wrote "There would be many things to say about this theory of matrices which should, it seems to me, precede the theory of determinants".[5]
Benjamin Peircepublished hisLinear Associative Algebra(1872), and his sonCharles Sanders Peirceextended the work later.[7]
Thetelegraphrequired an explanatory system, and the 1873 publication ofA Treatise on Electricity and Magnetisminstituted afield theoryof forces and requireddifferential geometryfor expression. Linear algebra is flat differential geometry and serves in tangent spaces tomanifolds.Electromagnetic symmetries of spacetime are expressed by theLorentz transformations,and much of the history of linear algebra is thehistory of Lorentz transformations.
The first modern and more precise definition of a vector space was introduced byPeanoin 1888;[5]by 1900, a theory of linear transformations of finite-dimensional vector spaces had emerged. Linear algebra took its modern form in the first half of the twentieth century, when many ideas and methods of previous centuries were generalized asabstract algebra.The development of computers led to increased research in efficientalgorithmsfor Gaussian elimination and matrix decompositions, and linear algebra became an essential tool for modelling and simulations.[5]
Vector spaces
[edit]Until the 19th century, linear algebra was introduced throughsystems of linear equationsandmatrices.In modern mathematics, the presentation throughvector spacesis generally preferred, since it is moresynthetic,more general (not limited to the finite-dimensional case), and conceptually simpler, although more abstract.
A vector space over afieldF(often the field of thereal numbers) is asetVequipped with twobinary operations.ElementsofVare calledvectors,and elements ofFare calledscalars.The first operation,vector addition,takes any two vectorsvandwand outputs a third vectorv+w.The second operation,scalar multiplication,takes any scalaraand any vectorvand outputs a newvectorav.The axioms that addition and scalar multiplication must satisfy are the following. (In the list below,u,vandware arbitrary elements ofV,andaandbare arbitrary scalars in the fieldF.)[8]
Axiom Signification Associativityof addition u+ (v+w) = (u+v) +w Commutativityof addition u+v=v+u Identity elementof addition There exists an element0inV,called thezero vector(or simplyzero), such thatv+0=vfor allvinV. Inverse elementsof addition For everyvinV,there exists an element−vinV,called theadditive inverseofv,such thatv+ (−v) =0 Distributivityof scalar multiplication with respect to vector addition a(u+v) =au+av Distributivity of scalar multiplication with respect to field addition (a+b)v=av+bv Compatibility of scalar multiplication with field multiplication a(bv) = (ab)v[a] Identity element of scalar multiplication 1v=v,where1denotes themultiplicative identityofF.
The first four axioms mean thatVis anabelian groupunder addition.
An element of a specific vector space may have various nature; for example, it could be asequence,afunction,apolynomialor amatrix.Linear algebra is concerned with those properties of such objects that are common to all vector spaces.
Linear maps
[edit]Linear mapsaremappingsbetween vector spaces that preserve the vector-space structure. Given two vector spacesVandWover a fieldF,a linear map (also called, in some contexts, linear transformation or linear mapping) is amap
that is compatible with addition and scalar multiplication, that is
for any vectorsu,vinVand scalarainF.
This implies that for any vectorsu,vinVand scalarsa,binF,one has
WhenV=Ware the same vector space, a linear mapT:V→Vis also known as alinear operatoronV.
Abijectivelinear map between two vector spaces (that is, every vector from the second space is associated with exactly one in the first) is anisomorphism.Because an isomorphism preserves linear structure, two isomorphic vector spaces are "essentially the same" from the linear algebra point of view, in the sense that they cannot be distinguished by using vector space properties. An essential question in linear algebra is testing whether a linear map is an isomorphism or not, and, if it is not an isomorphism, finding itsrange(or image) and the set of elements that are mapped to the zero vector, called thekernelof the map. All these questions can be solved by usingGaussian eliminationor some variant of thisalgorithm.
Subspaces, span, and basis
[edit]The study of those subsets of vector spaces that are in themselves vector spaces under the induced operations is fundamental, similarly as for many mathematical structures. These subsets are calledlinear subspaces.More precisely, a linear subspace of a vector spaceVover a fieldFis asubsetWofVsuch thatu+vandauare inW,for everyu,vinW,and everyainF.(These conditions suffice for implying thatWis a vector space.)
For example, given a linear mapT:V→W,theimageT(V)ofV,and theinverse imageT−1(0)of0(calledkernelor null space), are linear subspaces ofWandV,respectively.
Another important way of forming a subspace is to considerlinear combinationsof a setSof vectors: the set of all sums
wherev1,v2,...,vkare inS,anda1,a2,...,akare inFform a linear subspace called thespanofS.The span ofSis also the intersection of all linear subspaces containingS.In other words, it is the smallest (for the inclusion relation) linear subspace containingS.
A set of vectors islinearly independentif none is in the span of the others. Equivalently, a setSof vectors is linearly independent if the only way to express the zero vector as a linear combination of elements ofSis to take zero for every coefficientai.
A set of vectors that spans a vector space is called aspanning setorgenerating set.If a spanning setSislinearly dependent(that is not linearly independent), then some elementwofSis in the span of the other elements ofS,and the span would remain the same if one removewfromS.One may continue to remove elements ofSuntil getting alinearly independent spanning set.Such a linearly independent set that spans a vector spaceVis called abasisofV.The importance of bases lies in the fact that they are simultaneously minimal generating sets and maximal independent sets. More precisely, ifSis a linearly independent set, andTis a spanning set such thatS⊆T,then there is a basisBsuch thatS⊆B⊆T.
Any two bases of a vector spaceVhave the samecardinality,which is called thedimensionofV;this is thedimension theorem for vector spaces.Moreover, two vector spaces over the same fieldFareisomorphicif and only if they have the same dimension.[9]
If any basis ofV(and therefore every basis) has a finite number of elements,Vis afinite-dimensional vector space.IfUis a subspace ofV,thendimU≤ dimV.In the case whereVis finite-dimensional, the equality of the dimensions impliesU=V.
IfU1andU2are subspaces ofV,then
whereU1+U2denotes the span ofU1∪U2.[10]
Matrices
[edit]Matrices allow explicit manipulation of finite-dimensional vector spaces andlinear maps.Their theory is thus an essential part of linear algebra.
LetVbe a finite-dimensional vector space over a fieldF,and(v1,v2,...,vm)be a basis ofV(thusmis the dimension ofV). By definition of a basis, the map
is abijectionfromFm,the set of thesequencesofmelements ofF,ontoV.This is anisomorphismof vector spaces, ifFmis equipped of its standard structure of vector space, where vector addition and scalar multiplication are done component by component.
This isomorphism allows representing a vector by itsinverse imageunder this isomorphism, that is by thecoordinate vector(a1,...,am)or by thecolumn matrix
IfWis another finite dimensional vector space (possibly the same), with a basis(w1,...,wn),a linear mapffromWtoVis well defined by its values on the basis elements, that is(f(w1),...,f(wn)).Thus,fis well represented by the list of the corresponding column matrices. That is, if
forj= 1,...,n,thenfis represented by the matrix
withmrows andncolumns.
Matrix multiplicationis defined in such a way that the product of two matrices is the matrix of thecompositionof the corresponding linear maps, and the product of a matrix and a column matrix is the column matrix representing the result of applying the represented linear map to the represented vector. It follows that the theory of finite-dimensional vector spaces and the theory of matrices are two different languages for expressing exactly the same concepts.
Two matrices that encode the same linear transformation in different bases are calledsimilar.It can be proved that two matrices are similar if and only if one can transform one into the other byelementary row and column operations.For a matrix representing a linear map fromWtoV,the row operations correspond to change of bases inVand the column operations correspond to change of bases inW.Every matrix is similar to anidentity matrixpossibly bordered by zero rows and zero columns. In terms of vector spaces, this means that, for any linear map fromWtoV,there are bases such that a part of the basis ofWis mapped bijectively on a part of the basis ofV,and that the remaining basis elements ofW,if any, are mapped to zero.Gaussian eliminationis the basic algorithm for finding these elementary operations, and proving these results.
Linear systems
[edit]A finite set of linear equations in a finite set of variables, for example,x1,x2,...,xn,orx,y,...,zis called asystem of linear equationsor alinear system.[11][12][13][14][15]
Systems of linear equations form a fundamental part of linear algebra. Historically, linear algebra and matrix theory has been developed for solving such systems. In the modern presentation of linear algebra through vector spaces and matrices, many problems may be interpreted in terms of linear systems.
For example, let
(S) |
be a linear system.
To such a system, one may associate its matrix
and its right member vector
LetTbe the linear transformation associated to the matrixM.A solution of the system (S) is a vector
such that
that is an element of thepreimageofvbyT.
Let (S′) be the associatedhomogeneous system,where the right-hand sides of the equations are put to zero:
(S′) |
The solutions of (S′) are exactly the elements of thekernelofTor, equivalently,M.
TheGaussian-eliminationconsists of performingelementary row operationson theaugmented matrix
for putting it inreduced row echelon form.These row operations do not change the set of solutions of the system of equations. In the example, the reduced echelon form is
showing that the system (S) has the unique solution
It follows from this matrix interpretation of linear systems that the same methods can be applied for solving linear systems and for many operations on matrices and linear transformations, which include the computation of theranks,kernels,matrix inverses.
Endomorphisms and square matrices
[edit]A linearendomorphismis a linear map that maps a vector spaceVto itself. IfVhas a basis ofnelements, such an endomorphism is represented by a square matrix of sizen.
With respect to general linear maps, linear endomorphisms and square matrices have some specific properties that make their study an important part of linear algebra, which is used in many parts of mathematics, includinggeometric transformations,coordinate changes,quadratic forms,and many other part of mathematics.
Determinant
[edit]Thedeterminantof a square matrixAis defined to be[16]
whereSnis thegroup of all permutationsofnelements,σis a permutation, and(−1)σtheparityof the permutation. A matrix isinvertibleif and only if the determinant is invertible (i.e., nonzero if the scalars belong to a field).
Cramer's ruleis aclosed-form expression,in terms of determinants, of the solution of asystem ofnlinear equations innunknowns.Cramer's rule is useful for reasoning about the solution, but, except forn= 2or3,it is rarely used for computing a solution, sinceGaussian eliminationis a faster algorithm.
Thedeterminant of an endomorphismis the determinant of the matrix representing the endomorphism in terms of some ordered basis. This definition makes sense, since this determinant is independent of the choice of the basis.
Eigenvalues and eigenvectors
[edit]Iffis a linear endomorphism of a vector spaceVover a fieldF,aneigenvectoroffis a nonzero vectorvofVsuch thatf(v) =avfor some scalarainF.This scalarais aneigenvalueoff.
If the dimension ofVis finite, and a basis has been chosen,fandvmay be represented, respectively, by a square matrixMand a column matrixz;the equation defining eigenvectors and eigenvalues becomes
Using theidentity matrixI,whose entries are all zero, except those of the main diagonal, which are equal to one, this may be rewritten
Aszis supposed to be nonzero, this means thatM–aIis asingular matrix,and thus that its determinantdet (M−aI)equals zero. The eigenvalues are thus therootsof thepolynomial
IfVis of dimensionn,this is amonic polynomialof degreen,called thecharacteristic polynomialof the matrix (or of the endomorphism), and there are, at most,neigenvalues.
If a basis exists that consists only of eigenvectors, the matrix offon this basis has a very simple structure: it is adiagonal matrixsuch that the entries on themain diagonalare eigenvalues, and the other entries are zero. In this case, the endomorphism and the matrix are said to bediagonalizable.More generally, an endomorphism and a matrix are also said diagonalizable, if they become diagonalizable afterextendingthe field of scalars. In this extended sense, if the characteristic polynomial issquare-free,then the matrix is diagonalizable.
Asymmetric matrixis always diagonalizable. There are non-diagonalizable matrices, the simplest being
(it cannot be diagonalizable since its square is thezero matrix,and the square of a nonzero diagonal matrix is never zero).
When an endomorphism is not diagonalizable, there are bases on which it has a simple form, although not as simple as the diagonal form. TheFrobenius normal formdoes not need of extending the field of scalars and makes the characteristic polynomial immediately readable on the matrix. TheJordan normal formrequires to extend the field of scalar for containing all eigenvalues, and differs from the diagonal form only by some entries that are just above the main diagonal and are equal to 1.
Duality
[edit]Alinear formis a linear map from a vector spaceVover a fieldFto the field of scalarsF,viewed as a vector space over itself. Equipped bypointwiseaddition and multiplication by a scalar, the linear forms form a vector space, called thedual spaceofV,and usually denotedV*[17]orV′.[18][19]
Ifv1,...,vnis a basis ofV(this implies thatVis finite-dimensional), then one can define, fori= 1,...,n,a linear mapvi*such thatvi*(vi) = 1andvi*(vj) = 0ifj≠i.These linear maps form a basis ofV*,called thedual basisofv1,...,vn.(IfVis not finite-dimensional, thevi*may be defined similarly; they are linearly independent, but do not form a basis.)
ForvinV,the map
is a linear form onV*.This defines thecanonical linear mapfromVinto(V*)*,the dual ofV*,called thebidualofV.This canonical map is anisomorphismifVis finite-dimensional, and this allows identifyingVwith its bidual. (In the infinite dimensional case, the canonical map is injective, but not surjective.)
There is thus a complete symmetry between a finite-dimensional vector space and its dual. This motivates the frequent use, in this context, of thebra–ket notation
for denotingf(x).
Dual map
[edit]Let
be a linear map. For every linear formhonW,thecomposite functionh∘fis a linear form onV.This defines a linear map
between the dual spaces, which is called thedualor thetransposeoff.
IfVandWare finite dimensional, andMis the matrix offin terms of some ordered bases, then the matrix off*over the dual bases is thetransposeMTofM,obtained by exchanging rows and columns.
If elements of vector spaces and their duals are represented by column vectors, this duality may be expressed inbra–ket notationby
For highlighting this symmetry, the two members of this equality are sometimes written
Inner-product spaces
[edit]Besides these basic concepts, linear algebra also studies vector spaces with additional structure, such as aninner product.The inner product is an example of abilinear form,and it gives the vector space a geometric structure by allowing for the definition of length and angles. Formally, aninner productis a map
that satisfies the following threeaxiomsfor all vectorsu,v,winVand all scalarsainF:[20][21]
- Conjugatesymmetry:
- In,it is symmetric.
- Linearityin the first argument:
- Positive-definiteness:
- with equality only forv= 0.
We can define the length of a vectorvinVby
and we can prove theCauchy–Schwarz inequality:
In particular, the quantity
and so we can call this quantity the cosine of the angle between the two vectors.
Two vectors are orthogonal if⟨u,v⟩ = 0.An orthonormal basis is a basis where all basis vectors have length 1 and are orthogonal to each other. Given any finite-dimensional vector space, an orthonormal basis could be found by theGram–Schmidtprocedure. Orthonormal bases are particularly easy to deal with, since ifv=a1v1+ ⋯ +anvn,then
The inner product facilitates the construction of many useful concepts. For instance, given a transformT,we can define itsHermitian conjugateT*as the linear transform satisfying
IfTsatisfiesTT*=T*T,we callTnormal.It turns out that normal matrices are precisely the matrices that have an orthonormal system of eigenvectors that spanV.
Relationship with geometry
[edit]There is a strong relationship between linear algebra andgeometry,which started with the introduction byRené Descartes,in 1637, ofCartesian coordinates.In this new (at that time) geometry, now calledCartesian geometry,points are represented byCartesian coordinates,which are sequences of three real numbers (in the case of the usualthree-dimensional space). The basic objects of geometry, which arelinesandplanesare represented by linear equations. Thus, computing intersections of lines and planes amounts to solving systems of linear equations. This was one of the main motivations for developing linear algebra.
Mostgeometric transformation,such astranslations,rotations,reflections,rigid motions,isometries,andprojectionstransform lines into lines. It follows that they can be defined, specified and studied in terms of linear maps. This is also the case ofhomographiesandMöbius transformations,when considered as transformations of aprojective space.
Until the end of the 19th century, geometric spaces were defined byaxiomsrelating points, lines and planes (synthetic geometry). Around this date, it appeared that one may also define geometric spaces by constructions involving vector spaces (see, for example,Projective spaceandAffine space). It has been shown that the two approaches are essentially equivalent.[22]In classical geometry, the involved vector spaces are vector spaces over the reals, but the constructions may be extended to vector spaces over any field, allowing considering geometry over arbitrary fields, includingfinite fields.
Presently, most textbooks introduce geometric spaces from linear algebra, and geometry is often presented, at elementary level, as a subfield of linear algebra.
Usage and applications
[edit]Linear algebra is used in almost all areas of mathematics, thus making it relevant in almost all scientific domains that use mathematics. These applications may be divided into several wide categories.
Functional analysis
[edit]Functional analysisstudiesfunction spaces.These are vector spaces with additional structure, such asHilbert spaces.Linear algebra is thus a fundamental part of functional analysis and its applications, which include, in particular,quantum mechanics(wave functions) andFourier analysis(orthogonal basis).
Scientific computation
[edit]Nearly allscientific computationsinvolve linear algebra. Consequently, linear algebra algorithms have been highly optimized.BLASandLAPACKare the best known implementations. For improving efficiency, some of them configure the algorithms automatically, at run time, for adapting them to the specificities of the computer (cachesize, number of availablecores,...).
Someprocessors,typicallygraphics processing units(GPU), are designed with a matrix structure, for optimizing the operations of linear algebra.[citation needed]
Geometry of ambient space
[edit]Themodelingofambient spaceis based ongeometry.Sciences concerned with this space use geometry widely. This is the case withmechanicsandrobotics,for describingrigid body dynamics;geodesyfor describingEarth shape;perspectivity,computer vision,andcomputer graphics,for describing the relationship between a scene and its plane representation; and many other scientific domains.
In all these applications,synthetic geometryis often used for general descriptions and a qualitative approach, but for the study of explicit situations, one must compute withcoordinates.This requires the heavy use of linear algebra.
Study of complex systems
[edit]Most physical phenomena are modeled bypartial differential equations.To solve them, one usually decomposes the space in which the solutions are searched into small, mutually interactingcells.Forlinear systemsthis interaction involveslinear functions.Fornonlinear systems,this interaction is often approximated by linear functions.[b]This is called a linear model or first-order approximation. Linear models are frequently used for complex nonlinear real-world systems because it makesparametrizationmore manageable.[23]In both cases, very large matrices are generally involved.Weather forecasting(or more specifically,parametrization for atmospheric modeling) is a typical example of a real-world application, where the whole Earthatmosphereis divided into cells of, say, 100 km of width and 100 km of height.
Fluid Mechanics, Fluid Dynamics, and Thermal Energy Systems
[edit]Linear algebra, a branch of mathematics dealing withvector spacesandlinear mappingsbetween these spaces, plays a critical role in various engineering disciplines, includingfluid mechanics,fluid dynamics,andthermal energysystems. Its application in these fields is multifaceted and indispensable for solving complex problems.
Influid mechanics,linear algebra is integral to understanding and solving problems related to the behavior of fluids. It assists in the modeling and simulation of fluid flow, providing essential tools for the analysis offluid dynamicsproblems. For instance, linear algebraic techniques are used to solve systems ofdifferential equationsthat describe fluid motion. These equations, often complex andnon-linear,can be linearized using linear algebra methods, allowing for simpler solutions and analyses.
In the field of fluid dynamics, linear algebra finds its application incomputational fluid dynamics(CFD), a branch that usesnumerical analysisanddata structuresto solve and analyze problems involving fluid flows. CFD relies heavily on linear algebra for the computation of fluid flow andheat transferin various applications. For example, theNavier-Stokes equations,fundamental influid dynamics,are often solved using techniques derived from linear algebra. This includes the use ofmatricesandvectorsto represent and manipulate fluid flow fields.
Furthermore, linear algebra plays a crucial role inthermal energysystems, particularly inpower systemsanalysis. It is used to model and optimize the generation,transmission,anddistributionof electric power. Linear algebraic concepts such as matrix operations andeigenvalueproblems are employed to enhance the efficiency, reliability, and economic performance ofpower systems.The application of linear algebra in this context is vital for the design and operation of modernpower systems,includingrenewable energysources andsmart grids.
Overall, the application of linear algebra influid mechanics,fluid dynamics,andthermal energysystems is an example of the profound interconnection betweenmathematicsandengineering.It provides engineers with the necessary tools to model, analyze, and solve complex problems in these domains, leading to advancements in technology and industry.
Extensions and generalizations
[edit]This section presents several related topics that do not appear generally in elementary textbooks on linear algebra, but are commonly considered, in advanced mathematics, as parts of linear algebra.
Module theory
[edit]The existence of multiplicative inverses in fields is not involved in the axioms defining a vector space. One may thus replace the field of scalars by aringR,and this gives the structure called amoduleoverR,orR-module.
The concepts of linear independence, span, basis, and linear maps (also calledmodule homomorphisms) are defined for modules exactly as for vector spaces, with the essential difference that, ifRis not a field, there are modules that do not have any basis. The modules that have a basis are thefree modules,and those that are spanned by a finite set are thefinitely generated modules.Module homomorphisms between finitely generated free modules may be represented by matrices. The theory of matrices over a ring is similar to that of matrices over a field, except thatdeterminantsexist only if the ring iscommutative,and that a square matrix over a commutative ring isinvertibleonly if its determinant has amultiplicative inversein the ring.
Vector spaces are completely characterized by their dimension (up to an isomorphism). In general, there is not such a complete classification for modules, even if one restricts oneself to finitely generated modules. However, every module is acokernelof a homomorphism of free modules.
Modules over the integers can be identified withabelian groups,since the multiplication by an integer may be identified to a repeated addition. Most of the theory of abelian groups may be extended to modules over aprincipal ideal domain.In particular, over a principal ideal domain, every submodule of a free module is free, and thefundamental theorem of finitely generated abelian groupsmay be extended straightforwardly to finitely generated modules over a principal ring.
There are many rings for which there are algorithms for solving linear equations and systems of linear equations. However, these algorithms have generally acomputational complexitythat is much higher than the similar algorithms over a field. For more details, seeLinear equation over a ring.
Multilinear algebra and tensors
[edit]![]() | This section mayrequirecleanupto meet Wikipedia'squality standards.The specific problem is:The dual space is considered above, and the section must be rewritten to give an understandable summary of this subject.(September 2018) |
Inmultilinear algebra,one considers multivariable linear transformations, that is, mappings that are linear in each of a number of different variables. This line of inquiry naturally leads to the idea of thedual space,the vector spaceV*consisting of linear mapsf:V→FwhereFis the field of scalars. Multilinear mapsT:Vn→Fcan be described viatensor productsof elements ofV*.
If, in addition to vector addition and scalar multiplication, there is a bilinear vector productV×V→V,the vector space is called analgebra;for instance, associative algebras are algebras with an associate vector product (like the algebra of square matrices, or the algebra of polynomials).
Topological vector spaces
[edit]Vector spaces that are not finite dimensional often require additional structure to be tractable. Anormed vector spaceis a vector space along with a function called anorm,which measures the "size" of elements. The norm induces ametric,which measures the distance between elements, and induces atopology,which allows for a definition of continuous maps. The metric also allows for a definition oflimitsandcompleteness– a normed vector space that is complete is known as aBanach space.A complete metric space along with the additional structure of aninner product(a conjugate symmetricsesquilinear form) is known as aHilbert space,which is in some sense a particularly well-behaved Banach space.Functional analysisapplies the methods of linear algebra alongside those ofmathematical analysisto study various function spaces; the central objects of study in functional analysis areLpspaces,which are Banach spaces, and especially theL2space of square integrable functions, which is the only Hilbert space among them. Functional analysis is of particular importance to quantum mechanics, the theory of partial differential equations, digital signal processing, and electrical engineering. It also provides the foundation and theoretical framework that underlies the Fourier transform and related methods.
See also
[edit]- Fundamental matrix (computer vision)
- Geometric algebra
- Linear programming
- Linear regression,a statistical estimation method
- Numerical linear algebra
- Outline of linear algebra
- Transformation matrix
Explanatory notes
[edit]Citations
[edit]- ^Banerjee, Sudipto; Roy, Anindya (2014).Linear Algebra and Matrix Analysis for Statistics.Texts in Statistical Science (1st ed.). Chapman and Hall/CRC.ISBN978-1420095388.
- ^Strang, Gilbert (July 19, 2005).Linear Algebra and Its Applications(4th ed.). Brooks Cole.ISBN978-0-03-010567-8.
- ^Weisstein, Eric."Linear Algebra".MathWorld.Wolfram.Retrieved16 April2012.
- ^Hart, Roger (2010).The Chinese Roots of Linear Algebra.JHU Press.ISBN9780801899584.
- ^abcdVitulli, Marie."A Brief History of Linear Algebra and Matrix Theory".Department of Mathematics.University of Oregon. Archived fromthe originalon 2012-09-10.Retrieved2014-07-08.
- ^Koecher, M., Remmert, R. (1991). Hamilton’s Quaternions. In: Numbers. Graduate Texts in Mathematics, vol 123. Springer, New York, NY.https://doi.org/10.1007/978-1-4612-1005-4_10
- ^Benjamin Peirce(1872)Linear Associative Algebra,lithograph, new edition with corrections, notes, and an added 1875 paper by Peirce, plus notes by his sonCharles Sanders Peirce,published in theAmerican Journal of Mathematicsv. 4, 1881, Johns Hopkins University, pp. 221–226,GoogleEprintand as an extract, D. Van Nostrand, 1882,GoogleEprint.
- ^Roman (2005,ch. 1, p. 27)
- ^Axler (2015)p. 82, §3.59
- ^Axler (2015)p. 23, §1.45
- ^Anton (1987,p. 2)
- ^Beauregard & Fraleigh (1973,p. 65)
- ^Burden & Faires (1993,p. 324)
- ^Golub & Van Loan (1996,p. 87)
- ^Harper (1976,p. 57)
- ^Katznelson & Katznelson (2008)pp. 76–77, § 4.4.1–4.4.6
- ^Katznelson & Katznelson (2008)p. 37 §2.1.3
- ^Halmos (1974)p. 20, §13
- ^Axler (2015)p. 101, §3.94
- ^P. K. Jain, Khalil Ahmad (1995)."5.1 Definitions and basic properties of inner product spaces and Hilbert spaces".Functional analysis(2nd ed.). New Age International. p. 203.ISBN81-224-0801-X.
- ^Eduard Prugovec̆ki (1981)."Definition 2.1".Quantum mechanics in Hilbert space(2nd ed.). Academic Press. pp. 18ff.ISBN0-12-566060-X.
- ^Emil Artin(1957)Geometric AlgebraInterscience Publishers
- ^Savov, Ivan (2017).No Bullshit Guide to Linear Algebra.MinireferenceCo. pp. 150–155.ISBN9780992001025.
- ^"MIT OpenCourseWare. Special Topics in Mathematics with Applications: Linear Algebra and the Calculus of Variations - Mechanical Engineering".
- ^"FAMU-FSU College of Engineering. ME Undergraduate Curriculum".
- ^"University of Colorado Denver. Energy and Power Systems".
General and cited sources
[edit]- Anton, Howard (1987),Elementary Linear Algebra(5th ed.), New York:Wiley,ISBN0-471-84819-0
- Axler, Sheldon(18 December 2014),Linear Algebra Done Right,Undergraduate Texts in Mathematics(3rd ed.),Springer Publishing(published 2015),ISBN978-3-319-11079-0,MR3308468
- Beauregard, Raymond A.; Fraleigh, John B. (1973),A First Course In Linear Algebra: with Optional Introduction to Groups, Rings, and Fields,Boston:Houghton Mifflin Company,ISBN0-395-14017-X
- Burden, Richard L.; Faires, J. Douglas (1993),Numerical Analysis(5th ed.), Boston:Prindle, Weber and Schmidt,ISBN0-534-93219-3
- Golub, Gene H.;Van Loan, Charles F.(1996),Matrix Computations,Johns Hopkins Studies in Mathematical Sciences (3rd ed.), Baltimore:Johns Hopkins University Press,ISBN978-0-8018-5414-9
- Halmos, Paul Richard(1974),Finite-Dimensional Vector Spaces,Undergraduate Texts in Mathematics(1958 2nd ed.),Springer Publishing,ISBN0-387-90093-4,OCLC1251216
- Harper, Charlie (1976),Introduction to Mathematical Physics,New Jersey:Prentice-Hall,ISBN0-13-487538-9
- Katznelson, Yitzhak;Katznelson, Yonatan R. (2008),A (Terse) Introduction to Linear Algebra,American Mathematical Society,ISBN978-0-8218-4419-9
- Roman, Steven(March 22, 2005),Advanced Linear Algebra,Graduate Texts in Mathematics(2nd ed.), Springer,ISBN978-0-387-24766-3
Further reading
[edit]History
[edit]- Fearnley-Sander, Desmond, "Hermann Grassmann and the Creation of Linear Algebra",American Mathematical Monthly86(1979), pp. 809–817.
- Grassmann, Hermann(1844),Die lineale Ausdehnungslehre ein neuer Zweig der Mathematik: dargestellt und durch Anwendungen auf die übrigen Zweige der Mathematik, wie auch auf die Statik, Mechanik, die Lehre vom Magnetismus und die Krystallonomie erläutert,Leipzig: O. Wigand
Introductory textbooks
[edit]- Anton, Howard (2005),Elementary Linear Algebra (Applications Version)(9th ed.), Wiley International
- Banerjee, Sudipto; Roy, Anindya (2014),Linear Algebra and Matrix Analysis for Statistics,Texts in Statistical Science (1st ed.), Chapman and Hall/CRC,ISBN978-1420095388
- Bretscher, Otto (2004),Linear Algebra with Applications(3rd ed.), Prentice Hall,ISBN978-0-13-145334-0
- Farin, Gerald;Hansford, Dianne(2004),Practical Linear Algebra: A Geometry Toolbox,AK Peters,ISBN978-1-56881-234-2
- Hefferon, Jim(2020).Linear Algebra(4th ed.).Ann Arbor, Michigan:Orthogonal Publishing.ISBN978-1-944325-11-4.OCLC1178900366.OL30872051M.
- Kolman, Bernard; Hill, David R. (2007),Elementary Linear Algebra with Applications(9th ed.), Prentice Hall,ISBN978-0-13-229654-0
- Lay, David C. (2005),Linear Algebra and Its Applications(3rd ed.), Addison Wesley,ISBN978-0-321-28713-7
- Leon, Steven J. (2006),Linear Algebra With Applications(7th ed.), Pearson Prentice Hall,ISBN978-0-13-185785-8
- Murty, Katta G. (2014)Computational and Algorithmic Linear Algebra and n-Dimensional Geometry,World Scientific Publishing,ISBN978-981-4366-62-5.Chapter 1: Systems of Simultaneous Linear Equations
- Noble, B. & Daniel, J.W. (2nd Ed. 1977)[1],Pearson Higher Education,ISBN978-0130413437.
- Poole, David (2010),Linear Algebra: A Modern Introduction(3rd ed.), Cengage – Brooks/Cole,ISBN978-0-538-73545-2
- Ricardo, Henry (2010),A Modern Introduction To Linear Algebra(1st ed.), CRC Press,ISBN978-1-4398-0040-9
- Sadun, Lorenzo (2008),Applied Linear Algebra: the decoupling principle(2nd ed.), AMS,ISBN978-0-8218-4441-0
- Strang, Gilbert(2016),Introduction to Linear Algebra(5th ed.), Wellesley-Cambridge Press,ISBN978-09802327-7-6
- The Manga Guide to Linear Algebra (2012), byShin Takahashi,Iroha Inoue and Trend-Pro Co., Ltd.,ISBN978-1-59327-413-9
Advanced textbooks
[edit]- Bhatia, Rajendra (November 15, 1996),Matrix Analysis,Graduate Texts in Mathematics,Springer,ISBN978-0-387-94846-1
- Demmel, James W.(August 1, 1997),Applied Numerical Linear Algebra,SIAM,ISBN978-0-89871-389-3
- Dym, Harry(2007),Linear Algebra in Action,AMS,ISBN978-0-8218-3813-6
- Gantmacher, Felix R.(2005),Applications of the Theory of Matrices,Dover Publications,ISBN978-0-486-44554-0
- Gantmacher, Felix R. (1990),Matrix Theory Vol. 1(2nd ed.), American Mathematical Society,ISBN978-0-8218-1376-8
- Gantmacher, Felix R. (2000),Matrix Theory Vol. 2(2nd ed.), American Mathematical Society,ISBN978-0-8218-2664-5
- Gelfand, Israel M.(1989),Lectures on Linear Algebra,Dover Publications,ISBN978-0-486-66082-0
- Glazman, I. M.; Ljubic, Ju. I. (2006),Finite-Dimensional Linear Analysis,Dover Publications,ISBN978-0-486-45332-3
- Golan, Johnathan S. (January 2007),The Linear Algebra a Beginning Graduate Student Ought to Know(2nd ed.), Springer,ISBN978-1-4020-5494-5
- Golan, Johnathan S. (August 1995),Foundations of Linear Algebra,Kluwer,ISBN0-7923-3614-3
- Greub, Werner H. (October 16, 1981),Linear Algebra,Graduate Texts in Mathematics (4th ed.), Springer,ISBN978-0-8018-5414-9
- Hoffman, Kenneth;Kunze, Ray(1971),Linear algebra(2nd ed.), Englewood Cliffs, N.J.: Prentice-Hall, Inc.,MR0276251
- Halmos, Paul R.(August 20, 1993),Finite-Dimensional Vector Spaces,Undergraduate Texts in Mathematics,Springer,ISBN978-0-387-90093-3
- Friedberg, Stephen H.; Insel, Arnold J.; Spence, Lawrence E. (September 7, 2018),Linear Algebra(5th ed.), Pearson,ISBN978-0-13-486024-4
- Horn, Roger A.;Johnson, Charles R.(February 23, 1990),Matrix Analysis,Cambridge University Press,ISBN978-0-521-38632-6
- Horn, Roger A.; Johnson, Charles R. (June 24, 1994),Topics in Matrix Analysis,Cambridge University Press,ISBN978-0-521-46713-1
- Lang, Serge(March 9, 2004),Linear Algebra,Undergraduate Texts in Mathematics (3rd ed.), Springer,ISBN978-0-387-96412-6
- Marcus, Marvin;Minc, Henryk(2010),A Survey of Matrix Theory and Matrix Inequalities,Dover Publications,ISBN978-0-486-67102-4
- Meyer, Carl D. (February 15, 2001),Matrix Analysis and Applied Linear Algebra,Society for Industrial and Applied Mathematics (SIAM),ISBN978-0-89871-454-8,archived fromthe originalon October 31, 2009
- Mirsky, L.(1990),An Introduction to Linear Algebra,Dover Publications,ISBN978-0-486-66434-7
- Shafarevich, I. R.;Remizov, A. O (2012),Linear Algebra and Geometry,Springer,ISBN978-3-642-30993-9
- Shilov, Georgi E.(June 1, 1977),Linear algebra,Dover Publications,ISBN978-0-486-63518-7
- Shores, Thomas S. (December 6, 2006),Applied Linear Algebra and Matrix Analysis,Undergraduate Texts in Mathematics, Springer,ISBN978-0-387-33194-2
- Smith, Larry (May 28, 1998),Linear Algebra,Undergraduate Texts in Mathematics, Springer,ISBN978-0-387-98455-1
- Trefethen, Lloyd N.;Bau, David (1997),Numerical Linear Algebra,SIAM,ISBN978-0-898-71361-9
Study guides and outlines
[edit]- Leduc, Steven A. (May 1, 1996),Linear Algebra (Cliffs Quick Review),Cliffs Notes,ISBN978-0-8220-5331-6
- Lipschutz, Seymour; Lipson, Marc (December 6, 2000),Schaum's Outline of Linear Algebra(3rd ed.), McGraw-Hill,ISBN978-0-07-136200-9
- Lipschutz, Seymour (January 1, 1989),3,000 Solved Problems in Linear Algebra,McGraw–Hill,ISBN978-0-07-038023-3
- McMahon, David (October 28, 2005),Linear Algebra Demystified,McGraw–Hill Professional,ISBN978-0-07-146579-3
- Zhang, Fuzhen (April 7, 2009),Linear Algebra: Challenging Problems for Students,The Johns Hopkins University Press,ISBN978-0-8018-9125-0
External links
[edit]
Online Resources
[edit]
- MIT Linear Algebra Video Lectures,a series of 34 recorded lectures by ProfessorGilbert Strang(Spring 2010)
- International Linear Algebra Society
- "Linear algebra",Encyclopedia of Mathematics,EMS Press,2001 [1994]
- Linear AlgebraonMathWorld
- Matrix and Linear Algebra TermsonEarliest Known Uses of Some of the Words of Mathematics
- Earliest Uses of Symbols for Matrices and VectorsonEarliest Uses of Various Mathematical Symbols
- Essence of linear algebra,a video presentation from3Blue1Brownof the basics of linear algebra, with emphasis on the relationship between the geometric, the matrix and the abstract points of view
Online books
[edit]- Beezer, Robert A. (2009) [2004].A First Course in Linear Algebra.Gainesville, Florida:University Press of Florida.ISBN9781616100049.
- Connell, Edwin H. (2004) [1999].Elements of Abstract and Linear Algebra.University of Miami,Coral Gables, Florida:Self-published.
- Hefferon, Jim(2020).Linear Algebra(4th ed.).Ann Arbor, Michigan:Orthogonal Publishing.ISBN978-1-944325-11-4.OCLC1178900366.OL30872051M.
- Margalit, Dan;Rabinoff, Joseph (2019).Interactive Linear Algebra.Georgia Institute of Technology,Atlanta, Georgia:Self-published.
- Matthews, Keith R. (2013) [1991].Elementary Linear Algebra.University of Queensland,Brisbane, Australia:Self-published.
- Mikaelian, Vahagn H. (2020) [2017].Linear Algebra: Theory and Algorithms.Yerevan, Armenia:Self-published – viaResearchGate.
- Sharipov, Ruslan,Course of linear algebra and multidimensional geometry
- Treil, Sergei,Linear Algebra Done Wrong