Speech synthesis
Speech synthesisis the artificial production of humanspeech.A computer system used for this purpose is called aspeech synthesizer,and can be implemented insoftwareorhardwareproducts. Atext-to-speech(TTS) system converts normal language text into speech; other systems rendersymbolic linguistic representationslikephonetic transcriptionsinto speech.[1]The reverse process isspeech recognition.
Synthesized speech can be created byconcatenatingpieces of recorded speech that are stored in adatabase.Systems differ in the size of the stored speech units; a system that storesphonesordiphonesprovides the largest output range, but may lack clarity. For specific usage domains, the storage of entire words or sentences allows for high-quality output. Alternatively, a synthesizer can incorporate a model of thevocal tractand other human voice characteristics to create a completely "synthetic" voice output.[2]
The quality of a speech synthesizer is judged by its similarity to the human voice and by its ability to be understood clearly. An intelligible text-to-speech program allows people withvisual impairmentsorreading disabilitiesto listen to written words on a home computer. Many computeroperating systemshave included speech synthesizers since the early 1990s.

A text-to-speech system (or "engine" ) is composed of two parts:[3]afront-endand aback-end.The front-end has two major tasks. First, it converts raw text containing symbols like numbers and abbreviations into the equivalent of written-out words. This process is often calledtext normalization,pre-processing,ortokenization.The front-end then assignsphonetic transcriptionsto each word, and divides and marks the text intoprosodic units,likephrases,clauses,andsentences.The process of assigning phonetic transcriptions to words is calledtext-to-phonemeorgrapheme-to-phonemeconversion. Phonetic transcriptions andprosodyinformation together make up the symbolic linguistic representation that is output by the front-end. The back-end—often referred to as thesynthesizer—then converts the symbolic linguistic representation into sound. In certain systems, this part includes the computation of thetarget prosody(pitch contour, phoneme durations),[4]which is then imposed on the output speech.
History
[edit]Long before the invention ofelectronicsignal processing,some people tried to build machines to emulate human speech. Some early legends of the existence of "Brazen Heads"involved PopeSilvester II(d. 1003 AD),Albertus Magnus(1198–1280), andRoger Bacon(1214–1294).
In 1779, theGerman-DanishscientistChristian Gottlieb Kratzensteinwon the first prize in a competition announced by the RussianImperial Academy of Sciences and Artsfor models he built of the humanvocal tractthat could produce the five longvowelsounds (inInternational Phonetic Alphabetnotation:[aː],[eː],[iː],[oː]and[uː]).[5]There followed thebellows-operated "acoustic-mechanical speech machine"ofWolfgang von KempelenofPressburg,Hungary, described in a 1791 paper.[6]This machine added models of the tongue and lips, enabling it to produce consonants as well as vowels. In 1837,Charles Wheatstoneproduced a "speaking machine" based on von Kempelen's design, and in 1846, Joseph Faber exhibited the "Euphonia".In 1923, Paget resurrected Wheatstone's design.[7]
In the 1930s,Bell Labsdeveloped thevocoder,which automatically analyzed speech into its fundamental tones and resonances. From his work on the vocoder,Homer Dudleydeveloped a keyboard-operated voice-synthesizer calledThe Voder(Voice Demonstrator), which he exhibited at the1939 New York World's Fair.
Dr. Franklin S. Cooperand his colleagues atHaskins Laboratoriesbuilt thePattern playbackin the late 1940s and completed it in 1950. There were several different versions of this hardware device; only one currently survives. The machine converts pictures of the acoustic patterns of speech in the form of a spectrogram back into sound. Using this device,Alvin Libermanand colleagues discovered acoustic cues for the perception ofphoneticsegments (consonants and vowels).
Electronic devices
[edit]
The first computer-based speech-synthesis systems originated in the late 1950s. Noriko Umedaet al.developed the first general English text-to-speech system in 1968, at theElectrotechnical Laboratoryin Japan.[8]In 1961, physicistJohn Larry Kelly, Jrand his colleagueLouis Gerstman[9]used anIBM 704computer to synthesize speech, an event among the most prominent in the history ofBell Labs.[citation needed]Kelly's voice recorder synthesizer (vocoder) recreated the song "Daisy Bell",with musical accompaniment fromMax Mathews.Coincidentally,Arthur C. Clarkewas visiting his friend and colleague John Pierce at the Bell Labs Murray Hill facility. Clarke was so impressed by the demonstration that he used it in the climactic scene of his screenplay for his novel2001: A Space Odyssey,[10]where theHAL 9000computer sings the same song as astronautDave Bowmanputs it to sleep.[11]Despite the success of purely electronic speech synthesis, research into mechanical speech-synthesizers continues.[12][third-party source needed]
Linear predictive coding(LPC), a form ofspeech coding,began development with the work ofFumitada ItakuraofNagoya Universityand Shuzo Saito ofNippon Telegraph and Telephone(NTT) in 1966. Further developments in LPC technology were made byBishnu S. AtalandManfred R. SchroederatBell Labsduring the 1970s.[13]LPC was later the basis for early speech synthesizer chips, such as theTexas Instruments LPC Speech Chipsused in theSpeak & Spelltoys from 1978.
In 1975, Fumitada Itakura developed theline spectral pairs(LSP) method for high-compression speech coding, while at NTT.[14][15][16]From 1975 to 1981, Itakura studied problems in speech analysis and synthesis based on the LSP method.[16]In 1980, his team developed an LSP-based speech synthesizer chip. LSP is an important technology for speech synthesis and coding, and in the 1990s was adopted by almost all international speech coding standards as an essential component, contributing to the enhancement of digital speech communication over mobile channels and the internet.[15]
In 1975,MUSAwas released, and was one of the first Speech Synthesis systems. It consisted of a stand-alone computer hardware and a specialized software that enabled it to read Italian. A second version, released in 1978, was also able to sing Italian in an "a cappella"style.[17]
Dominant systems in the 1980s and 1990s were theDECtalksystem, based largely on the work ofDennis Klattat MIT, and the Bell Labs system;[18]the latter was one of the first multilingual language-independent systems, making extensive use ofnatural language processingmethods.
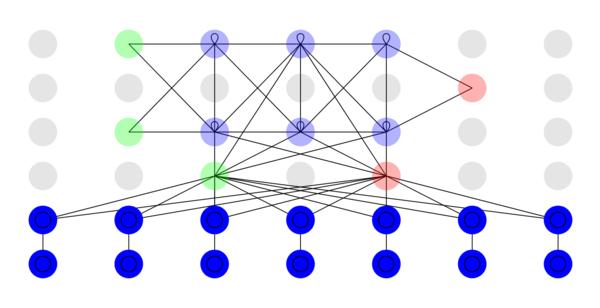

Handheldelectronics featuring speech synthesis began emerging in the 1970s. One of the first was theTelesensory Systems Inc.(TSI)Speech+portable calculator for the blind in 1976.[19][20]Other devices had primarily educational purposes, such as theSpeak & Spell toyproduced byTexas Instrumentsin 1978.[21]Fidelity released a speaking version of its electronic chess computer in 1979.[22]The firstvideo gameto feature speech synthesis was the 1980shoot 'em uparcade game,Stratovox(known in Japan asSpeak & Rescue), fromSun Electronics.[23][24]The firstpersonal computer gamewith speech synthesis wasManbiki Shoujo(Shoplifting Girl), released in 1980 for thePET 2001,for which the game's developer, Hiroshi Suzuki, developed a "zero cross"programming technique to produce a synthesized speech waveform.[25]Another early example, the arcade version ofBerzerk,also dates from 1980. TheMilton Bradley Companyproduced the first multi-playerelectronic gameusing voice synthesis,Milton,in the same year.
In 1976, Computalker Consultants released their CT-1 Speech Synthesizer. Designed by D. Lloyd Rice and Jim Cooper, it was an analog synthesizer built to work with microcomputers using the S-100 bus standard.[26]
Early electronic speech-synthesizers sounded robotic and were often barely intelligible. The quality of synthesized speech has steadily improved, but as of 2016[update]output from contemporary speech synthesis systems remains clearly distinguishable from actual human speech.
Synthesized voices typically sounded male until 1990, whenAnn Syrdal,atAT&T Bell Laboratories,created a female voice.[27]
Kurzweil predicted in 2005 that as thecost-performance ratiocaused speech synthesizers to become cheaper and more accessible, more people would benefit from the use of text-to-speech programs.[28]
Synthesizer technologies
[edit]The most important qualities of a speech synthesis system arenaturalnessandintelligibility.[29]Naturalness describes how closely the output sounds like human speech, while intelligibility is the ease with which the output is understood. The ideal speech synthesizer is both natural and intelligible. Speech synthesis systems usually try to maximize both characteristics.
The two primary technologies generating synthetic speech waveforms areconcatenative synthesisandformantsynthesis.Each technology has strengths and weaknesses, and the intended uses of a synthesis system will typically determine which approach is used.
Concatenation synthesis
[edit]Concatenative synthesis is based on the concatenation (stringing together) of segments of recorded speech. Generally, concatenative synthesis produces the most natural-sounding synthesized speech. However, differences between natural variations in speech and the nature of the automated techniques for segmenting the waveforms sometimes result in audible glitches in the output. There are three main sub-types of concatenative synthesis.
Unit selection synthesis
[edit]Unit selection synthesis uses large databases of recorded speech. During database creation, each recorded utterance is segmented into some or all of the following: individualphones,diphones,half-phones,syllables,morphemes,words,phrases,andsentences.Typically, the division into segments is done using a specially modifiedspeech recognizerset to a "forced alignment" mode with some manual correction afterward, using visual representations such as thewaveformandspectrogram.[30]Anindexof the units in the speech database is then created based on the segmentation and acoustic parameters like thefundamental frequency(pitch), duration, position in the syllable, and neighboring phones. Atrun time,the desired target utterance is created by determining the best chain of candidate units from the database (unit selection). This process is typically achieved using a specially weighteddecision tree.
Unit selection provides the greatest naturalness, because it applies only a small amount ofdigital signal processing(DSP) to the recorded speech. DSP often makes recorded speech sound less natural, although some systems use a small amount of signal processing at the point of concatenation to smooth the waveform. The output from the best unit-selection systems is often indistinguishable from real human voices, especially in contexts for which the TTS system has been tuned. However, maximum naturalness typically require unit-selection speech databases to be very large, in some systems ranging into thegigabytesof recorded data, representing dozens of hours of speech.[31]Also, unit selection algorithms have been known to select segments from a place that results in less than ideal synthesis (e.g. minor words become unclear) even when a better choice exists in the database.[32]Recently, researchers have proposed various automated methods to detect unnatural segments in unit-selection speech synthesis systems.[33]
Diphone synthesis
[edit]Diphone synthesis uses a minimal speech database containing all thediphones(sound-to-sound transitions) occurring in a language. The number of diphones depends on thephonotacticsof the language: for example, Spanish has about 800 diphones, and German about 2500. In diphone synthesis, only one example of each diphone is contained in the speech database. At runtime, the targetprosodyof a sentence is superimposed on these minimal units by means ofdigital signal processingtechniques such aslinear predictive coding,PSOLA[34]orMBROLA.[35]or more recent techniques such as pitch modification in the source domain usingdiscrete cosine transform.[36]Diphone synthesis suffers from the sonic glitches of concatenative synthesis and the robotic-sounding nature of formant synthesis, and has few of the advantages of either approach other than small size. As such, its use in commercial applications is declining,[citation needed]although it continues to be used in research because there are a number of freely available software implementations. An early example of Diphone synthesis is a teaching robot,Leachim,that was invented byMichael J. Freeman.[37]Leachim contained information regarding class curricular and certain biographical information about the students whom it was programmed to teach.[38]It was tested in a fourth grade classroom inthe Bronx, New York.[39][40]
Domain-specific synthesis
[edit]Domain-specific synthesis concatenates prerecorded words and phrases to create complete utterances. It is used in applications where the variety of texts the system will output is limited to a particular domain, like transit schedule announcements or weather reports.[41]The technology is very simple to implement, and has been in commercial use for a long time, in devices like talking clocks and calculators. The level of naturalness of these systems can be very high because the variety of sentence types is limited, and they closely match the prosody and intonation of the original recordings.[citation needed]
Because these systems are limited by the words and phrases in their databases, they are not general-purpose and can only synthesize the combinations of words and phrases with which they have been preprogrammed. The blending of words within naturally spoken language however can still cause problems unless the many variations are taken into account. For example, innon-rhoticdialects of English the"r"in words like"clear"/ˈklɪə/is usually only pronounced when the following word has a vowel as its first letter (e.g."clear out"is realized as/ˌklɪəɹˈʌʊt/). Likewise inFrench,many final consonants become no longer silent if followed by a word that begins with a vowel, an effect calledliaison.Thisalternationcannot be reproduced by a simple word-concatenation system, which would require additional complexity to becontext-sensitive.
Formant synthesis
[edit]Formantsynthesis does not use human speech samples at runtime. Instead, the synthesized speech output is created usingadditive synthesisand an acoustic model (physical modelling synthesis).[42]Parameters such asfundamental frequency,voicing,andnoiselevels are varied over time to create awaveformof artificial speech. This method is sometimes calledrules-based synthesis;however, many concatenative systems also have rules-based components. Many systems based on formant synthesis technology generate artificial, robotic-sounding speech that would never be mistaken for human speech. However, maximum naturalness is not always the goal of a speech synthesis system, and formant synthesis systems have advantages over concatenative systems. Formant-synthesized speech can be reliably intelligible, even at very high speeds, avoiding the acoustic glitches that commonly plague concatenative systems. High-speed synthesized speech is used by the visually impaired to quickly navigate computers using ascreen reader.Formant synthesizers are usually smaller programs than concatenative systems because they do not have a database of speech samples. They can therefore be used inembedded systems,wherememoryandmicroprocessorpower are especially limited. Because formant-based systems have complete control of all aspects of the output speech, a wide variety of prosodies andintonationscan be output, conveying not just questions and statements, but a variety of emotions and tones of voice.
Examples of non-real-time but highly accurate intonation control in formant synthesis include the work done in the late 1970s for theTexas InstrumentstoySpeak & Spell,and in the early 1980sSegaarcademachines[43]and in manyAtari, Inc.arcade games[44]using theTMS5220 LPC Chips.Creating proper intonation for these projects was painstaking, and the results have yet to be matched by real-time text-to-speech interfaces.[45]
Articulatory synthesis
[edit]Articulatory synthesis consists of computational techniques for synthesizing speech based on models of the humanvocal tractand the articulation processes occurring there. The first articulatory synthesizer regularly used for laboratory experiments was developed atHaskins Laboratoriesin the mid-1970s byPhilip Rubin,Tom Baer, and Paul Mermelstein. This synthesizer, known as ASY, was based on vocal tract models developed atBell Laboratoriesin the 1960s and 1970s by Paul Mermelstein, Cecil Coker, and colleagues.
Until recently, articulatory synthesis models have not been incorporated into commercial speech synthesis systems. A notable exception is theNeXT-based system originally developed and marketed by Trillium Sound Research, a spin-off company of theUniversity of Calgary,where much of the original research was conducted. Following the demise of the various incarnations of NeXT (started bySteve Jobsin the late 1980s and merged with Apple Computer in 1997), the Trillium software was published under the GNU General Public License, with work continuing asgnuspeech.The system, first marketed in 1994, provides full articulatory-based text-to-speech conversion using a waveguide or transmission-line analog of the human oral and nasal tracts controlled by Carré's "distinctive region model".
More recent synthesizers, developed by Jorge C. Lucero and colleagues, incorporate models of vocal fold biomechanics, glottal aerodynamics and acoustic wave propagation in the bronchi, trachea, nasal and oral cavities, and thus constitute full systems of physics-based speech simulation.[46][47]
HMM-based synthesis
[edit]HMM-based synthesis is a synthesis method based onhidden Markov models,also called Statistical Parametric Synthesis. In this system, thefrequency spectrum(vocal tract),fundamental frequency(voice source), and duration (prosody) of speech are modeled simultaneously by HMMs. Speechwaveformsare generated from HMMs themselves based on themaximum likelihoodcriterion.[48]
Sinewave synthesis
[edit]Sinewave synthesis is a technique for synthesizing speech by replacing theformants(main bands of energy) with pure tone whistles.[49]
Deep learning-based synthesis
[edit]Deep learning speech synthesis usesdeep neural networks(DNN) to produce artificial speech from text (text-to-speech) or spectrum (vocoder). The deep neural networks are trained using a large amount of recorded speech and, in the case of a text-to-speech system, the associated labels and/or input text.
15.aiuses amulti-speaker model—hundreds of voices are trained concurrently rather than sequentially, decreasing the required training time and enabling the model to learn and generalize shared emotional context, even for voices with no exposure to such emotional context.[50]Thedeep learningmodel used by the application isnondeterministic:each time that speech is generated from the same string of text, the intonation of the speech will be slightly different. The application also supports manually altering theemotionof a generated line usingemotional contextualizers(a term coined by this project), a sentence or phrase that conveys the emotion of the take that serves as a guide for the model during inference.[51][52]
ElevenLabsis primarily known for itsbrowser-based,AI-assisted text-to-speech software, Speech Synthesis, which can produce lifelike speech by synthesizingvocal emotionandintonation.[53]The company states its software is built to adjust the intonation and pacing of delivery based on the context of language input used.[54]It uses advanced algorithms to analyze the contextual aspects of text, aiming to detect emotions like anger, sadness, happiness, or alarm, which enables the system to understand the user's sentiment,[55]resulting in a more realistic and human-like inflection. Other features include multilingual speech generation and long-form content creation with contextually-aware voices.[56][57]
The DNN-based speech synthesizers are approaching the naturalness of the human voice. Examples of disadvantages of the method are low robustness when the data are not sufficient, lack of controllability and low performance in auto-regressive models.
For tonal languages, such as Chinese or Taiwanese language, there are different levels of tone sandhi required and sometimes the output of speech synthesizer may result in the mistakes of tone sandhi.[58]
Audio deepfakes
[edit]In 2023,VICEreporter Joseph Cox published findings that he had recorded five minutes of himself talking and then used a tool developed by ElevenLabs to create voice deepfakes that defeated a bank'svoice-authenticationsystem.[66]
Challenges
[edit]Text normalization challenges
[edit]The process of normalizing text is rarely straightforward. Texts are full ofheteronyms,numbers,andabbreviationsthat all require expansion into a phonetic representation. There are many spellings in English which are pronounced differently based on context. For example, "My latest project is to learn how to better project my voice" contains two pronunciations of "project".
Most text-to-speech (TTS) systems do not generatesemanticrepresentations of their input texts, as processes for doing so are unreliable, poorly understood, and computationally ineffective. As a result, variousheuristictechniques are used to guess the proper way to disambiguatehomographs,like examining neighboring words and using statistics about frequency of occurrence.
Recently TTS systems have begun to use HMMs (discussedabove) to generate "parts of speech"to aid in disambiguating homographs. This technique is quite successful for many cases such as whether" read "should be pronounced as" red "implying past tense, or as" reed "implying present tense. Typical error rates when using HMMs in this fashion are usually below five percent. These techniques also work well for most European languages, although access to required trainingcorporais frequently difficult in these languages.
Deciding how to convert numbers is another problem that TTS systems have to address. It is a simple programming challenge to convert a number into words (at least in English), like "1325" becoming "one thousand three hundred twenty-five". However, numbers occur in many different contexts; "1325" may also be read as "one three two five", "thirteen twenty-five" or "thirteen hundred and twenty five". A TTS system can often infer how to expand a number based on surrounding words, numbers, and punctuation, and sometimes the system provides a way to specify the context if it is ambiguous.[67]Roman numerals can also be read differently depending on context. For example, "Henry VIII" reads as "Henry the Eighth", while "Chapter VIII" reads as "Chapter Eight".
Similarly, abbreviations can be ambiguous. For example, the abbreviation "in" for "inches" must be differentiated from the word "in", and the address "12 St John St." uses the same abbreviation for both "Saint" and "Street". TTS systems with intelligent front ends can make educated guesses about ambiguous abbreviations, while others provide the same result in all cases, resulting in nonsensical (and sometimes comical) outputs, such as "Ulysses S. Grant"being rendered as" Ulysses South Grant ".
Text-to-phoneme challenges
[edit]Speech synthesis systems use two basic approaches to determine the pronunciation of a word based on itsspelling,a process which is often called text-to-phoneme orgrapheme-to-phoneme conversion (phonemeis the term used bylinguiststo describe distinctive sounds in alanguage). The simplest approach to text-to-phoneme conversion is the dictionary-based approach, where a large dictionary containing all the words of a language and their correctpronunciationsis stored by the program. Determining the correct pronunciation of each word is a matter of looking up each word in the dictionary and replacing the spelling with the pronunciation specified in the dictionary. The other approach is rule-based, in which pronunciation rules are applied to words to determine their pronunciations based on their spellings. This is similar to the "sounding out", orsynthetic phonics,approach to learning reading.
Each approach has advantages and drawbacks. The dictionary-based approach is quick and accurate, but completely fails if it is given a word which is not in its dictionary. As dictionary size grows, so too does the memory space requirements of the synthesis system. On the other hand, the rule-based approach works on any input, but the complexity of the rules grows substantially as the system takes into account irregular spellings or pronunciations. (Consider that the word "of" is very common in English, yet is the only word in which the letter "f" is pronounced[v].) As a result, nearly all speech synthesis systems use a combination of these approaches.
Languages with aphonemic orthographyhave a very regular writing system, and the prediction of the pronunciation of words based on their spellings is quite successful. Speech synthesis systems for such languages often use the rule-based method extensively, resorting to dictionaries only for those few words, like foreign names and loanwords, whose pronunciations are not obvious from their spellings. On the other hand, speech synthesis systems for languages like English, which have extremely irregular spelling systems, are more likely to rely on dictionaries, and to use rule-based methods only for unusual words, or words that are not in their dictionaries.
Evaluation challenges
[edit]The consistent evaluation of speech synthesis systems may be difficult because of a lack of universally agreed objective evaluation criteria. Different organizations often use different speech data. The quality of speech synthesis systems also depends on the quality of the production technique (which may involve analogue or digital recording) and on the facilities used to replay the speech. Evaluating speech synthesis systems has therefore often been compromised by differences between production techniques and replay facilities.
Since 2005, however, some researchers have started to evaluate speech synthesis systems using a common speech dataset.[68]
Prosodics and emotional content
[edit]A study in the journalSpeech Communicationby Amy Drahota and colleagues at theUniversity of Portsmouth,UK,reported that listeners to voice recordings could determine, at better than chance levels, whether or not the speaker was smiling.[69][70][71]It was suggested that identification of the vocal features that signal emotional content may be used to help make synthesized speech sound more natural. One of the related issues is modification of thepitch contourof the sentence, depending upon whether it is an affirmative, interrogative or exclamatory sentence. One of the techniques for pitch modification[72]usesdiscrete cosine transformin the source domain (linear predictionresidual). Such pitch synchronous pitch modification techniques need a priori pitch marking of the synthesis speech database using techniques such as epoch extraction using dynamicplosionindex applied on the integrated linear prediction residual of thevoicedregions of speech.[73]
Dedicated hardware
[edit]
- Icophone
- General Instrument SP0256-AL2
- National SemiconductorDT1050 Digitalker (Mozer –Forrest Mozer)
- Texas Instruments LPC Speech Chips[74]
Hardware and software systems
[edit]Popular systems offering speech synthesis as a built-in capability.
Texas Instruments
[edit]In the early 1980s, TI was known as a pioneer in speech synthesis, and a highly popular plug-in speech synthesizer module was available for the TI-99/4 and 4A. Speech synthesizers were offered free with the purchase of a number of cartridges and were used by many TI-written video games (games offered with speech during this promotion includedAlpinerandParsec). The synthesizer uses a variant of linear predictive coding and has a small in-built vocabulary. The original intent was to release small cartridges that plugged directly into the synthesizer unit, which would increase the device's built-in vocabulary. However, the success of software text-to-speech in the Terminal Emulator II cartridge canceled that plan.
Mattel
[edit]TheMattelIntellivisiongame console offered theIntellivoiceVoice Synthesis module in 1982. It included theSP0256 Narratorspeech synthesizer chip on a removable cartridge. The Narrator had 2kB of Read-Only Memory (ROM), and this was utilized to store a database of generic words that could be combined to make phrases in Intellivision games. Since the Orator chip could also accept speech data from external memory, any additional words or phrases needed could be stored inside the cartridge itself. The data consisted of strings of analog-filter coefficients to modify the behavior of the chip's synthetic vocal-tract model, rather than simple digitized samples.
SAM
[edit]Also released in 1982,Software Automatic Mouthwas the first commercial all-software voice synthesis program. It was later used as the basis forMacintalk.The program was available for non-Macintosh Apple computers (including the Apple II, and the Lisa), various Atari models and the Commodore 64. The Apple version preferred additional hardware that contained DACs, although it could instead use the computer's one-bit audio output (with the addition of much distortion) if the card was not present. The Atari made use of the embedded POKEY audio chip. Speech playback on the Atari normally disabled interrupt requests and shut down the ANTIC chip during vocal output. The audible output is extremely distorted speech when the screen is on. The Commodore 64 made use of the 64's embedded SID audio chip.
Atari
[edit]Arguably, the first speech system integrated into anoperating systemwas the circa 1983 unreleased Atari1400XL/1450XLcomputers. These used the Votrax SC01 chip and afinite state machineto enable World English Spelling text-to-speech synthesis.[75]
TheAtari STcomputers were sold with "stspeech.tos" on floppy disk.
Apple
[edit]The first speech system integrated into anoperating systemthat shipped in quantity wasApple Computer'sMacInTalk.The software was licensed from third-party developers Joseph Katz and Mark Barton (later, SoftVoice, Inc.) and was featured during the 1984 introduction of the Macintosh computer. This January demo required 512 kilobytes of RAM memory. As a result, it could not run in the 128 kilobytes of RAM the first Mac actually shipped with.[76]So, the demo was accomplished with a prototype 512k Mac, although those in attendance were not told of this and the synthesis demo created considerable excitement for the Macintosh. In the early 1990s Apple expanded its capabilities offering system wide text-to-speech support. With the introduction of faster PowerPC-based computers they included higher quality voice sampling. Apple also introducedspeech recognitioninto its systems which provided a fluid command set. More recently, Apple has added sample-based voices. Starting as a curiosity, the speech system of AppleMacintoshhas evolved into a fully supported program,PlainTalk,for people with vision problems.VoiceOverwas for the first time featured in 2005 inMac OS X Tiger(10.4). During 10.4 (Tiger) and first releases of 10.5 (Leopard) there was only one standard voice shipping with Mac OS X. Starting with 10.6 (Snow Leopard), the user can choose out of a wide range list of multiple voices. VoiceOver voices feature the taking of realistic-sounding breaths between sentences, as well as improved clarity at high read rates over PlainTalk. Mac OS X also includessay,acommand-line basedapplication that converts text to audible speech. TheAppleScriptStandard Additions includes a say verb that allows a script to use any of the installed voices and to control the pitch, speaking rate and modulation of the spoken text.
Amazon
[edit]Used inAlexaand asSoftware as a Servicein AWS[77](from 2017).
AmigaOS
[edit]
The second operating system to feature advanced speech synthesis capabilities wasAmigaOS,introduced in 1985. The voice synthesis was licensed byCommodore Internationalfrom SoftVoice, Inc., who also developed the originalMacinTalktext-to-speech system. It featured a complete system of voice emulation for American English, with both male and female voices and "stress" indicator markers, made possible through theAmiga's audiochipset.[78]The synthesis system was divided into a translator library which converted unrestricted English text into a standard set of phonetic codes and a narrator device which implemented a formant model of speech generation.. AmigaOS also featured a high-level "Speak Handler",which allowed command-line users to redirect text output to speech. Speech synthesis was occasionally used in third-party programs, particularly word processors and educational software. The synthesis software remained largely unchanged from the first AmigaOS release and Commodore eventually removed speech synthesis support from AmigaOS 2.1 onward.
Despite the American English phoneme limitation, an unofficial version with multilingual speech synthesis was developed. This made use of an enhanced version of the translator library which could translate a number of languages, given a set of rules for each language.[79]
Microsoft Windows
[edit]ModernWindowsdesktop systems can useSAPI 4andSAPI 5components to support speech synthesis andspeech recognition.SAPI 4.0 was available as an optional add-on forWindows 95andWindows 98.Windows 2000addedNarrator,a text-to-speech utility for people who have visual impairment. Third-party programs such as JAWS for Windows, Window-Eyes, Non-visual Desktop Access, Supernova and System Access can perform various text-to-speech tasks such as reading text aloud from a specified website, email account, text document, the Windows clipboard, the user's keyboard typing, etc. Not all programs can use speech synthesis directly.[80]Some programs can use plug-ins, extensions or add-ons to read text aloud. Third-party programs are available that can read text from the system clipboard.
Microsoft Speech Serveris a server-based package for voice synthesis and recognition. It is designed for network use withweb applicationsandcall centers.
Votrax
[edit]From 1971 to 1996, Votrax produced a number of commercial speech synthesizer components. A Votrax synthesizer was included in the first generation Kurzweil Reading Machine for the Blind.
Text-to-speech systems
[edit]Text-to-speech (TTS) refers to the ability of computers to read text aloud. A TTS engine converts written text to a phonemic representation, then converts the phonemic representation to waveforms that can be output as sound. TTS engines with different languages, dialects and specialized vocabularies are available through third-party publishers.[81]
Android
[edit]Version 1.6 ofAndroidadded support for speech synthesis (TTS).[82]
Internet
[edit]Currently, there are a number ofapplications,pluginsand gadgets that can read messages directly from ane-mail clientand web pages from aweb browserorGoogle Toolbar.Some specialized software can narrateRSS-feeds.On one hand, online RSS-narrators simplify information delivery by allowing users to listen to their favourite news sources and to convert them topodcasts.On the other hand, on-line RSS-readers are available on almost any personal computer connected to the Internet. Users can download generated audio files to portable devices, e.g. with a help ofpodcastreceiver, and listen to them while walking, jogging or commuting to work.
A growing field in Internet based TTS is web-basedassistive technology,e.g. 'Browsealoud' from a UK company andReadspeaker.It can deliver TTS functionality to anyone (for reasons of accessibility, convenience, entertainment or information) with access to a web browser. The non-profit projectPediaphonwas created in 2006 to provide a similar web-based TTS interface to the Wikipedia.[83]
Other work is being done in the context of theW3Cthrough the W3C Audio Incubator Group with the involvement of The BBC and Google Inc.
Open source
[edit]Someopen-source softwaresystems are available, such as:
- eSpeakwhich supports a broad range of languages.
- Festival Speech Synthesis Systemwhich uses diphone-based synthesis, as well as more modern and better-sounding techniques.
- gnuspeechwhich uses articulatory synthesis[84]from theFree Software Foundation.
Others
[edit]- Following the commercial failure of the hardware-based Intellivoice, gaming developers sparingly used software synthesis in later games[citation needed].Earlier systems from Atari, such as theAtari 5200(Baseball) and theAtari 2600(Quadrunand Open Sesame), also had games utilizing software synthesis.[citation needed]
- Somee-book readers,such as theAmazon Kindle,SamsungE6,PocketBook eReaderPro,enTourage eDGe,and the Bebook Neo.
- TheBBC Microincorporated the Texas Instruments TMS5220 speech synthesis chip,
- Some models of Texas Instruments home computers produced in 1979 and 1981 (Texas Instruments TI-99/4 and TI-99/4A) were capable of text-to-phoneme synthesis or reciting complete words and phrases (text-to-dictionary), using a very popular Speech Synthesizer peripheral. TI used a proprietarycodecto embed complete spoken phrases into applications, primarily video games.[85]
- IBM'sOS/2 Warp 4included VoiceType, a precursor toIBM ViaVoice.
- GPSNavigation units produced byGarmin,Magellan,TomTomand others use speech synthesis for automobile navigation.
- Yamahaproduced a music synthesizer in 1999, theYamaha FS1Rwhich included a Formant synthesis capability. Sequences of up to 512 individual vowel and consonant formants could be stored and replayed, allowing short vocal phrases to be synthesized.
Digital sound-alikes
[edit]At the 2018Conference on Neural Information Processing Systems(NeurIPS) researchers fromGooglepresented the work 'Transfer Learning from Speaker Verification to Multispeaker Text-To-Speech Synthesis', whichtransfers learningfromspeaker verificationto achieve text-to-speech synthesis, that can be made to sound almost like anybody from a speech sample of only 5 seconds.[86]
Also researchers fromBaidu Researchpresented avoice cloningsystem with similar aims at the 2018 NeurIPS conference,[87]though the result is rather unconvincing.
By 2019 the digital sound-alikes found their way to the hands of criminals asSymantecresearchers know of 3 cases where digital sound-alikes technology has been used for crime.[88][89]
This increases the stress on the disinformation situation coupled with the facts that
- Human image synthesissince the early 2000s has improved beyond the point of human's inability to tell a real human imaged with a real camera from a simulation of a human imaged with a simulation of a camera.
- 2D video forgery techniques were presented in 2016 that allownear real-timecounterfeiting offacial expressionsin existing 2D video.[90]
- InSIGGRAPH2017 an audio driven digital look-alike of upper torso of Barack Obama was presented by researchers fromUniversity of Washington.It was driven only by a voice track as source data for the animation after the training phase to acquirelip syncand wider facial information from training material consisting of 2D videos with audio had been completed.[91]
In March 2020, afreewareweb application called15.aithat generates high-quality voices from an assortment of fictional characters from a variety of media sources was released.[92]Initial characters includedGLaDOSfromPortal,Twilight SparkleandFluttershyfrom the showMy Little Pony: Friendship Is Magic,and theTenth DoctorfromDoctor Who.
Speech synthesis markup languages
[edit]A number ofmarkup languageshave been established for the rendition of text as speech in anXML-compliant format. The most recent isSpeech Synthesis Markup Language(SSML), which became aW3C recommendationin 2004. Older speech synthesis markup languages include Java Speech Markup Language (JSML) andSABLE.Although each of these was proposed as a standard, none of them have been widely adopted.[citation needed]
Speech synthesis markup languages are distinguished from dialogue markup languages.VoiceXML,for example, includes tags related to speech recognition, dialogue management and touchtone dialing, in addition to text-to-speech markup.[citation needed]
Applications
[edit]Speech synthesis has long been a vital assistive technology tool and its application in this area is significant and widespread. It allows environmental barriers to be removed for people with a wide range of disabilities. The longest application has been in the use ofscreen readersfor people with visual impairment, but text-to-speech systems are now commonly used by people withdyslexiaand otherreading disabilitiesas well as by pre-literate children.[93]They are also frequently employed to aid those with severespeech impairmentusually through a dedicatedvoice output communication aid.[94]Work to personalize a synthetic voice to better match a person's personality or historical voice is becoming available.[95]A noted application, of speech synthesis, was theKurzweil Reading Machine for the Blindwhich incorporated text-to-phonetics software based on work fromHaskins Laboratoriesand a black-box synthesizer built byVotrax.[96]

Speech synthesis techniques are also used in entertainment productions such as games and animations. In 2007, Animo Limited announced the development of a software application package based on its speech synthesis software FineSpeech, explicitly geared towards customers in the entertainment industries, able to generate narration and lines of dialogue according to user specifications.[97]The application reached maturity in 2008, when NECBiglobeannounced a web service that allows users to create phrases from the voices of characters from the JapaneseanimeseriesCode Geass: Lelouch of the Rebellion R2.[98]15.ai has been frequently used forcontent creationin variousfandoms,including theMy Little Pony: Friendship Is Magicfandom,theTeam Fortress 2fandom, thePortalfandom, and theSpongeBob SquarePantsfandom.[citation needed]
Text-to-speech for disability and impaired communication aids have become widely available. Text-to-speech is also finding new applications; for example, speech synthesis combined withspeech recognitionallows for interaction with mobile devices vianatural language processinginterfaces. Some users have also created AIvirtual assistantsusing 15.ai and external voice control software.[99][100]
Text-to-speech is also used in second language acquisition. Voki, for instance, is an educational tool created by Oddcast that allows users to create their own talking avatar, using different accents. They can be emailed, embedded on websites or shared on social media.
Content creators have used voice cloning tools to recreate their voices for podcasts,[101][102]narration,[103]and comedy shows.[104][105][106]Publishers and authors have also used such software to narrate audiobooks and newsletters.[107][108]Another area of application is AI video creation with talking heads. Webapps and video editors like Elai.io orSynthesiaallow users to create video content involving AI avatars, who are made to speak using text-to-speech technology.[109][110]
Speech synthesis is a valuable computational aid for the analysis and assessment of speech disorders. Avoice qualitysynthesizer, developed by Jorge C. Lucero et al. at theUniversity of Brasília,simulates the physics ofphonationand includes models of vocal frequency jitter and tremor, airflow noise and laryngeal asymmetries.[46]The synthesizer has been used to mimic thetimbreofdysphonicspeakers with controlled levels of roughness, breathiness and strain.[47]
Singing synthesis
[edit]See also
[edit]References
[edit]- ^Allen, Jonathan; Hunnicutt, M. Sharon; Klatt, Dennis (1987).From Text to Speech: The MITalk system.Cambridge University Press.ISBN978-0-521-30641-6.
- ^Rubin, P.; Baer, T.; Mermelstein, P. (1981). "An articulatory synthesizer for perceptual research".Journal of the Acoustical Society of America.70(2): 321–328.Bibcode:1981ASAJ...70..321R.doi:10.1121/1.386780.
- ^van Santen, Jan P. H.; Sproat, Richard W.; Olive, Joseph P.; Hirschberg, Julia (1997).Progress in Speech Synthesis.Springer.ISBN978-0-387-94701-3.
- ^Van Santen, J. (April 1994). "Assignment of segmental duration in text-to-speech synthesis".Computer Speech & Language.8(2): 95–128.doi:10.1006/csla.1994.1005.
- ^History and Development of Speech Synthesis,Helsinki University of Technology, Retrieved on November 4, 2006
- ^Mechanismus der menschlichen Sprache nebst der Beschreibung seiner sprechenden Maschine( "Mechanism of the human speech with description of its speaking machine", J. B. Degen, Wien).(in German)
- ^Mattingly, Ignatius G. (1974). Sebeok, Thomas A. (ed.)."Speech synthesis for phonetic and phonological models"(PDF).Current Trends in Linguistics.12.Mouton, The Hague: 2451–2487. Archived fromthe original(PDF)on 2013-05-12.Retrieved2011-12-13.
- ^Klatt, D (1987). "Review of text-to-speech conversion for English".Journal of the Acoustical Society of America.82(3): 737–93.Bibcode:1987ASAJ...82..737K.doi:10.1121/1.395275.PMID2958525.
- ^Lambert, Bruce (March 21, 1992)."Louis Gerstman, 61, a Specialist In Speech Disorders and Processes".The New York Times.
- ^"Arthur C. Clarke Biography".Archived fromthe originalon December 11, 1997.Retrieved5 December2017.
- ^"Where" HAL "First Spoke (Bell Labs Speech Synthesis website)".Bell Labs. Archived fromthe originalon 2000-04-07.Retrieved2010-02-17.
- ^Anthropomorphic Talking Robot Waseda-Talker SeriesArchived2016-03-04 at theWayback Machine
- ^Gray, Robert M. (2010)."A History of Realtime Digital Speech on Packet Networks: Part II of Linear Predictive Coding and the Internet Protocol"(PDF).Found. Trends Signal Process.3(4): 203–303.doi:10.1561/2000000036.ISSN1932-8346.Archived(PDF)from the original on 2022-10-09.
- ^Zheng, F.; Song, Z.; Li, L.; Yu, W. (1998)."The Distance Measure for Line Spectrum Pairs Applied to Speech Recognition"(PDF).Proceedings of the 5th International Conference on Spoken Language Processing (ICSLP'98)(3): 1123–6.Archived(PDF)from the original on 2022-10-09.
- ^ab"List of IEEE Milestones".IEEE.Retrieved15 July2019.
- ^ab"Fumitada Itakura Oral History".IEEE Global History Network. 20 May 2009.Retrieved2009-07-21.
- ^Billi, Roberto; Canavesio, Franco;Ciaramella, Alberto;Nebbia, Luciano (1 November 1995). "Interactive voice technology at work: The CSELT experience".Speech Communication.17(3): 263–271.doi:10.1016/0167-6393(95)00030-R.
- ^Sproat, Richard W. (1997).Multilingual Text-to-Speech Synthesis: The Bell Labs Approach.Springer.ISBN978-0-7923-8027-6.
- ^[TSI Speech+ & other speaking calculators]
- ^Gevaryahu, Jonathan, [ "TSI S14001A Speech Synthesizer LSI Integrated Circuit Guide" ][dead link]
- ^Breslow, et al.US 4326710:"Talking electronic game", April 27, 1982
- ^Voice Chess Challenger
- ^Gaming's most important evolutionsArchived2011-06-15 at theWayback Machine,GamesRadar
- ^Adlum, Eddie (November 1985)."The Replay Years: Reflections from Eddie Adlum".RePlay.Vol. 11, no. 2. pp. 134-175 (160-3).
- ^Szczepaniak, John (2014).The Untold History of Japanese Game Developers.Vol. 1. SMG Szczepaniak. pp. 544–615.ISBN978-0992926007.
- ^"A Short History of Computalker".Smithsonian Speech Synthesis History Project.
- ^CadeMetz (2020-08-20)."Ann Syrdal, Who Helped Give Computers a Female Voice, Dies at 74".The New York Times.Retrieved2020-08-23.
- ^Kurzweil, Raymond(2005).The Singularity is Near.Penguin Books.ISBN978-0-14-303788-0.
- ^Taylor, Paul (2009).Text-to-speech synthesis.Cambridge, UK: Cambridge University Press. p.3.ISBN9780521899277.
- ^Alan W. Black,Perfect synthesis for all of the people all of the time.IEEE TTS Workshop 2002.
- ^John Kominek andAlan W. Black.(2003). CMU ARCTIC databases for speech synthesis. CMU-LTI-03-177. Language Technologies Institute, School of Computer Science, Carnegie Mellon University.
- ^Julia Zhang.Language Generation and Speech Synthesis in Dialogues for Language Learning,masters thesis, Section 5.6 on page 54.
- ^William Yang Wang and Kallirroi Georgila. (2011).Automatic Detection of Unnatural Word-Level Segments in Unit-Selection Speech Synthesis,IEEE ASRU 2011.
- ^"Pitch-Synchronous Overlap and Add (PSOLA) Synthesis".Archived fromthe originalon February 22, 2007.Retrieved2008-05-28.
- ^T. Dutoit, V. Pagel, N. Pierret, F. Bataille, O. van der Vrecken.The MBROLA Project: Towards a set of high quality speech synthesizers of use for non commercial purposes.ICSLP Proceedings,1996.
- ^Muralishankar, R; Ramakrishnan, A.G.; Prathibha, P (2004). "Modification of Pitch using DCT in the Source Domain".Speech Communication.42(2): 143–154.doi:10.1016/j.specom.2003.05.001.
- ^"Education: Marvel of The Bronx".Time.1974-04-01.ISSN0040-781X.Retrieved2019-05-28.
- ^"1960 - Rudy the Robot - Michael Freeman (American)".cyberneticzoo.com.2010-09-13.Retrieved2019-05-23.
- ^New York Magazine.New York Media, LLC. 1979-07-30.
- ^The Futurist.World Future Society. 1978. pp. 359, 360, 361.
- ^L.F. Lamel,J.L. Gauvain, B. Prouts, C. Bouhier, R. Boesch.Generation and Synthesis of Broadcast Messages,Proceedings ESCA-NATO Workshop and Applications of Speech Technology,September 1993.
- ^Dartmouth College:Music and ComputersArchived2011-06-08 at theWayback Machine,1993.
- ^Examples includeAstro Blaster,Space Fury,andStar Trek: Strategic Operations Simulator
- ^Examples includeStar Wars,Firefox,Return of the Jedi,Road Runner,The Empire Strikes Back,Indiana Jones and the Temple of Doom,720°,Gauntlet,Gauntlet II,A.P.B.,Paperboy,RoadBlasters,Vindicators Part II,Escape from the Planet of the Robot Monsters.
- ^John Holmes and Wendy Holmes (2001).Speech Synthesis and Recognition(2nd ed.). CRC.ISBN978-0-7484-0856-6.
- ^abLucero, J. C.; Schoentgen, J.; Behlau, M. (2013)."Physics-based synthesis of disordered voices"(PDF).Interspeech 2013.Lyon, France: International Speech Communication Association: 587–591.doi:10.21437/Interspeech.2013-161.S2CID17451802.RetrievedAug 27,2015.
- ^abEnglert, Marina; Madazio, Glaucya; Gielow, Ingrid; Lucero, Jorge; Behlau, Mara (2016). "Perceptual error identification of human and synthesized voices".Journal of Voice.30(5): 639.e17–639.e23.doi:10.1016/j.jvoice.2015.07.017.PMID26337775.
- ^"The HMM-based Speech Synthesis System".Hts.sp.nitech.ac.j.Retrieved2012-02-22.
- ^Remez, R.; Rubin, P.; Pisoni, D.; Carrell, T. (22 May 1981)."Speech perception without traditional speech cues"(PDF).Science.212(4497): 947–949.Bibcode:1981Sci...212..947R.doi:10.1126/science.7233191.PMID7233191.Archived fromthe original(PDF)on 2011-12-16.Retrieved2011-12-14.
- ^Valle, Rafael (2020). "Mellotron: Multispeaker expressive voice synthesis by conditioning on rhythm, pitch and global style tokens".arXiv:1910.11997[eess].
- ^Kurosawa, Yuki (2021-01-19)."ゲームキャラ âm thanh đọc み thượng げソフト “15.ai” công khai trung. 『Undertale』や『Portal』のキャラに hảo きなセリフを ngôn ってもらえる ".AUTOMATON.Archivedfrom the original on 2021-01-19.Retrieved2021-01-19.
- ^Yoshiyuki, Furushima (2021-01-18)."『Portal』のGLaDOSや『UNDERTALE』のサンズがテキストを đọc み thượng げてくれる. Văn chương に込められた cảm tình まで tái hiện することを mục chỉ すサービス “15.ai” が thoại đề に ".Denfaminicogamer.Archivedfrom the original on 2021-01-18.Retrieved2021-01-18.
- ^"Generative AI comes for cinema dubbing: Audio AI startup ElevenLabs raises pre-seed".Sifted.January 23, 2023.Retrieved2023-02-03.
- ^Ashworth, Boone (April 12, 2023)."AI Can Clone Your Favorite Podcast Host's Voice".Wired.Retrieved2023-04-25.
- ^WIRED Staff."This Podcast Is Not Hosted by AI Voice Clones. We Swear".Wired.ISSN1059-1028.Retrieved2023-07-25.
- ^Wiggers, Kyle (2023-06-20)."Voice-generating platform ElevenLabs raises $19M, launches detection tool".TechCrunch.Retrieved2023-07-25.
- ^Bonk, Lawrence."ElevenLabs' Powerful New AI Tool Lets You Make a Full Audiobook in Minutes".Lifewire.Retrieved2023-07-25.
- ^Zhu, Jian (2020-05-25)."Probing the phonetic and phonological knowledge of tones in Mandarin TTS models".Speech Prosody 2020.ISCA: ISCA: 930–934.arXiv:1912.10915.doi:10.21437/speechprosody.2020-190.S2CID209444942.
- ^Smith, Hannah; Mansted, Katherine (April 1, 2020).Weaponised deep fakes: National security and democracy.Vol. 28.Australian Strategic Policy Institute.pp. 11–13.ISSN2209-9689.
{{cite book}}
:CS1 maint: date and year (link) - ^Lyu, Siwei (2020)."Deepfake Detection: Current Challenges and Next Steps".2020 IEEE International Conference on Multimedia & Expo Workshops (ICMEW).pp. 1–6.arXiv:2003.09234.doi:10.1109/icmew46912.2020.9105991.ISBN978-1-7281-1485-9.S2CID214605906.Retrieved2022-06-29.
- ^Diakopoulos, Nicholas; Johnson, Deborah (June 2020)."Anticipating and addressing the ethical implications of deepfakes in the context of elections".New Media & Society.23(7) (published 2020-06-05): 2072–2098.doi:10.1177/1461444820925811.ISSN1461-4448.S2CID226196422.
- ^Murphy, Margi (20 February 2024)."Deepfake Audio Boom Exploits One Billion-Dollar Startup's AI".Bloomberg.
- ^Chadha, Anupama; Kumar, Vaibhav; Kashyap, Sonu; Gupta, Mayank (2021), Singh, Pradeep Kumar; Wierzchoń, Sławomir T.; Tanwar, Sudeep; Ganzha, Maria (eds.),"Deepfake: An Overview",Proceedings of Second International Conference on Computing, Communications, and Cyber-Security,Lecture Notes in Networks and Systems, vol. 203, Singapore: Springer Singapore, pp. 557–566,doi:10.1007/978-981-16-0733-2_39,ISBN978-981-16-0732-5,S2CID236666289,retrieved2022-06-29
- ^"AI gave Val Kilmer his voice back. But critics worry the technology could be misused".Washington Post.ISSN0190-8286.Retrieved2022-06-29.
- ^Etienne, Vanessa (August 19, 2021)."Val Kilmer Gets His Voice Back After Throat Cancer Battle Using AI Technology: Hear the Results".PEOPLE.com.Retrieved2022-07-01.
- ^Newman, Lily Hay."AI-Generated Voice Deepfakes Aren't Scary Good—Yet".Wired.ISSN1059-1028.Retrieved2023-07-25.
- ^"Speech synthesis".World Wide Web Organization.
- ^"Blizzard Challenge".Festvox.org.Retrieved2012-02-22.
- ^"Smile -and the world can hear you".University of Portsmouth. January 9, 2008. Archived fromthe originalon May 17, 2008.
- ^"Smile – And The World Can Hear You, Even If You Hide".Science Daily.January 2008.
- ^Drahota, A. (2008)."The vocal communication of different kinds of smile"(PDF).Speech Communication.50(4): 278–287.doi:10.1016/j.specom.2007.10.001.S2CID46693018.Archived fromthe original(PDF)on 2013-07-03.
- ^Muralishankar, R.; Ramakrishnan, A. G.; Prathibha, P. (February 2004). "Modification of pitch using DCT in the source domain".Speech Communication.42(2): 143–154.doi:10.1016/j.specom.2003.05.001.
- ^Prathosh, A. P.; Ramakrishnan, A. G.; Ananthapadmanabha, T. V. (December 2013). "Epoch extraction based on integrated linear prediction residual using plosion index".IEEE Trans. Audio Speech Language Processing.21(12): 2471–2480.doi:10.1109/TASL.2013.2273717.S2CID10491251.
- ^EE Times. "TI will exit dedicated speech-synthesis chips, transfer products to SensoryArchived2012-05-28 at theWayback Machine."June 14, 2001.
- ^"1400XL/1450XL Speech Handler External Reference Specification"(PDF).Archived fromthe original(PDF)on 2012-03-24.Retrieved2012-02-22.
- ^"It Sure Is Great To Get Out Of That Bag!".folklore.org.Retrieved2013-03-24.
- ^"Amazon Polly".Amazon Web Services, Inc.Retrieved2020-04-28.
- ^Miner, Jay;et al. (1991).Amiga Hardware Reference Manual(3rd ed.).Addison-WesleyPublishing Company, Inc.ISBN978-0-201-56776-2.
- ^Devitt, Francesco (30 June 1995)."Translator Library (Multilingual-speech version)".Archived fromthe originalon 26 February 2012.Retrieved9 April2013.
- ^"Accessibility Tutorials for Windows XP: Using Narrator".Microsoft. 2011-01-29. Archived fromthe originalon June 21, 2003.Retrieved2011-01-29.
- ^"How to configure and use Text-to-Speech in Windows XP and in Windows Vista".Microsoft. 2007-05-07.Retrieved2010-02-17.
- ^Jean-Michel Trivi (2009-09-23)."An introduction to Text-To-Speech in Android".Android-developers.blogspot.com.Retrieved2010-02-17.
- ^Andreas Bischoff,The Pediaphon – Speech Interface to the free Wikipedia Encyclopedia for Mobile Phones,PDA's and MP3-Players, Proceedings of the 18th International Conference on Database and Expert Systems Applications, Pages: 575–579ISBN0-7695-2932-1,2007
- ^"gnuspeech".Gnu.org.Retrieved2010-02-17.
- ^"Smithsonian Speech Synthesis History Project (SSSHP) 1986–2002".Mindspring.com. Archived fromthe originalon 2013-10-03.Retrieved2010-02-17.
- ^ Jia, Ye; Zhang, Yu; Weiss, Ron J. (2018-06-12), "Transfer Learning from Speaker Verification to Multispeaker Text-To-Speech Synthesis",Advances in Neural Information Processing Systems,31:4485–4495,arXiv:1806.04558
- ^ Arık, Sercan Ö.; Chen, Jitong; Peng, Kainan; Ping, Wei; Zhou, Yanqi (2018),"Neural Voice Cloning with a Few Samples",Advances in Neural Information Processing Systems,31,arXiv:1802.06006
- ^ "Fake voices 'help cyber-crooks steal cash'".bbc.com.BBC.2019-07-08.Retrieved2019-09-11.
- ^ Drew, Harwell (2019-09-04)."An artificial-intelligence first: Voice-mimicking software reportedly used in a major theft".Washington Post.Retrieved2019-09-08.
- ^Thies, Justus (2016)."Face2Face: Real-time Face Capture and Reenactment of RGB Videos".Proc. Computer Vision and Pattern Recognition (CVPR), IEEE.Retrieved2016-06-18.
- ^Suwajanakorn, Supasorn; Seitz, Steven; Kemelmacher-Shlizerman, Ira (2017),Synthesizing Obama: Learning Lip Sync from Audio,University of Washington,retrieved2018-03-02
- ^ Ng, Andrew (2020-04-01)."Voice Cloning for the Masses".deeplearning.ai.The Batch. Archived fromthe originalon 2020-08-07.Retrieved2020-04-02.
- ^Brunow, David A.; Cullen, Theresa A. (2021-07-03)."Effect of Text-to-Speech and Human Reader on Listening Comprehension for Students with Learning Disabilities".Computers in the Schools.38(3): 214–231.doi:10.1080/07380569.2021.1953362.hdl:11244/316759.ISSN0738-0569.S2CID243101945.
- ^Triandafilidi, Ioanis I.; Tatarnikova, T. M.; Poponin, A. S. (2022-05-30)."Speech Synthesis System for People with Disabilities".2022 Wave Electronics and its Application in Information and Telecommunication Systems (WECONF).St. Petersburg, Russian Federation: IEEE. pp. 1–5.doi:10.1109/WECONF55058.2022.9803600.ISBN978-1-6654-7083-4.S2CID250118756.
- ^Zhao, Yunxin; Song, Minguang; Yue, Yanghao; Kuruvilla-Dugdale, Mili (2021-07-27)."Personalizing TTS Voices for Progressive Dysarthria".2021 IEEE EMBS International Conference on Biomedical and Health Informatics (BHI).Athens, Greece: IEEE. pp. 1–4.doi:10.1109/BHI50953.2021.9508522.ISBN978-1-6654-0358-0.S2CID236982893.
- ^"Evolution of Reading Machines for the Blind: Haskins Laboratories" Research as a Case History "(PDF).Journal of Rehabilitation Research and Development.21(1). 1984.
- ^"Speech Synthesis Software for Anime Announced".Anime News Network.2007-05-02.Retrieved2010-02-17.
- ^"Code Geass Speech Synthesizer Service Offered in Japan".Animenewsnetwork.com. 2008-09-09.Retrieved2010-02-17.
- ^Kurosawa, Yuki (2021-01-19)."ゲームキャラ âm thanh đọc み thượng げソフト “15.ai” công khai trung. 『Undertale』や『Portal』のキャラに hảo きなセリフを ngôn ってもらえる ".AUTOMATON.Archivedfrom the original on 2021-01-19.Retrieved2021-01-19.
- ^Yoshiyuki, Furushima (2021-01-18)."『Portal』のGLaDOSや『UNDERTALE』のサンズがテキストを đọc み thượng げてくれる. Văn chương に込められた cảm tình まで tái hiện することを mục chỉ すサービス “15.ai” が thoại đề に ".Denfaminicogamer.Archivedfrom the original on 2021-01-18.Retrieved2021-01-18.
- ^"Now hear this: Voice cloning AI startup ElevenLabs nabs $19M from a16z and other heavy hitters".VentureBeat.2023-06-20.Retrieved2023-07-25.
- ^"Sztuczna inteligencja czyta głosem Jarosława Kuźniara. Rewolucja w radiu i podcastach".Press.pl(in Polish). April 9, 2023.Retrieved2023-04-25.
- ^Ashworth, Boone (April 12, 2023)."AI Can Clone Your Favorite Podcast Host's Voice".Wired.Retrieved2023-04-25.
- ^Knibbs, Kate."Generative AI Podcasts Are Here. Prepare to Be Bored".Wired.ISSN1059-1028.Retrieved2023-07-25.
- ^Suciu, Peter."Arrested Succession Parody On YouTube Features 'Narration' By AI-Generated Ron Howard".Forbes.Retrieved2023-07-25.
- ^Fadulu, Lola (2023-07-06)."Can A.I. Be Funny? This Troupe Thinks So".The New York Times.ISSN0362-4331.Retrieved2023-07-25.
- ^Kanetkar, Riddhi."Hot AI startup ElevenLabs, founded by ex-Google and Palantir staff, is set to raise $18 million at a $100 million valuation. Check out the 14-slide pitch deck it used for its $2 million pre-seed".Business Insider.Retrieved2023-07-25.
- ^"AI-Generated Voice Firm Clamps Down After 4chan Makes Celebrity Voices for Abuse".www.vice.com.January 30, 2023.Retrieved2023-02-03.
- ^"Usage of text-to-speech in AI video generation".elai.io.Retrieved10 August2022.
- ^"AI Text to speech for videos".synthesia.io.Retrieved12 October2023.
- ^Bruno, Chelsea A (2014-03-25).Vocal Synthesis and Deep Listening(Master of Music Music thesis). Florida International University.doi:10.25148/etd.fi14040802.
External links
[edit]
- Speech synthesisatCurlie
- Simulated singing with the singing robot Pavarobottior a description from theBBConhow the robot synthesized the singing.