p-value
Innull-hypothesis significance testing,the-value[note 1]is the probability of obtaining test results at least as extreme as theresult actually observed,under the assumption that thenull hypothesisis correct.[2][3]A very smallp-value means that such an extreme observedoutcomewould be very unlikely under the null hypothesis. Even though reportingp-values of statistical tests is common practice inacademic publicationsof many quantitative fields, misinterpretation andmisuse of p-valuesis widespread and has been a major topic in mathematics andmetascience.[4][5]In 2016, the American Statistical Association (ASA) made a formal statement that "p-values do not measure the probability that the studied hypothesis is true, or the probability that the data were produced by random chance alone "and that" ap-value, or statistical significance, does not measure the size of an effect or the importance of a result "or" evidence regarding a model or hypothesis ".[6]That said, a 2019 task force by ASA has issued a statement on statistical significance and replicability, concluding with: "p-values and significance tests, when properly applied and interpreted, increase the rigor of the conclusions drawn from data ".[7]
Basic concepts[edit]
In statistics, every conjecture concerning the unknownprobability distributionof a collection of random variables representing the observed datain some study is called astatistical hypothesis.If we state one hypothesis only and the aim of the statistical test is to see whether this hypothesis is tenable, but not to investigate other specific hypotheses, then such a test is called anull hypothesis test.
As our statistical hypothesis will, by definition, state some property of the distribution, thenull hypothesisis the default hypothesis under which that property does not exist. The null hypothesis is typically that some parameter (such as a correlation or a difference between means) in the populations of interest is zero. Our hypothesis might specify the probability distribution ofprecisely, or it might only specify that it belongs to some class of distributions. Often, we reduce the data to a single numerical statistic, e.g.,,whose marginal probability distribution is closely connected to a main question of interest in the study.
Thep-value is used in the context of null hypothesis testing in order to quantify thestatistical significanceof a result, the result being the observed value of the chosen statistic.[note 2]The lower thep-value is, the lower the probability of getting that result if the null hypothesis were true. A result is said to bestatistically significantif it allows us to reject the null hypothesis. All other things being equal, smallerp-values are taken as stronger evidence against the null hypothesis.
Loosely speaking, rejection of the null hypothesis implies that there is sufficient evidence against it.
As a particular example, if a null hypothesis states that a certain summary statisticfollows the standardnormal distributionthen the rejection of this null hypothesis could mean that (i) the mean ofis not 0, or (ii) thevarianceofis not 1, or (iii)is not normally distributed. Different tests of the same null hypothesis would be more or less sensitive to different alternatives. However, even if we do manage to reject the null hypothesis for all 3 alternatives, and even if we know that the distribution is normal and variance is 1, the null hypothesis test does not tell us which non-zero values of the mean are now most plausible. The more independent observations from the same probability distribution one has, the more accurate the test will be, and the higher the precision with which one will be able to determine the mean value and show that it is not equal to zero; but this will also increase the importance of evaluating the real-world or scientific relevance of this deviation.
Definition and interpretation[edit]
Definition[edit]
Thep-value is the probability under the null hypothesis of obtaining a real-valued test statistic at least as extreme as the one obtained. Consider an observed test-statisticfrom unknown distribution.Then thep-valueis what the prior probability would be of observing a test-statistic value at least as "extreme" asif null hypothesiswere true. That is:
- for a one-sided right-tail test-statistic distribution.
- for a one-sided left-tail test-statistic distribution.
- for a two-sided test-statistic distribution. If the distribution ofis symmetric about zero, then
Interpretations[edit]
The error that a practising statistician would consider the more important to avoid (which is a subjective judgment) is called the error of the first kind. The first demand of the mathematical theory is to deduce such test criteria as would ensure that the probability of committing an error of the first kind would equal (or approximately equal, or not exceed) a preassigned number α, such as α = 0.05 or 0.01, etc. This number is called the level of significance.
— Jerzy Neyman, "The Emergence of Mathematical Statistics"[8]
In a significance test, the null hypothesisis rejected if thep-value is less than or equal to a predefined threshold value,which is referred to as the Alpha level orsignificance level.is not derived from the data, but rather is set by the researcher before examining the data.is commonly set to 0.05, though lower Alpha levels are sometimes used. In 2018, a group of statisticians led by Daniel Benjamin proposed the adoption of the 0.005 value as standard value for statistical significance worldwide.[9]
Differentp-values based on independent sets of data can be combined, for instance usingFisher's combined probability test.
Distribution[edit]
Thep-value is a function of the chosen test statisticand is therefore arandom variable.If the null hypothesis fixes the probability distribution ofprecisely (e.g.whereis the only parameter), and if that distribution is continuous, then when the null-hypothesis is true, thep-value isuniformly distributedbetween 0 and 1. Regardless of the truth of the,thep-value is not fixed; if the same test is repeated independently with fresh data, one will typically obtain a differentp-value in each iteration.
Usually only a singlep-value relating to a hypothesis is observed, so thep-value is interpreted by a significance test, and no effort is made to estimate the distribution it was drawn from. When a collection ofp-values are available (e.g. when considering a group of studies on the same subject), the distribution ofp-values is sometimes called ap-curve.[10] Ap-curve can be used to assess the reliability of scientific literature, such as by detecting publication bias orp-hacking. [10][11]
Distribution for composite hypothesis[edit]
In parametric hypothesis testing problems, asimple or point hypothesisrefers to a hypothesis where the parameter's value is assumed to be a single number. In contrast, in acomposite hypothesisthe parameter's value is given by a set of numbers. When the null-hypothesis is composite (or the distribution of the statistic is discrete), then when the null-hypothesis is true the probability of obtaining ap-value less than or equal to any number between 0 and 1 is still less than or equal to that number. In other words, it remains the case that very small values are relatively unlikely if the null-hypothesis is true, and that a significance test at levelis obtained by rejecting the null-hypothesis if thep-value is less than or equal to.[12][13]
For example, when testing the null hypothesis that a distribution is normal with a mean less than or equal to zero against the alternative that the mean is greater than zero (,variance known), the null hypothesis does not specify the exact probability distribution of the appropriate test statistic. In this example that would be theZ-statisticbelonging to the one-sided one-sampleZ-test. For each possible value of the theoretical mean, theZ-test statistic has a different probability distribution. In these circumstances thep-value is defined by taking the least favorable null-hypothesis case, which is typically on the border between null and alternative. This definition ensures the complementarity of p-values and Alpha -levels:means one only rejects the null hypothesis if thep-value is less than or equal to,and the hypothesis test will indeed have amaximumtype-1 error rate of.
Usage[edit]
Thep-value is widely used instatistical hypothesis testing,specifically in null hypothesis significance testing. In this method, before conducting the study, one first chooses a model (thenull hypothesis) and the Alpha levelα(most commonly 0.05). After analyzing the data, if thep-value is less thanα,that is taken to mean that the observed data is sufficiently inconsistent with thenull hypothesisfor the null hypothesis to be rejected. However, that does not prove that the null hypothesis is false. Thep-value does not, in itself, establish probabilities of hypotheses. Rather, it is a tool for deciding whether to reject the null hypothesis.[14]
Misuse[edit]
According to theASA,there is widespread agreement thatp-values are often misused and misinterpreted.[3]One practice that has been particularly criticized is accepting the alternative hypothesis for anyp-value nominally less than 0.05 without other supporting evidence. Althoughp-values are helpful in assessing how incompatible the data are with a specified statistical model, contextual factors must also be considered, such as "the design of a study, the quality of the measurements, the external evidence for the phenomenon under study, and the validity of assumptions that underlie the data analysis".[3]Another concern is that thep-value is often misunderstood as being the probability that the null hypothesis is true.[3][15]
Some statisticians have proposed abandoningp-values and focusing more on other inferential statistics,[3]such asconfidence intervals,[16][17]likelihood ratios,[18][19]orBayes factors,[20][21][22]but there is heated debate on the feasibility of these alternatives.[23][24]Others have suggested to remove fixed significance thresholds and to interpretp-values as continuous indices of the strength of evidence against the null hypothesis.[25][26]Yet others suggested to report alongsidep-values the prior probability of a real effect that would be required to obtain a false positive risk (i.e. the probability that there is no real effect) below a pre-specified threshold (e.g. 5%).[27]
That said, in 2019 a task force by ASA had convened to consider the use of statistical methods in scientific studies, specifically hypothesis tests andp-values, and their connection to replicability.[7]It states that "Different measures of uncertainty can complement one another; no single measure serves all purposes", citingp-value as one of these measures. They also stress thatp-values can provide valuable information when considering the specific value as well as when compared to some threshold. In general, it stresses that "p-values and significance tests, when properly applied and interpreted, increase the rigor of the conclusions drawn from data ".
Calculation[edit]
Usually,is atest statistic.A test statistic is the output of ascalarfunction of all the observations. This statistic provides a single number, such as at-statisticor anF-statistic.As such, the test statistic follows a distribution determined by the function used to define that test statistic and the distribution of the input observational data.
For the important case in which the data are hypothesized to be a random sample from a normal distribution, depending on the nature of the test statistic and the hypotheses of interest about its distribution, different null hypothesis tests have been developed. Some such tests are thez-testfor hypotheses concerning the mean of anormal distributionwith known variance, thet-testbased onStudent'st-distributionof a suitable statistic for hypotheses concerning the mean of a normal distribution when the variance is unknown, theF-testbased on theF-distributionof yet another statistic for hypotheses concerning the variance. For data of other nature, for instance, categorical (discrete) data, test statistics might be constructed whose null hypothesis distribution is based on normal approximations to appropriate statistics obtained by invoking thecentral limit theoremfor large samples, as in the case ofPearson's chi-squared test.
Thus computing ap-value requires a null hypothesis, a test statistic (together with deciding whether the researcher is performing aone-tailed testor atwo-tailed test), and data. Even though computing the test statistic on given data may be easy, computing the sampling distribution under the null hypothesis, and then computing itscumulative distribution function(CDF) is often a difficult problem. Today, this computation is done using statistical software, often via numeric methods (rather than exact formulae), but, in the early and mid 20th century, this was instead done via tables of values, and one interpolated or extrapolatedp-values from these discrete values[citation needed].Rather than using a table ofp-values, Fisher instead inverted the CDF, publishing a list of values of the test statistic for given fixedp-values; this corresponds to computing thequantile function(inverse CDF).
Example[edit]
Testing the fairness of a coin[edit]
As an example of a statistical test, an experiment is performed to determine whether acoin flipisfair(equal chance of landing heads or tails) or unfairly biased (one outcome being more likely than the other).
Suppose that the experimental results show the coin turning up heads 14 times out of 20 total flips. The full datawould be a sequence of twenty times the symbol "H" or "T". The statistic on which one might focus could be the total numberof heads. The null hypothesis is that the coin is fair, and coin tosses are independent of one another. If a right-tailed test is considered, which would be the case if one is actually interested in the possibility that the coin is biased towards falling heads, then thep-value of this result is the chance of a fair coin landing on headsat least14 times out of 20 flips. That probability can be computed frombinomial coefficientsas
This probability is thep-value, considering only extreme results that favor heads. This is called aone-tailed test.However, one might be interested in deviations in either direction, favoring either heads or tails. The two-tailedp-value, which considers deviations favoring either heads or tails, may instead be calculated. As thebinomial distributionis symmetrical for a fair coin, the two-sidedp-value is simply twice the above calculated single-sidedp-value: the two-sidedp-value is 0.115.
In the above example:
- Null hypothesis (H0): The coin is fair, with Pr(heads) = 0.5.
- Test statistic: Number of heads.
- Alpha level (designated threshold of significance): 0.05.
- ObservationO:14 heads out of 20 flips.
- Two-tailedp-value of observationOgivenH0= 2 × min(Pr(no. of heads ≥ 14 heads), Pr(no. of heads ≤ 14 heads)) = 2 × min(0.058, 0.978) = 2 × 0.058 = 0.115.
The Pr(no. of heads ≤ 14 heads) = 1 − Pr(no. of heads ≥ 14 heads) + Pr(no. of head = 14) = 1 − 0.058 + 0.036 = 0.978; however, the symmetry of this binomial distribution makes it an unnecessary computation to find the smaller of the two probabilities. Here, the calculatedp-value exceeds 0.05, meaning that the data falls within the range of what would happen 95% of the time, if the coin were fair. Hence, the null hypothesis is not rejected at the 0.05 level.
However, had one more head been obtained, the resultingp-value (two-tailed) would have been 0.0414 (4.14%), in which case the null hypothesis would be rejected at the 0.05 level.
Multistage experiment design[edit]
The difference between the two meanings of "extreme" appear when we consider a multistage experiment for testing the fairness of the coin. Suppose we design the experiment as follows:
- Flip the coin twice. If both comes up heads or tails, end the experiment.
- Else, flip the coin 4 more times.
This experiment has 7 types of outcomes: 2 heads, 2 tails, 5 heads 1 tail,..., 1 head 5 tails. We now calculate thep-value of the "3 heads 3 tails" outcome.
If we use the test statistic,then under the null hypothesis is exactly 1 for two-sidedp-value, and exactlyfor one-sided left-tailp-value, and same for one-sided right-tailp-value.
If we consider every outcome that has equal or lower probability than "3 heads 3 tails" as "at least as extreme", then thep-value is exactly
However, suppose we have planned to simply flip the coin 6 times no matter what happens, then the second definition ofp-value would mean that thep-value of "3 heads 3 tails" is exactly 1.
Thus, the "at least as extreme" definition ofp-value is deeply contextual and depends on what the experimenterplannedto do even in situations that did not occur.
History[edit]

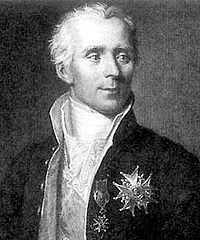

P-value computations date back to the 1700s, where they were computed for thehuman sex ratioat birth, and used to compute statistical significance compared to the null hypothesis of equal probability of male and female births.[28]John Arbuthnotstudied this question in 1710,[29][30][31][32]and examined birth records in London for each of the 82 years from 1629 to 1710. In every year, the number of males born in London exceeded the number of females. Considering more male or more female births as equally likely, the probability of the observed outcome is 1/282,or about 1 in 4,836,000,000,000,000,000,000,000; in modern terms, thep-value. This is vanishingly small, leading Arbuthnot that this was not due to chance, but to divine providence: "From whence it follows, that it is Art, not Chance, that governs." In modern terms, he rejected the null hypothesis of equally likely male and female births at thep= 1/282significance level. This and other work by Arbuthnot is credited as "… the first use of significance tests…"[33]the first example of reasoning about statistical significance,[34]and "… perhaps the first published report of anonparametric test…",[30]specifically thesign test;see details atSign test § History.
The same question was later addressed byPierre-Simon Laplace,who instead used aparametrictest, modeling the number of male births with abinomial distribution:[35]
In the 1770s Laplace considered the statistics of almost half a million births. The statistics showed an excess of boys compared to girls. He concluded by calculation of ap-value that the excess was a real, but unexplained, effect.
Thep-value was first formally introduced byKarl Pearson,in hisPearson's chi-squared test,[36]using thechi-squared distributionand notated as capital P.[36]Thep-values for thechi-squared distribution(for various values ofχ2and degrees of freedom), now notated asP,were calculated in (Elderton 1902), collected in (Pearson 1914,pp. xxxi–xxxiii, 26–28, Table XII) .
Ronald Fisherformalized and popularized the use of thep-value in statistics,[37][38]with it playing a central role in his approach to the subject.[39]In his highly influential bookStatistical Methods for Research Workers(1925), Fisher proposed the levelp= 0.05, or a 1 in 20 chance of being exceeded by chance, as a limit forstatistical significance,and applied this to a normal distribution (as a two-tailed test), thus yielding the rule of two standard deviations (on a normal distribution) for statistical significance (see68–95–99.7 rule).[40][note 3][41]
He then computed a table of values, similar to Elderton but, importantly, reversed the roles ofχ2andp.That is, rather than computingpfor different values ofχ2(and degrees of freedomn), he computed values ofχ2that yield specifiedp-values, specifically 0.99, 0.98, 0.95, 0,90, 0.80, 0.70, 0.50, 0.30, 0.20, 0.10, 0.05, 0.02, and 0.01.[42]That allowed computed values ofχ2to be compared against cutoffs and encouraged the use ofp-values (especially 0.05, 0.02, and 0.01) as cutoffs, instead of computing and reportingp-values themselves. The same type of tables were then compiled in (Fisher & Yates 1938), which cemented the approach.[41]
As an illustration of the application ofp-values to the design and interpretation of experiments, in his following bookThe Design of Experiments(1935), Fisher presented thelady tasting teaexperiment,[43]which is the archetypal example of thep-value.
To evaluate a lady's claim that she (Muriel Bristol) could distinguish by taste how tea is prepared (first adding the milk to the cup, then the tea, or first tea, then milk), she was sequentially presented with 8 cups: 4 prepared one way, 4 prepared the other, and asked to determine the preparation of each cup (knowing that there were 4 of each). In that case, the null hypothesis was that she had no special ability, the test wasFisher's exact test,and thep-value wasso Fisher was willing to reject the null hypothesis (consider the outcome highly unlikely to be due to chance) if all were classified correctly. (In the actual experiment, Bristol correctly classified all 8 cups.)
Fisher reiterated thep= 0.05 threshold and explained its rationale, stating:[44]
It is usual and convenient for experimenters to take 5 per cent as a standard level of significance, in the sense that they are prepared to ignore all results which fail to reach this standard, and, by this means, to eliminate from further discussion the greater part of the fluctuations which chance causes have introduced into their experimental results.
He also applies this threshold to the design of experiments, noting that had only 6 cups been presented (3 of each), a perfect classification would have only yielded ap-value ofwhich would not have met this level of significance.[44]Fisher also underlined the interpretation ofp,as the long-run proportion of values at least as extreme as the data, assuming the null hypothesis is true.
In later editions, Fisher explicitly contrasted the use of thep-value for statistical inference in science with the Neyman–Pearson method, which he terms "Acceptance Procedures".[45]Fisher emphasizes that while fixed levels such as 5%, 2%, and 1% are convenient, the exactp-value can be used, and the strength of evidence can and will be revised with further experimentation. In contrast, decision procedures require a clear-cut decision, yielding an irreversible action, and the procedure is based on costs of error, which, he argues, are inapplicable to scientific research.
Related indices[edit]
TheE-valuecan refer to two concepts, both of which are related to the p-value and both of which play a role inmultiple testing.First,it corresponds to a generic, more robust alternative to the p-valuethat can deal withoptional continuationof experiments. Second, it is also used to abbreviate "expect value", which is theexpectednumber of times that one expects to obtain a test statistic at least as extreme as the one that was actually observed if one assumes that the null hypothesis is true.[46]This expect-value is the product of the number of tests and thep-value.
Theq-valueis the analog of thep-value with respect to thepositive false discovery rate.[47]It is used inmultiple hypothesis testingto maintain statistical power while minimizing thefalse positive rate.[48]
TheProbability of Direction (pd)is theBayesiannumerical equivalent of thep-value.[49]It corresponds to the proportion of theposterior distributionthat is of the median's sign, typically varying between 50% and 100%, and representing the certainty with which an effect is positive or negative.
Second-generation p-valuesextend the concept of p-values by not considering extremely small, practically irrelevanteffect sizesas significant.[50]
See also[edit]
- Student's t-test
- Bonferroni correction
- Counternull
- Fisher's method of combiningp-values
- Generalizedp-value
- Harmonic meanp-value
- Holm–Bonferroni method
- Multiple comparisons problem
- p-rep
- p-value fallacy
Notes[edit]
- ^Italicisation, capitalisation and hyphenation of the term vary. For example,AMA styleuses "Pvalue ",APA styleuses "pvalue ", and theAmerican Statistical Associationuses "p-value ". In all cases, the" p "stands for probability.[1]
- ^The statistical significance of a result does not imply that the result also has real-world relevance. For instance, a medication might have a statistically significant effect that is too small to be interesting.
- ^To be more specific, thep= 0.05 corresponds to about 1.96 standard deviations for a normal distribution (two-tailed test), and 2 standard deviations corresponds to about a 1 in 22 chance of being exceeded by chance, orp≈ 0.045; Fisher notes these approximations.
References[edit]
- ^"ASA House Style"(PDF).Amstat News.American Statistical Association.
- ^Aschwanden C(2015-11-24)."Not Even Scientists Can Easily Explain P-values".FiveThirtyEight.Archived fromthe originalon 25 September 2019.Retrieved11 October2019.
- ^abcdeWasserstein RL, Lazar NA (7 March 2016)."The ASA's Statement on p-Values: Context, Process, and Purpose".The American Statistician.70(2): 129–133.doi:10.1080/00031305.2016.1154108.
- ^Hubbard R, Lindsay RM (2008). "WhyPValues Are Not a Useful Measure of Evidence in Statistical Significance Testing ".Theory & Psychology.18(1): 69–88.doi:10.1177/0959354307086923.S2CID143487211.
- ^Munafò MR,Nosek BA, Bishop DV, Button KS, Chambers CD, du Sert NP, et al. (January 2017)."A manifesto for reproducible science".Nature Human Behaviour.1(1): 0021.doi:10.1038/s41562-016-0021.PMC7610724.PMID33954258.S2CID6326747.
- ^Wasserstein, Ronald L.; Lazar, Nicole A. (2016-04-02)."The ASA Statement on p -Values: Context, Process, and Purpose".The American Statistician.70(2): 129–133.doi:10.1080/00031305.2016.1154108.ISSN0003-1305.S2CID124084622.
- ^abBenjamini, Yoav; De Veaux, Richard D.; Efron, Bradley; Evans, Scott; Glickman, Mark; Graubard, Barry I.; He, Xuming; Meng, Xiao-Li; Reid, Nancy M.; Stigler, Stephen M.; Vardeman, Stephen B.; Wikle, Christopher K.; Wright, Tommy; Young, Linda J.; Kafadar, Karen (2021-10-02)."ASA President's Task Force Statement on Statistical Significance and Replicability".Chance.34(4). Informa UK Limited: 10–11.doi:10.1080/09332480.2021.2003631.ISSN0933-2480.
- ^Neyman, Jerzy(1976). "The Emergence of Mathematical Statistics: A Historical Sketch with Particular Reference to the United States". In Owen, D.B. (ed.).On the History of Statistics and Probability.Textbooks and Monographs. New York: Marcel Dekker Inc. p. 161.
- ^Benjamin, Daniel J.; Berger, James O.; Johannesson, Magnus; Nosek, Brian A.; Wagenmakers, E.-J.; Berk, Richard; Bollen, Kenneth A.; Brembs, Björn; Brown, Lawrence; Camerer, Colin; Cesarini, David; Chambers, Christopher D.; Clyde, Merlise; Cook, Thomas D.; De Boeck, Paul; Dienes, Zoltan; Dreber, Anna; Easwaran, Kenny; Efferson, Charles; Fehr, Ernst; Fidler, Fiona; Field, Andy P.; Forster, Malcolm; George, Edward I.; Gonzalez, Richard; Goodman, Steven; Green, Edwin; Green, Donald P.; Greenwald, Anthony G.; Hadfield, Jarrod D.; Hedges, Larry V.; Held, Leonhard; Hua Ho, Teck; Hoijtink, Herbert; Hruschka, Daniel J.; Imai, Kosuke; Imbens, Guido; Ioannidis, John P. A.; Jeon, Minjeong; Jones, James Holland; Kirchler, Michael; Laibson, David; List, John; Little, Roderick; Lupia, Arthur; Machery, Edouard; Maxwell, Scott E.; McCarthy, Michael; Moore, Don A.; Morgan, Stephen L.; Munafó, Marcus; Nakagawa, Shinichi; Nyhan, Brendan; Parker, Timothy H.; Pericchi, Luis; Perugini, Marco; Rouder, Jeff; Rousseau, Judith; Savalei, Victoria; Schönbrodt, Felix D.; Sellke, Thomas; Sinclair, Betsy; Tingley, Dustin; Van Zandt, Trisha; Vazire, Simine; Watts, Duncan J.; Winship, Christopher; Wolpert, Robert L.; Xie, Yu; Young, Cristobal; Zinman, Jonathan; Johnson, Valen E. (1 September 2017)."Redefine statistical significance".Nature Human Behaviour.2(1): 6–10.doi:10.1038/s41562-017-0189-z.eISSN2397-3374.hdl:10281/184094.PMID30980045.S2CID256726352.
- ^abHead ML, Holman L, Lanfear R, Kahn AT, Jennions MD (March 2015)."The extent and consequences of p-hacking in science".PLOS Biology.13(3): e1002106.doi:10.1371/journal.pbio.1002106.PMC4359000.PMID25768323.
- ^Simonsohn U, Nelson LD, Simmons JP (November 2014). "p-Curve and Effect Size: Correcting for Publication Bias Using Only Significant Results ".Perspectives on Psychological Science.9(6): 666–681.doi:10.1177/1745691614553988.PMID26186117.S2CID39975518.
- ^Bhattacharya B, Habtzghi D (2002). "Median of the p value under the alternative hypothesis".The American Statistician.56(3): 202–6.doi:10.1198/000313002146.S2CID33812107.
- ^Hung HM, O'Neill RT, Bauer P, Köhne K (March 1997)."The behavior of the P-value when the alternative hypothesis is true".Biometrics(Submitted manuscript).53(1): 11–22.doi:10.2307/2533093.JSTOR2533093.PMID9147587.
- ^Nuzzo R(February 2014)."Scientific method: statistical errors".Nature.506(7487): 150–152.Bibcode:2014Natur.506..150N.doi:10.1038/506150a.PMID24522584.
- ^Colquhoun D (November 2014)."An investigation of the false discovery rate and the misinterpretation of p-values".Royal Society Open Science.1(3): 140216.arXiv:1407.5296.Bibcode:2014RSOS....140216C.doi:10.1098/rsos.140216.PMC4448847.PMID26064558.
- ^Lee DK (December 2016)."Alternatives to P value: confidence interval and effect size".Korean Journal of Anesthesiology.69(6): 555–562.doi:10.4097/kjae.2016.69.6.555.PMC5133225.PMID27924194.
- ^Ranstam J (August 2012)."Why the P-value culture is bad and confidence intervals a better alternative".Osteoarthritis and Cartilage.20(8): 805–808.doi:10.1016/j.joca.2012.04.001.PMID22503814.
- ^Perneger TV (May 2001)."Sifting the evidence. Likelihood ratios are alternatives to P values".BMJ.322(7295): 1184–1185.doi:10.1136/bmj.322.7295.1184.PMC1120301.PMID11379590.
- ^Royall R (2004). "The Likelihood Paradigm for Statistical Evidence".The Nature of Scientific Evidence.pp. 119–152.doi:10.7208/chicago/9780226789583.003.0005.ISBN9780226789576.
- ^Schimmack U (30 April 2015)."Replacing p-values with Bayes-Factors: A Miracle Cure for the Replicability Crisis in Psychological Science".Replicability-Index.Retrieved7 March2017.
- ^Marden JI (December 2000). "Hypothesis Testing: From p Values to Bayes Factors".Journal of the American Statistical Association.95(452): 1316–1320.doi:10.2307/2669779.JSTOR2669779.
- ^Stern HS (16 February 2016)."A Test by Any Other Name: P Values, Bayes Factors, and Statistical Inference".Multivariate Behavioral Research.51(1): 23–29.doi:10.1080/00273171.2015.1099032.PMC4809350.PMID26881954.
- ^Murtaugh PA (March 2014)."In defense of P values".Ecology.95(3): 611–617.Bibcode:2014Ecol...95..611M.doi:10.1890/13-0590.1.PMID24804441.
- ^Aschwanden C(7 March 2016)."Statisticians Found One Thing They Can Agree On: It's Time To Stop Misusing P-Values".FiveThirtyEight.
- ^Amrhein V,Korner-Nievergelt F, Roth T (2017)."The earth is flat (p> 0.05): significance thresholds and the crisis of unreplicable research ".PeerJ.5:e3544.doi:10.7717/peerj.3544.PMC5502092.PMID28698825.
- ^Amrhein V,Greenland S (January 2018). "Remove, rather than redefine, statistical significance".Nature Human Behaviour.2(1): 4.doi:10.1038/s41562-017-0224-0.PMID30980046.S2CID46814177.
- ^Colquhoun D (December 2017)."The reproducibility of research and the misinterpretation ofp-values ".Royal Society Open Science.4(12): 171085.doi:10.1098/rsos.171085.PMC5750014.PMID29308247.
- ^Brian E,Jaisson M(2007). "Physico-Theology and Mathematics (1710–1794)".The Descent of Human Sex Ratio at Birth.Springer Science & Business Media. pp.1–25.ISBN978-1-4020-6036-6.
- ^Arbuthnot J (1710)."An argument for Divine Providence, taken from the constant regularity observed in the births of both sexes"(PDF).Philosophical Transactions of the Royal Society of London.27(325–336): 186–190.doi:10.1098/rstl.1710.0011.S2CID186209819.
- ^abConover WJ (1999). "Chapter 3.4: The Sign Test".Practical Nonparametric Statistics(Third ed.). Wiley. pp. 157–176.ISBN978-0-471-16068-7.
- ^Sprent P (1989).Applied Nonparametric Statistical Methods(Second ed.). Chapman & Hall.ISBN978-0-412-44980-2.
- ^Stigler SM (1986).The History of Statistics: The Measurement of Uncertainty Before 1900.Harvard University Press. pp.225–226.ISBN978-0-67440341-3.
- ^Bellhouse P (2001). "John Arbuthnot". InHeyde CC,Seneta E(eds.).Statisticians of the Centuries.Springer. pp. 39–42.ISBN978-0-387-95329-8.
- ^Hald A (1998). "Chapter 4. Chance or Design: Tests of Significance".A History of Mathematical Statistics from 1750 to 1930.Wiley. p. 65.
- ^Stigler SM (1986).The History of Statistics: The Measurement of Uncertainty Before 1900.Harvard University Press. p.134.ISBN978-0-67440341-3.
- ^abPearson K(1900)."On the criterion that a given system of deviations from the probable in the case of a correlated system of variables is such that it can be reasonably supposed to have arisen from random sampling"(PDF).Philosophical Magazine.Series 5.50(302): 157–175.doi:10.1080/14786440009463897.
- ^Biau, David Jean; Jolles, Brigitte M.; Porcher, Raphaël (2010)."P Value and the Theory of Hypothesis Testing: An Explanation for New Researchers".Clinical Orthopaedics and Related Research.468(3): 885–892.doi:10.1007/s11999-009-1164-4.ISSN0009-921X.PMC2816758.PMID19921345.
- ^Brereton, Richard G. (2021)."P values and multivariate distributions: Non-orthogonal terms in regression models".Chemometrics and Intelligent Laboratory Systems.210:104264.doi:10.1016/j.chemolab.2021.104264.
- ^Hubbard R, Bayarri MJ (2003), "Confusion Over Measures of Evidence (p′s) Versus Errors (α′s) in Classical Statistical Testing ",The American Statistician,57(3): 171–178 [p. 171],doi:10.1198/0003130031856,S2CID55671953
- ^Fisher 1925,p. 47, ChapterIII. Distributions.
- ^abDallal 2012,Note 31:Why P=0.05?.
- ^Fisher 1925,pp. 78–79, 98, ChapterIV. Tests of Goodness of Fit, Independence and Homogeneity; with Table ofχ2,Table III. Table ofχ2.
- ^Fisher 1971,II. The Principles of Experimentation, Illustrated by a Psycho-physical Experiment.
- ^abFisher 1971,Section 7. The Test of Significance.
- ^Fisher 1971,Section 12.1 Scientific Inference and Acceptance Procedures.
- ^"Definition of E-value".National Institutes of Health.
- ^Storey JD (2003)."The positive false discovery rate: a Bayesian interpretation and the q-value".The Annals of Statistics.31(6): 2013–2035.doi:10.1214/aos/1074290335.
- ^Storey JD, Tibshirani R (August 2003)."Statistical significance for genomewide studies".Proceedings of the National Academy of Sciences of the United States of America.100(16): 9440–9445.Bibcode:2003PNAS..100.9440S.doi:10.1073/pnas.1530509100.PMC170937.PMID12883005.
- ^Makowski D, Ben-Shachar MS, Chen SH, Lüdecke D (10 December 2019)."Indices of Effect Existence and Significance in the Bayesian Framework".Frontiers in Psychology.10:2767.doi:10.3389/fpsyg.2019.02767.PMC6914840.PMID31920819.
- ^An Introduction to Second-Generation p-Values Jeffrey D. Blume, Robert A. Greevy, Valerie F. Welty, Jeffrey R. Smith &William D. Duponthttps:// tandfonline /doi/full/10.1080/00031305.2018.1537893
Further reading[edit]
- Denworth L (October 2019). "A Significant Problem: Standard scientific methods are under fire. Will anything change?".Scientific American.321(4): 62–67 (63).
The use ofpvaluesfor nearly a century [since 1925] to determinestatistical significanceofexperimentalresults has contributed to an illusion ofcertaintyand [to]reproducibility crisesin manyscientific fields.There is growing determination to reform statistical analysis... Some [researchers] suggest changing statistical methods, whereas others would do away with a threshold for defining "significant" results.
- Elderton WP(1902)."Tables for Testing the Goodness of Fit of Theory to Observation".Biometrika.1(2): 155–163.doi:10.1093/biomet/1.2.155.
- Fisher RA(1925).Statistical Methods for Research Workers.Edinburgh, Scotland: Oliver & Boyd.ISBN978-0-05-002170-5.
- Fisher RA(1971) [1935].The Design of Experiments(9th ed.). Macmillan.ISBN978-0-02-844690-5.
- Fisher RA, Yates F (1938).Statistical tables for biological, agricultural and medical research.London, England.
{{cite book}}
:CS1 maint: location missing publisher (link) - Stigler SM(1986).The history of statistics: the measurement of uncertainty before 1900.Cambridge, Mass: Belknap Press of Harvard University Press.ISBN978-0-674-40340-6.
- Hubbard R,Armstrong JS(2006)."Why We Don't Really Know What Statistical Significance Means: Implications for Educators"(PDF).Journal of Marketing Education.28(2): 114–120.doi:10.1177/0273475306288399.hdl:2092/413.S2CID34729227.Archived fromthe original(PDF)on May 18, 2006.
- Hubbard R, Lindsay RM (2008)."WhyPValues Are Not a Useful Measure of Evidence in Statistical Significance Testing "(PDF).Theory & Psychology.18(1): 69–88.doi:10.1177/0959354307086923.S2CID143487211.Archived fromthe original(PDF)on 2016-10-21.Retrieved2015-08-28.
- Stigler S(December 2008)."Fisher and the 5% level".Chance.21(4): 12.doi:10.1007/s00144-008-0033-3.
- Dallal GE (2012).The Little Handbook of Statistical Practice.
- Biau DJ, Jolles BM, Porcher R (March 2010)."P value and the theory of hypothesis testing: an explanation for new researchers".Clinical Orthopaedics and Related Research.468(3): 885–892.doi:10.1007/s11999-009-1164-4.PMC2816758.PMID19921345.
- Reinhart A (2015).Statistics Done Wrong: The Woefully Complete Guide.No Starch Press.p. 176.ISBN978-1593276201.
- Benjamini, Yoav;De Veaux, Richard D.;Efron, Bradley;Evans, Scott; Glickman, Mark; Graubard, Barry I.; He, Xuming;Meng, Xiao-Li;Reid, Nancy;Stigler, Stephen M.;Vardeman, Stephen B.; Wikle, Christopher K.; Wright, Tommy; Young, Linda J.;Kafadar, Karen(2021)."The ASA President's Task Force Statement on Statistical Significance and Replicability".Annals of Applied Statistics.15(3): 1084–1085.doi:10.1214/21-AOAS1501.
- Benjamin, Daniel J.; Berger, James O.; Johannesson, Magnus; Nosek, Brian A.; Wagenmakers, E.-J.; Berk, Richard; Bollen, Kenneth A.; Brembs, Björn; Brown, Lawrence; Camerer, Colin; Cesarini, David; Chambers, Christopher D.; Clyde, Merlise; Cook, Thomas D.; De Boeck, Paul; Dienes, Zoltan; Dreber, Anna; Easwaran, Kenny; Efferson, Charles; Fehr, Ernst; Fidler, Fiona; Field, Andy P.; Forster, Malcolm; George, Edward I.; Gonzalez, Richard; Goodman, Steven; Green, Edwin; Green, Donald P.; Greenwald, Anthony G.; Hadfield, Jarrod D.; Hedges, Larry V.; Held, Leonhard; Hua Ho, Teck; Hoijtink, Herbert; Hruschka, Daniel J.; Imai, Kosuke; Imbens, Guido; Ioannidis, John P. A.; Jeon, Minjeong; Jones, James Holland; Kirchler, Michael; Laibson, David; List, John; Little, Roderick; Lupia, Arthur; Machery, Edouard; Maxwell, Scott E.; McCarthy, Michael; Moore, Don A.; Morgan, Stephen L.; Munafó, Marcus; Nakagawa, Shinichi; Nyhan, Brendan; Parker, Timothy H.; Pericchi, Luis; Perugini, Marco; Rouder, Jeff; Rousseau, Judith; Savalei, Victoria; Schönbrodt, Felix D.; Sellke, Thomas; Sinclair, Betsy; Tingley, Dustin; Van Zandt, Trisha; Vazire, Simine; Watts, Duncan J.; Winship, Christopher; Wolpert, Robert L.; Xie, Yu; Young, Cristobal; Zinman, Jonathan; Johnson, Valen E. (1 September 2017)."Redefine statistical significance".Nature Human Behaviour.2(1): 6–10.doi:10.1038/s41562-017-0189-z.eISSN2397-3374.hdl:10281/184094.PMID30980045.S2CID256726352.
External links[edit]

- Free onlinep-values calculatorsfor various specific tests (chi-square, Fisher's F-test, etc.).
- Understandingp-values,including a Java applet that illustrates how the numerical values ofp-values can give quite misleading impressions about the truth or falsity of the hypothesis under test.
- StatQuest: P Values, clearly explainedonYouTube
- StatQuest: P-value pitfalls and power calculationsonYouTube
- Science Isn’t Broken - Article on howp-values can be manipulated and an interactive tool to visualize it.